Uncovering Multivariate Structural Dependency for Analyzing Irregularly Sampled Time Series
MACHINE LEARNING AND KNOWLEDGE DISCOVERY IN DATABASES: RESEARCH TRACK, ECML PKDD 2023, PT V(2023)
摘要
Predictive analytics on Irregularly Sampled Multivariate Time Series (IS-MTS) presents a challenging problem in many real-world applications. Previous methods have primarily focused on incorporating temporal information into prediction while little effort is made to exploit the intrinsic structural information interchange among different IS-MTS at the same or across different timestamps. Recent developments in graph-based learning have shown promise in modeling spatial and structural dependencies of graph data. However, when applied to IS-MTS, they face significant challenges due to the complex data characteristics: 1) variable time intervals between observations; 2) asynchronous time points across dimensions resulting in missing values; 3) a lack of prior knowledge of connectivity structure for information propagation. To address these challenges, we propose a multivariate temporal graph network that coherently captures structural interactions, learns time-aware dependencies, and handles challenging characteristics of IS-MTS data. Specifically, we first develop a multivariate interaction module that handles the frequent missing values and adaptively extracts graph structural relations using a novel reinforcement learning module. Second, we design a correlation-aware neighborhood aggregation mechanism to capture within and across time dependencies and structural interactions. Third, we construct a novel masked time-aware self-attention to explicitly consider timestamp information and interval irregularity for determining optimal attention weights and distinguishing the influence of observation embeddings. Based on an extensive experimental evaluation, we demonstrate that our method outperforms a variety of competitors for the IS-MTS classification task.
更多查看译文
关键词
Irregularly sampled multivariate time series,graph structure learning,temporal attention,multivariate feature interaction
AI 理解论文
溯源树
样例
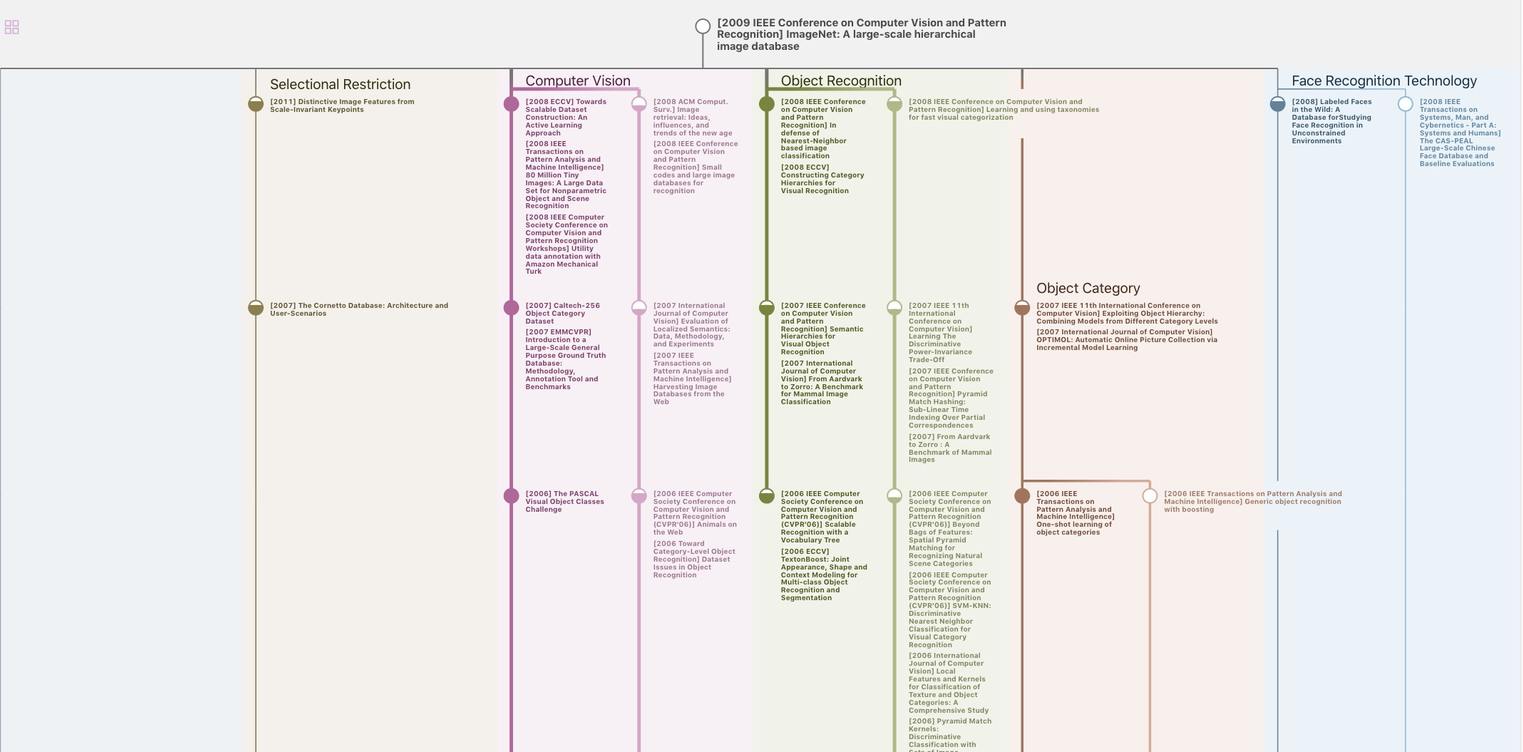
生成溯源树,研究论文发展脉络
Chat Paper
正在生成论文摘要