Regularization for Uplift Regression
MACHINE LEARNING AND KNOWLEDGE DISCOVERY IN DATABASES: RESEARCH TRACK, ECML PKDD 2023, PT I(2023)
摘要
We address the problem of regularization of linear regression models in uplift modeling and heterogeneous treatment effect estimation. We consider interaction models which are commonly used by statisticians in medicine and social sciences to estimate the causal effect of a treatment, and introduce a new type of such a model. We demonstrate the equivalence of all interaction models when no regularization is present, and that this is no longer the case when the model is regularized. Interaction terms introduce implicit correlations between treatment and control coefficients into the regularizer, a fact which has not been previously noted. The correlations depend on the type of interaction model, and by interpreting the regularizer as a prior distribution we were able to pinpoint cases when a given regularized interaction model is most appropriate. An interesting property of the proposed new interaction type is that it allows for smooth interpolation between two types of uplift regression models: the double model and the transformed target model. Our results are valid for both ridge (L2) and Lasso (L1) regularization. Experiments on synthetic data fully confirm our analyses. We also compare the usefulness of various regularization schemes on real data.
更多查看译文
关键词
uplift modeling,heterogeneous treatment effect,regularization,linear models,Lasso
AI 理解论文
溯源树
样例
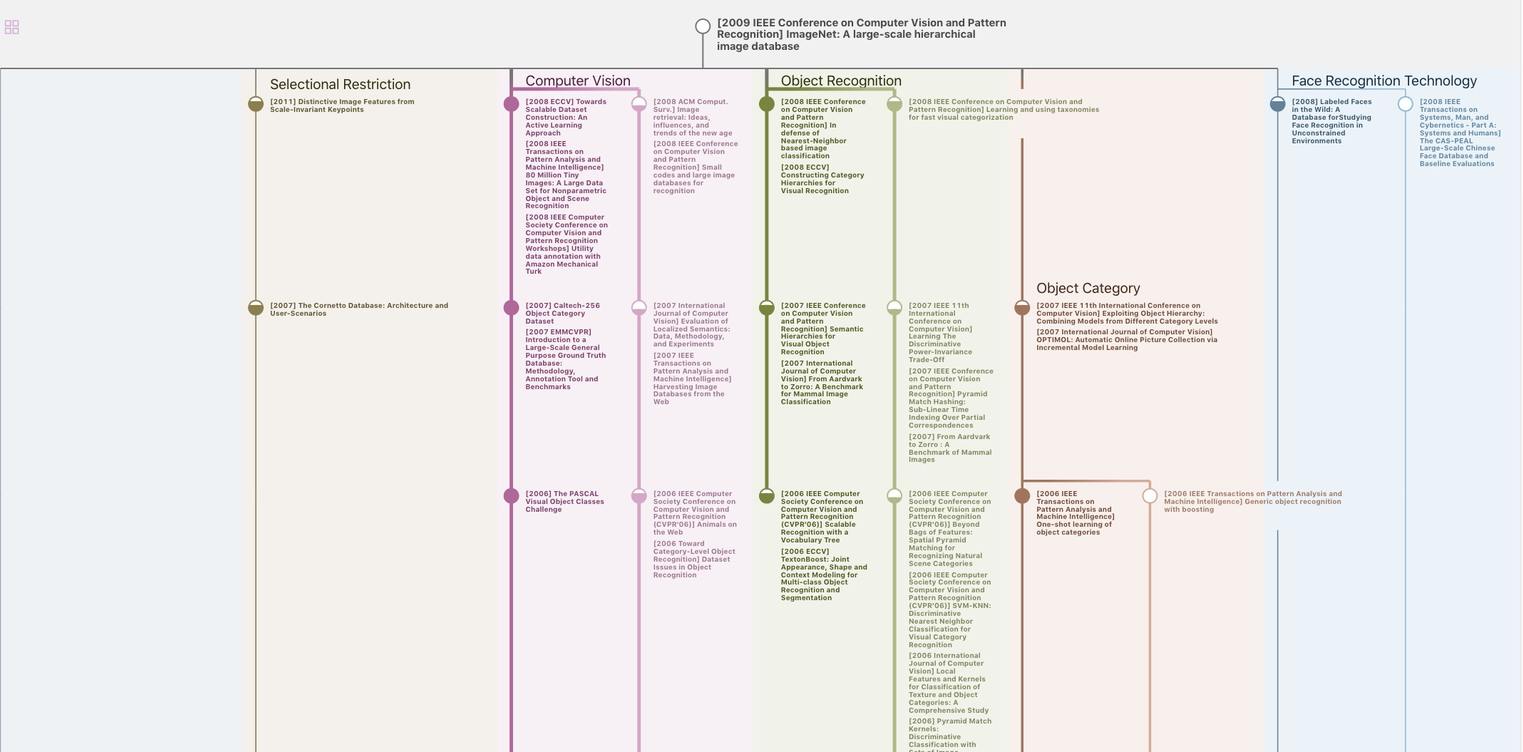
生成溯源树,研究论文发展脉络
Chat Paper
正在生成论文摘要