Meta-HRNet: A High Resolution Network for Coarse-to-Fine Few-Shot Classification
MACHINE LEARNING AND KNOWLEDGE DISCOVERY IN DATABASES: RESEARCH TRACK, ECML PKDD 2023, PT II(2023)
摘要
Fine-grained classification has achieved success with the application of deep learning on large datasets. However, in practical scenarios, fine-grained categories often suffer from a lack of training data due to the difficulty of labeling. Leveraging accessible coarse-grained labeled data provides a promising way to alleviate this challenge, that is, the model learns from a large number of coarse-grained labeled data to perform better on fine-grained classification. In this paper, we focus on this coarse-to-fine few-shot problem and attribute the difficulty of this problem to two factors: the undistinguishable appearance of fine-grained images and the lack of fine-grained training samples. To address the first factor, we demonstrate that high-resolution features can capture more distinctive details that are useful for fine-grained classification tasks. Thus, we construct an improved high-resolution network called Meta-HRNet to capture rich details and filter the crucial detailed information for fine-grained classification. To address the second factor, we train the model by a two-step strategy that combines supervised training and episodic training. During the first training stage, the backbone of Meta-HRNet is optimized to obtain a basic ability of detailed representation. In the second stage, the attention module of the Meta-HRNet is trained to learn and sift key details given a low number of training samples. The effectiveness of our model is verified on four datasets. Experimental results demonstrate that the attention paid to the important details of images contributes to improving the performance of fine-grained classification tasks.
更多查看译文
关键词
fine-grained classification,few-shot learning,high-resolution representation,machine learning
AI 理解论文
溯源树
样例
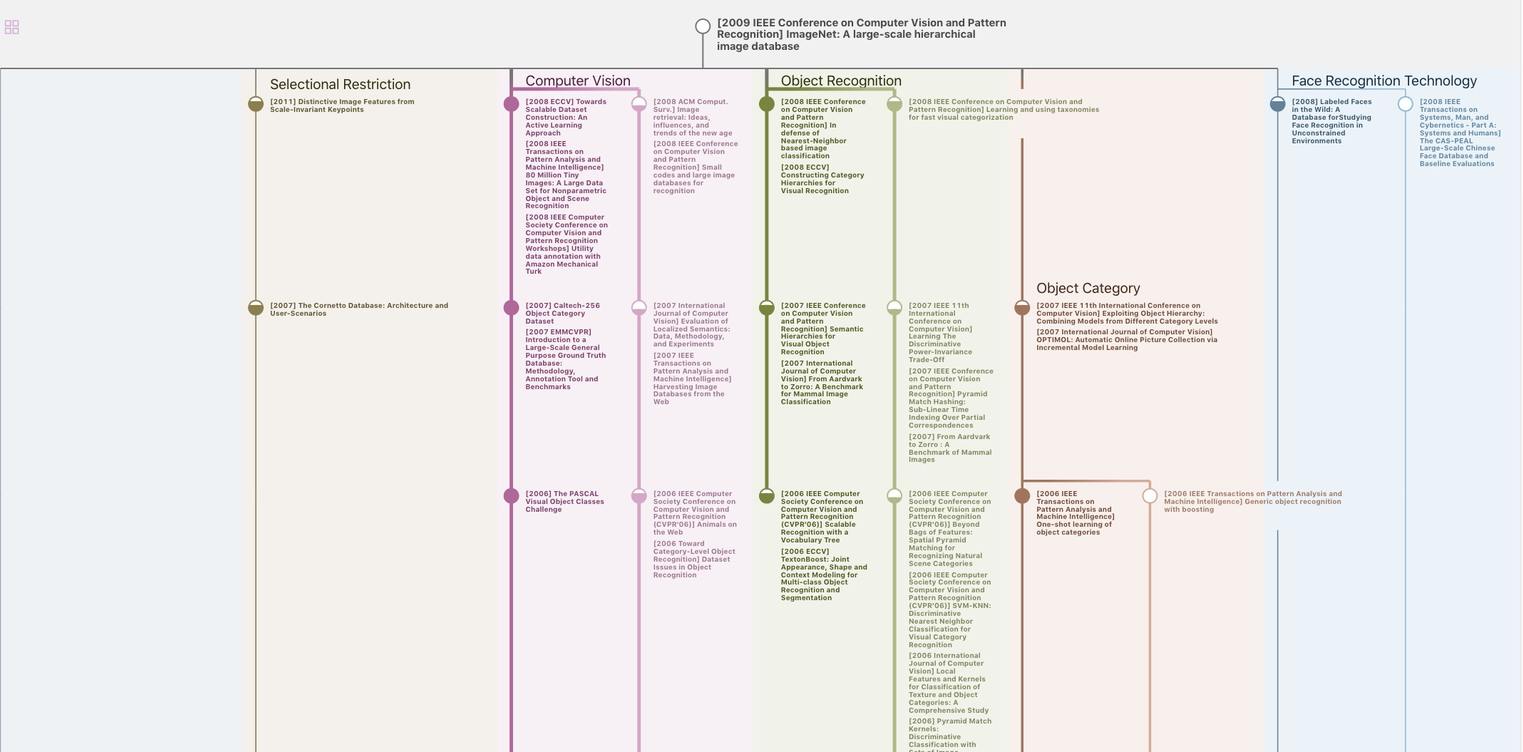
生成溯源树,研究论文发展脉络
Chat Paper
正在生成论文摘要