Insights from the HARP Framework: Using an AI-Driven Approach for Efficient Resource Allocation in HPC Scientific Workflows.
PEARC(2023)
摘要
High-performance computing (HPC) is essential for scientific research, and efficient utilization of such high-demand resources requires end users to understand their scientific workflows or tools and their synergy with the execution environment. There needs to be more workflow-specific history for machine learning (ML) models to estimate resource requirements tailored to a specific workflow or set of applications. In this work, we present the potential problems encountered while manually finetuning a workflow for optimal resource utilization without overprovisioning or under-allocating the resources. We highlight the need for an AI-driven framework to estimate the resource requirements of applications within the workflow and recommend optimal resource allocation configurations. We introduce our generalizable AI-driven application, the HPC Application Resource (runtime) Predictor (HARP) Framework. HARP generates execution history against application parameters and available hardware, builds several regression models, and selects the best model to recommend cost-based or time-based configurations for optimal resource allocation. Our work demonstrates the effectiveness of HARP for estimating resource requirements against Ohio Supercomputer Center (OSC) for training a fully-connected neural net for image classification.
更多查看译文
关键词
efficient resource allocation,resource allocation,harp framework,ai-driven
AI 理解论文
溯源树
样例
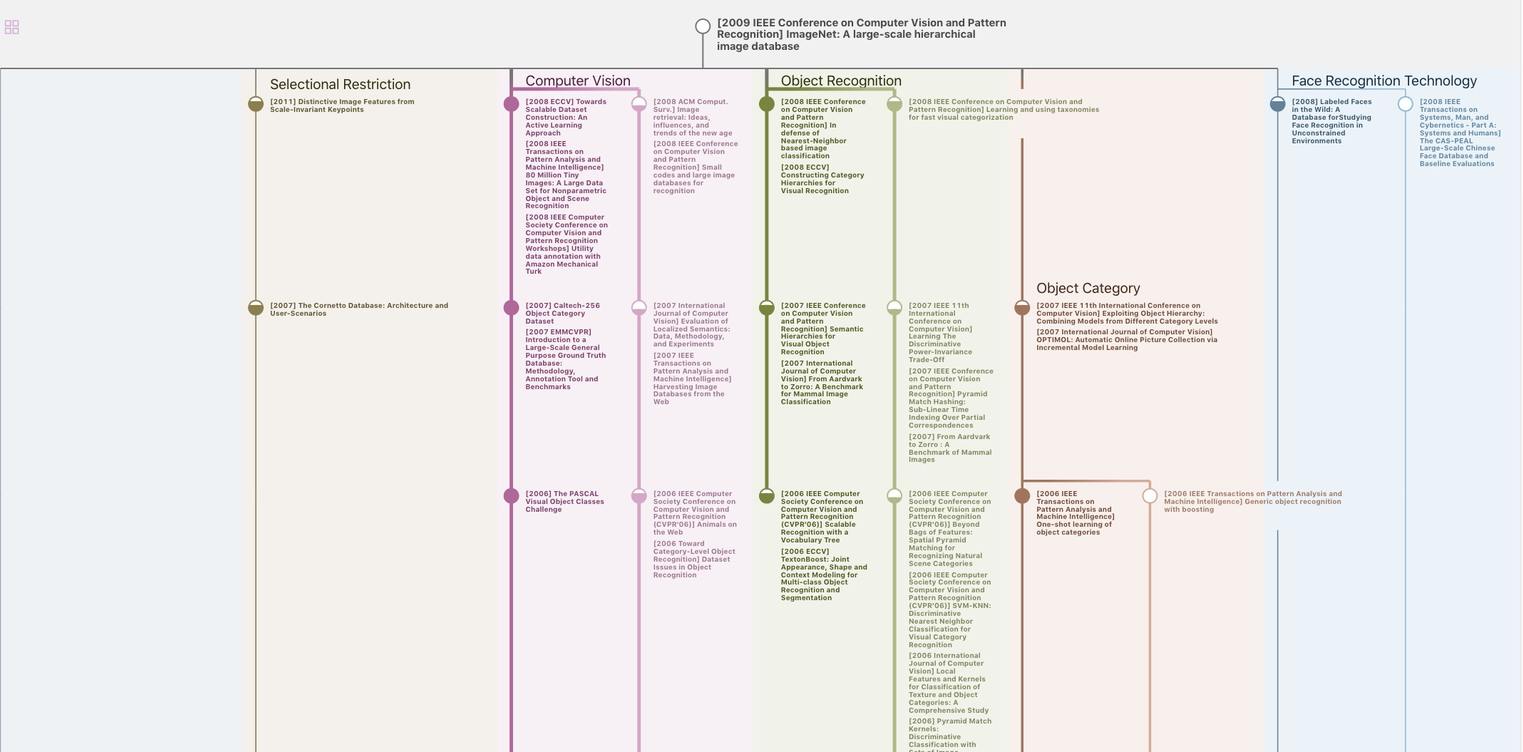
生成溯源树,研究论文发展脉络
Chat Paper
正在生成论文摘要