A Modified MOEA/D Based on Guided Search Directions for Large-scale Multiobjective Optimization.
CEC(2023)
摘要
Approximating the Pareto fronts of large-scale multiobjective optimization problems (LSMOPs) is a very changeling task due to their huge search spaces caused by the large number of decision variables. It is a commonly-used idea that large scale optimization problems are often transformed into small scale optimization problems that can be solved by existing optimization methods. In this paper, we investigate an improved version of MOEA/D with dimensionality reduction for large-scale multiobjective optimization, denoted by LS-MOEA/D-GSD. The major ideas in our proposed method focus on two aspects. On the one hand, the original search space of LSMOPs is transformed into a small-scale MOP on weight variables of several guided search directions via the genetic operators in differential evolution. On the other hand, the computational resources are allocated to both the original search space and reduced search space. Some experiments are conducted to test the performance of our proposed algorithm on the well-known large scale multiobjective test suites, i.e., LSMOP1-9 with up to 1000 variables. Our experimental results show that our algorithm outperforms several state-of-the-art multiobjective evolutionary algorithms for large scale multiobjective optimization.
更多查看译文
关键词
Large-scale multiobjective optimization,Dimensionality Reduction,Descent Search Directions
AI 理解论文
溯源树
样例
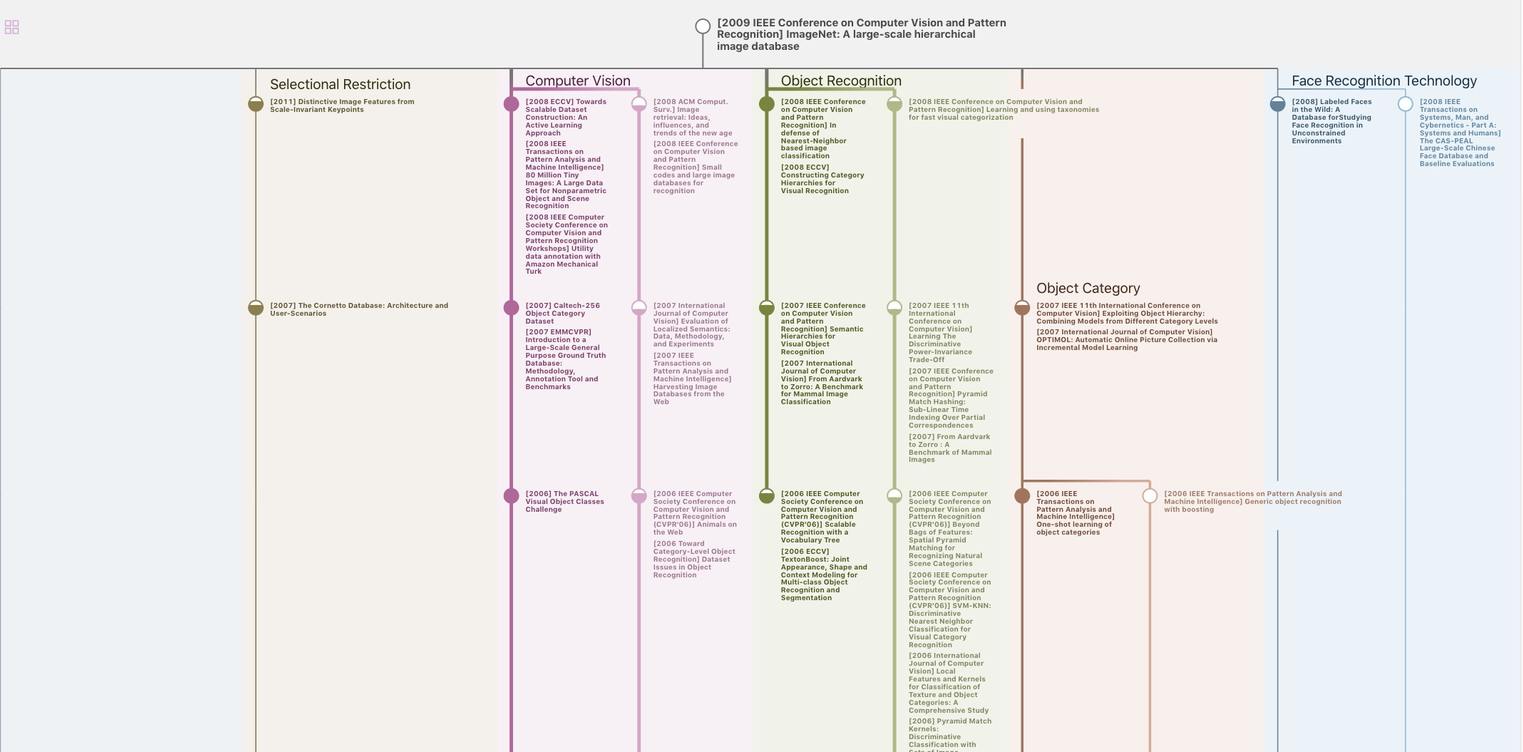
生成溯源树,研究论文发展脉络
Chat Paper
正在生成论文摘要