EFFECT: An End-to-End Framework for Evaluating Strategies for Parallel AI Anomaly Detection
INNS DLIA@IJCNN(2023)
摘要
Neural networks achieve high accuracy in tasks like image recognition or segmentation. However, their application in safety-critical domains is limited due to their black-box nature and vulnerability to specific types of attacks. To mitigate this, methods detecting out-of-distribution or adversarial attacks in parallel to the network inference were introduced. These methods are hard to compare because they were developed for different use cases, datasets, and networks. To fill this gap, we introduce EFFECT, an end-to-end framework to evaluate and compare new methods for anomaly detection, without the need for retraining and by using traces of intermediate inference results. The presented workflow works with every preexisting neural network architecture and evaluates the considered anomaly detection methods in terms of accuracy and computational complexity. We demonstrate EFFECT's capabilities, by creating new detectors for ShuffleNet and MobileNetV2 for anomaly detection as well as fault origin detection. EFFECT allows us to design an anomaly detector, based on the Mahalanobis distance as well as CNN based detectors. For both use cases, we achieve accuracies of over 85 %, classifying inferences as normal or abnormal, and thus beating existing methods.
更多查看译文
关键词
AI security,inference monitoring,layer tracing,evaluation framework,anomaly detection
AI 理解论文
溯源树
样例
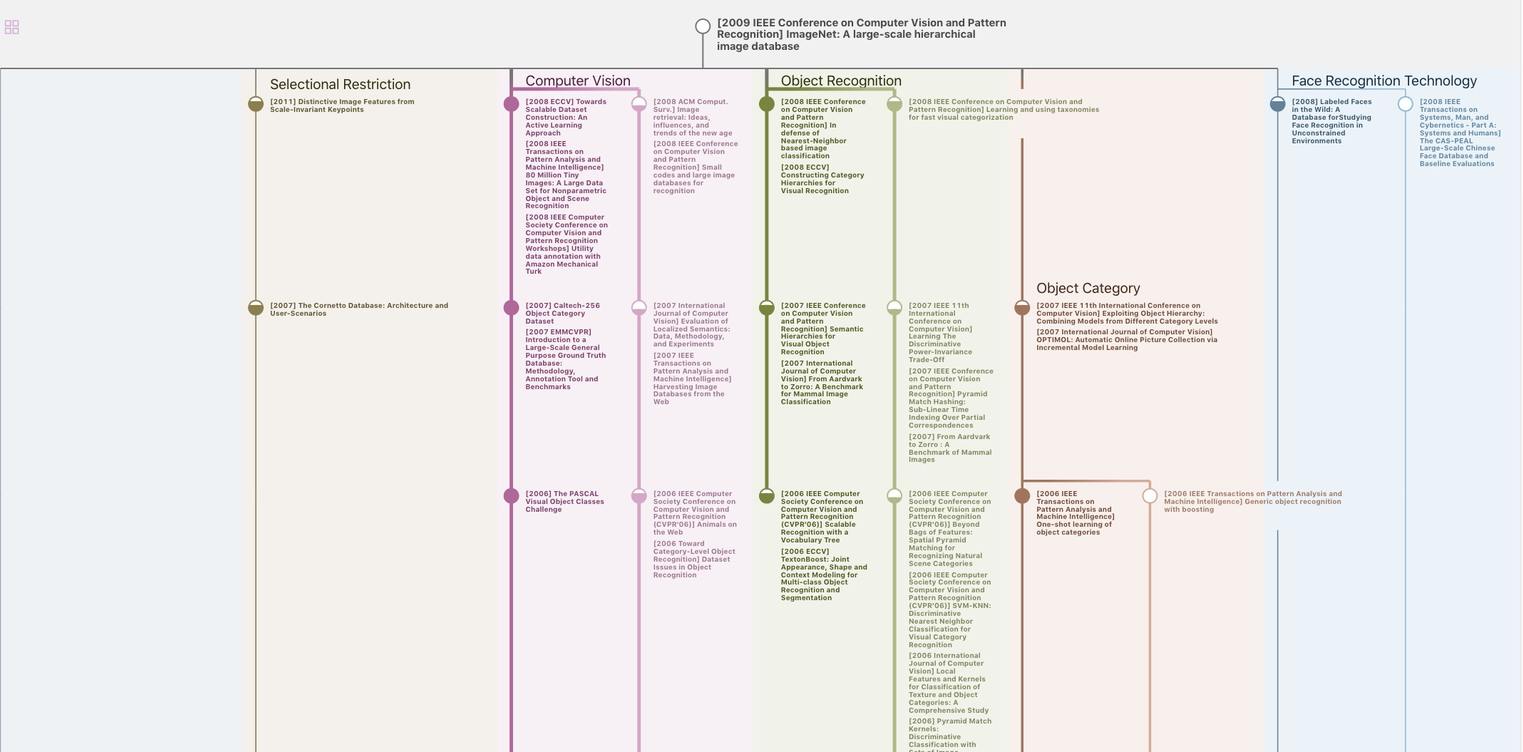
生成溯源树,研究论文发展脉络
Chat Paper
正在生成论文摘要