Galliot: Path Merging Based Betweenness Centrality Algorithm on GPU.
INFOCOM(2023)
摘要
Betweenness centrality (BC) is widely used to measure a vertex’s significance by using the frequency of a vertex appearing in the shortest path between other vertices. However, most recent algorithms in BC computation suffer from the problem of high auxiliary memory consumption. To reduce BC computing’s memory consumption, we propose a path-mergingbased algorithm called Galliot to calculate the BC values on GPU, which aims to minimize the on-board memory consumption and enable the BC computation of large-scale graphs. The proposed algorithm requires $\mathcal{O}$(n) space and runs in $\mathcal{O}$(mn) time on unweighted graphs. We present the theoretical principle for the proposed path merging method. Moreover, we propose a locality-oriented policy to maintain and update the worklist to improve GPU data locality. In addition, we conducted extensive experiments on NVIDIA GPUs to show the performance of Galliot. The results show that Galliot can process the larger graphs, which have 11.32× more vertices and 5.67× more edges than the graphs that recent works. Moreover, Galliot can achieve up to 38.77× speedup over the existing methods.
更多查看译文
关键词
Betweenness Centrality,Path Merging Method,Edge Sharing,GPGPU,Parallelism
AI 理解论文
溯源树
样例
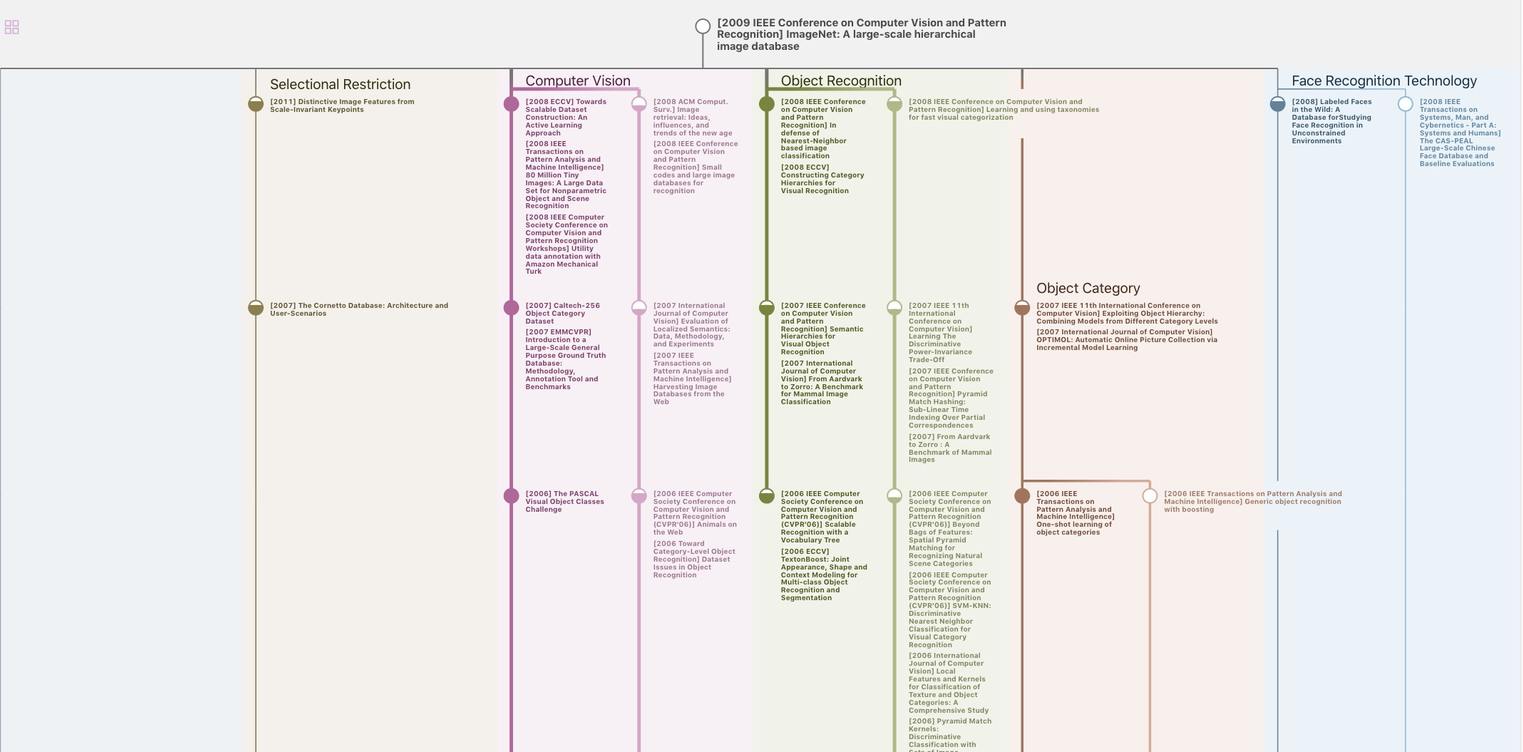
生成溯源树,研究论文发展脉络
Chat Paper
正在生成论文摘要