Distribution-based Learnable Filters with Side Information for Sequential Recommendation
PROCEEDINGS OF THE 17TH ACM CONFERENCE ON RECOMMENDER SYSTEMS, RECSYS 2023(2023)
摘要
Sequential Recommendation aims to predict the next item by mining out the dynamic preference from user previous interactions. However, most methods represent each item as a single fixed vector, which is incapable of capturing the uncertainty of item-item transitions that result from time-dependent and multifarious interests of users. Besides, they struggle to effectively exploit side information that helps to better express user preferences. Finally, the noise in user's access sequence, which is due to accidental clicks, can interfere with the next item prediction and lead to lower recommendation performance. To deal with these issues, we propose DLFS-Rec, a simple and novel model that combines Distribution-based Learnable Filters with Side information for sequential Recommendation. Specifically, items and their side information are represented by stochastic Gaussian distribution, which is described by mean and covariance embeddings, and then the corresponding embeddings are fused to generate a final representation for each item. To attenuate noise, stacked learnable filter layers are applied to smooth the fused embeddings. Extensive experiments on four public real-world datasets demonstrate the superiority of the proposed model over state-of-the-art baselines, especially on cold start users and items. Codes are available at https://github.com/zxiang30/DLFS-Rec.
更多查看译文
关键词
Sequential Recommendation,Learnable Filter,Gaussian Distribution,Side Information
AI 理解论文
溯源树
样例
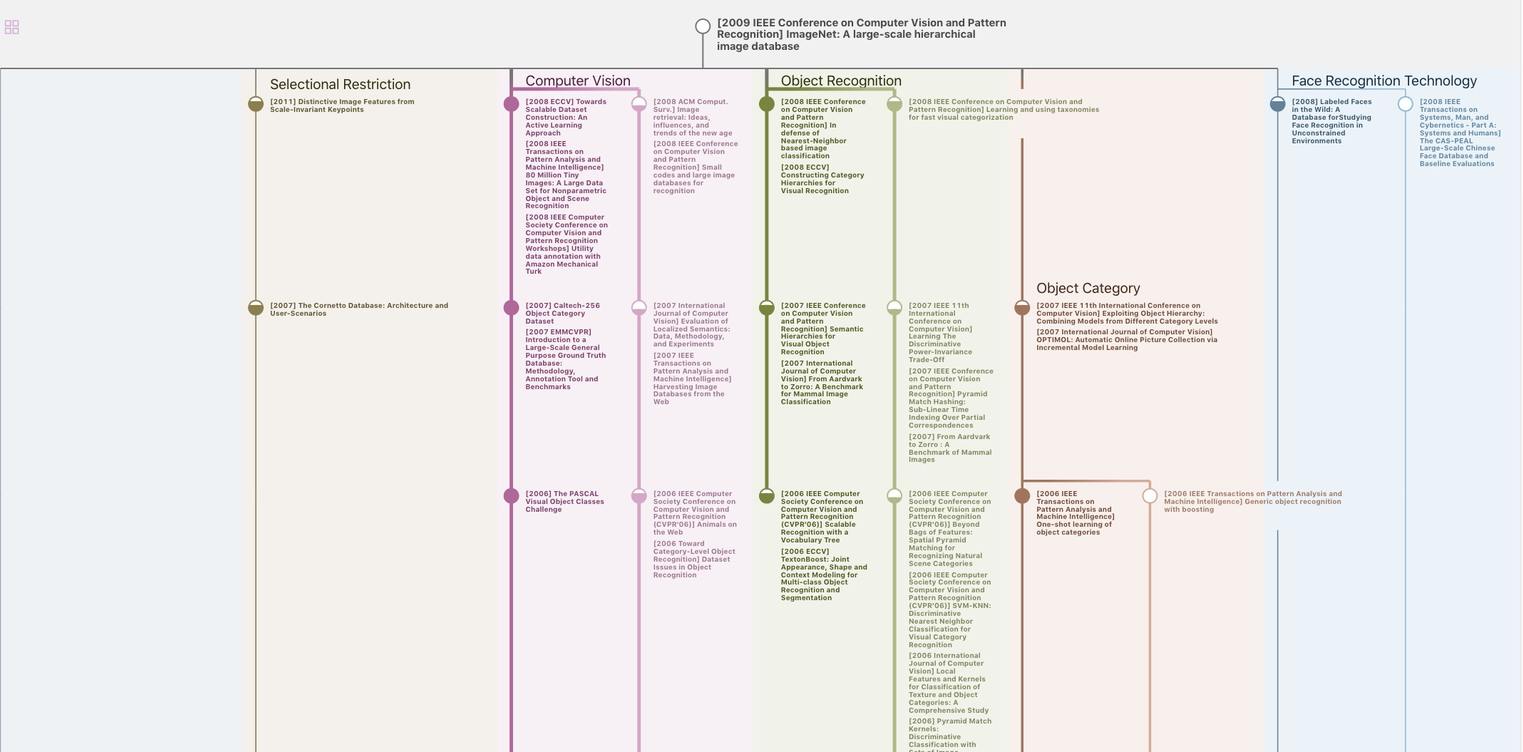
生成溯源树,研究论文发展脉络
Chat Paper
正在生成论文摘要