Investigating the effects of incremental training on neural ranking models
PROCEEDINGS OF THE 17TH ACM CONFERENCE ON RECOMMENDER SYSTEMS, RECSYS 2023(2023)
摘要
Recommender systems are an essential component of online platforms providing users with personalized experiences. Some recommendation scenarios such as social networks and news are extremely dynamic in nature with user interests changing over time and new items being continuously added due to breaking news and trending events. Incremental training is a popular technique to keep recommender models up-to-date in such dynamic platforms. In this paper, we provide an empirical analysis of a large industry dataset from the Sharechat app MOJ, a social media platform featuring short videos, to answer relevant questions like - How often should I retrain the models? - do different model architectures, features and dataset sizes benefit differently from incremental training? - Does incremental training equally benefit all users and items?
更多查看译文
关键词
Recommender Systems,Incremental Training,Feature Engineering,Streaming data,Deep Learning
AI 理解论文
溯源树
样例
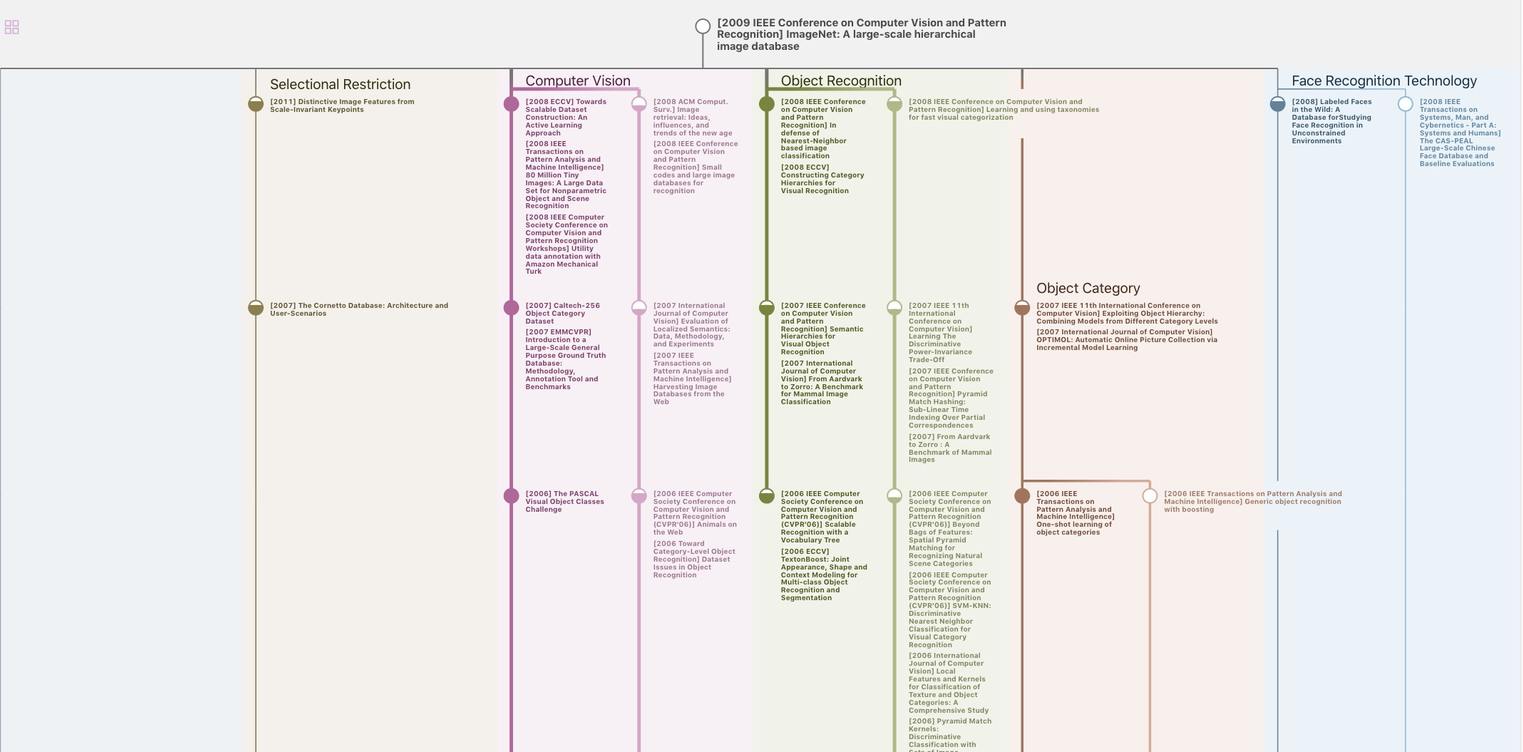
生成溯源树,研究论文发展脉络
Chat Paper
正在生成论文摘要