CAENet: Efficient Multi-task Learning for Joint Semantic Segmentation and Depth Estimation
MACHINE LEARNING AND KNOWLEDGE DISCOVERY IN DATABASES: RESEARCH TRACK, ECML PKDD 2023, PT V(2023)
摘要
In this paper, we propose an efficient multi-task method, named Context-aware Attentive Enrichment Network (CAENet), to deal with the problem of real-time joint semantic segmentation and depth estimation. Building upon a light-weight encoder backbone, an efficient decoder is devised to fully leverage available information from multi-scale encoder features. In particular, a new Inception Residual Pooling (IRP) module is designed to efficiently extract contextual information from the high-level features with diverse receptive fields to improve semantic understanding ability. Then the context-aware features are enriched adaptively with spatial details from low-level features via a Light-weight Attentive Fusion (LAF) module using pseudo stereoscopic attention mechanism. These two modules are progressively used in a recursive manner to generate high-resolution shared features, which are further processed by task-specific heads to produce final outputs. Such network design effectively captures beneficial information for both semantic segmentation and depth estimation tasks while largely reducing the computational budget. Extensive experiments across multi-task benchmarks validate that CAENet achieves state-of-the-art performance with comparable inference speed against other real-time competing methods. Code is available at https://github.com/wlx-zju/CAENet.
更多查看译文
关键词
Multi-task learning,Semantic segmentation,Depth estimation,Light-weight network
AI 理解论文
溯源树
样例
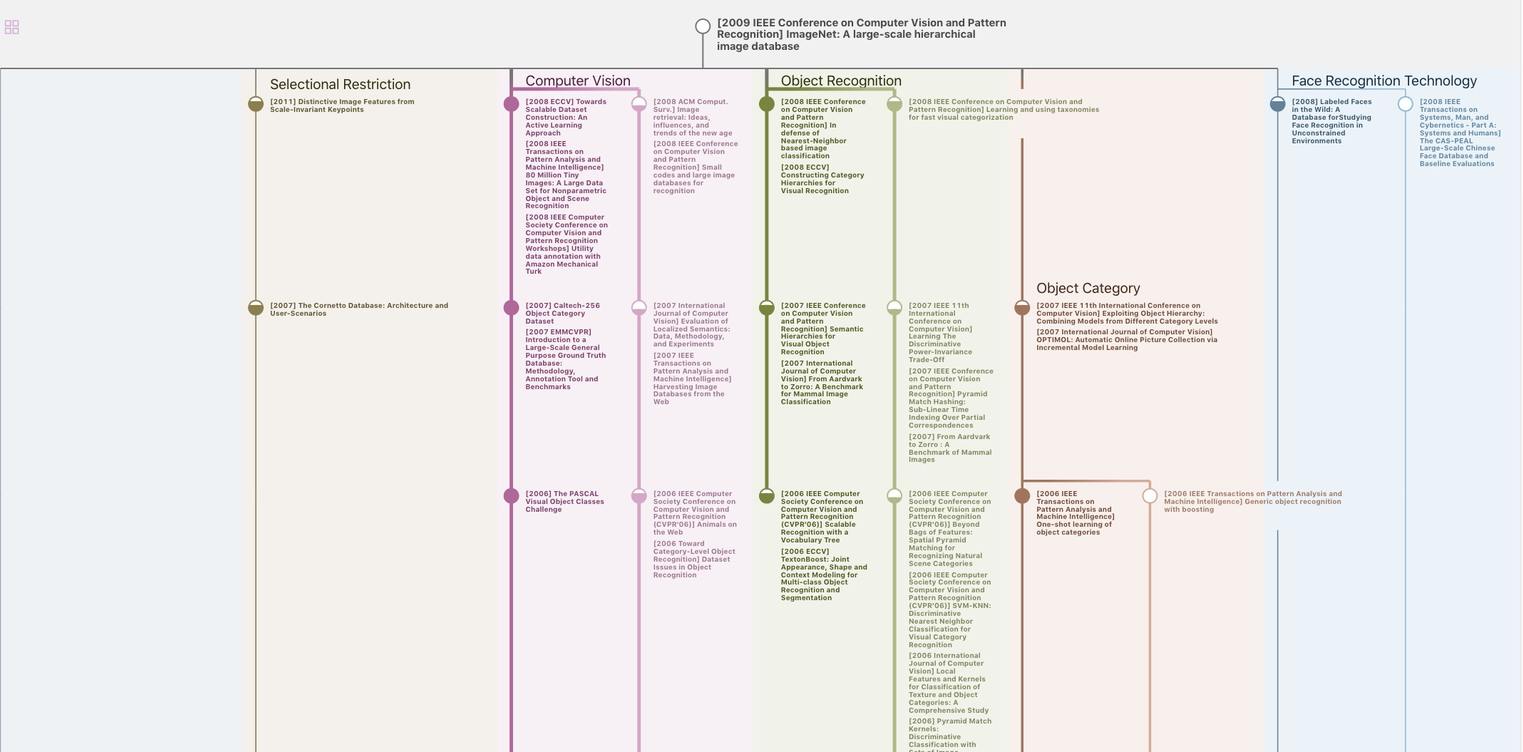
生成溯源树,研究论文发展脉络
Chat Paper
正在生成论文摘要