Testing Quality of Training in QoE-Aware SFC Orchestration Based on DRL Approach
TESTING SOFTWARE AND SYSTEMS, ICTSS 2023(2023)
摘要
In this paper, we propose a Deep Reinforcement Learning (DRL) approach to optimize a learning policy for Service Function Chaining (SFC) orchestration based on maximizing Quality of Experience (QoE) while meeting Quality of Service (QoS) requirements in Software Defined Networking (SDN)/Network Functions Virtualization (NFV) environments. We adopt an incremental orchestration strategy suitable to online setting and enabling to investigate SFC orchestration by processing each incoming SFC request as a multi-step DRL problem. DRL implementation is achieved using Deep Q-Networks (DQNs) variant referred to as Double DQN. We particularly focus on evaluating performance and robustness of the DRL agent during training phase by investigating and testing the quality of training. In this regard, we define a testing metric monitoring the performance of the DRL agent and quantified by a QoE threshold score to reach on average during the last 100 runs of the training phase. We show through numerical results how DRL agent behaves during training phase and how it attempts to reach for different network scales a predefined average QoE threshold score. We highlight also network scalability effect on achieving a suitable performance-convergence trade-off.
更多查看译文
关键词
Learning Quality,DRL,SDN/NFV,SFC Orchestration
AI 理解论文
溯源树
样例
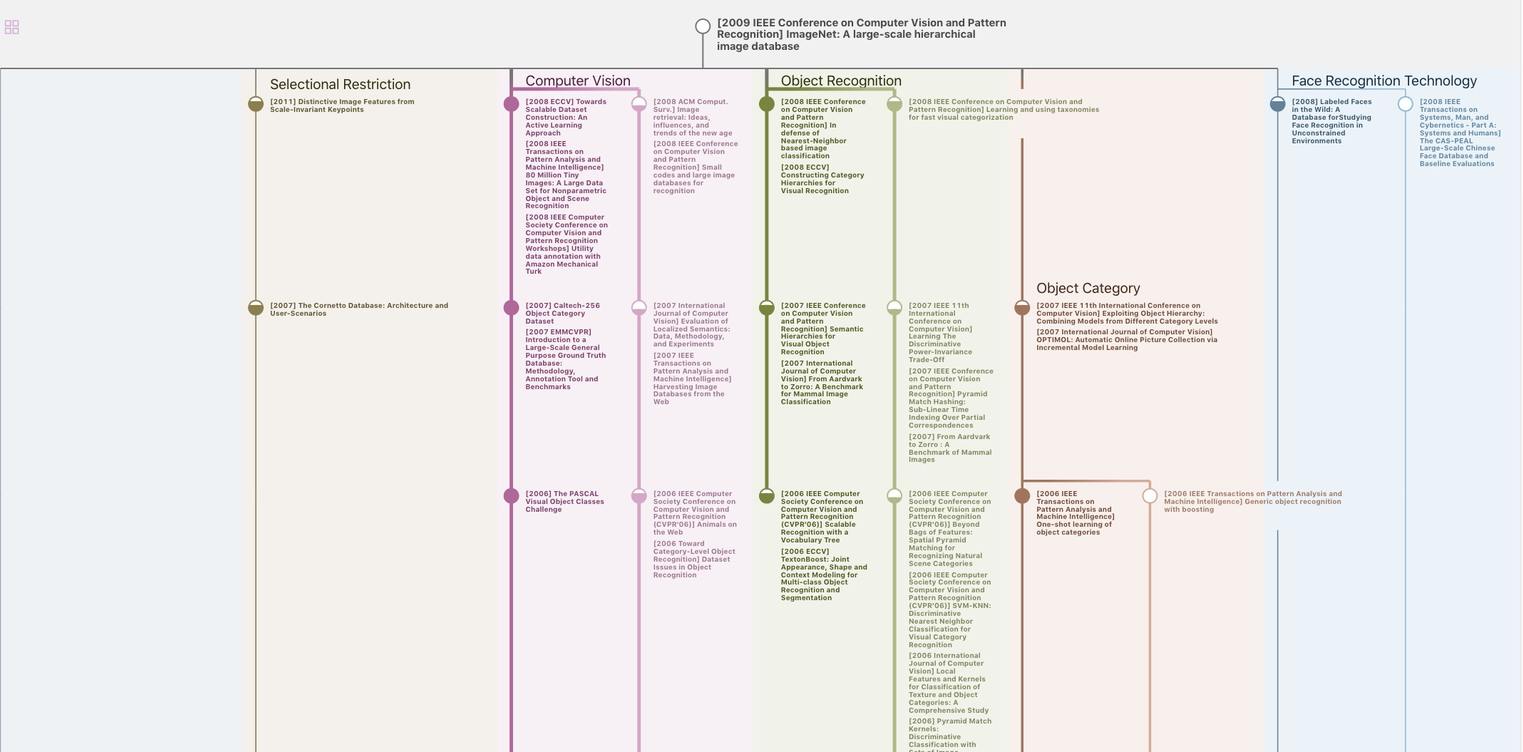
生成溯源树,研究论文发展脉络
Chat Paper
正在生成论文摘要