Targeting the Source: Selective Data Curation for Debiasing NLP Models
MACHINE LEARNING AND KNOWLEDGE DISCOVERY IN DATABASES: RESEARCH TRACK, ECML PKDD 2023, PT II(2023)
摘要
Unjustified social stereotypes have lately been found to taint the predictions of NLP models. Thus, an increasing amount of research focuses on developing methods to mitigate social bias. Most proposed approaches update the parameters of models post-hoc, running the risk of forgetting the predictive task of interest. In this work, we propose a novel way of debiasing NLP models by debiasing and curating their training data. To do so, we propose an unsupervised pipeline to identify which instances in the training data mention stereotypes that tally with the stereotypes encoded in NLP models. Then we either remove or augment these problematic instances, and train NLP models on less biased data. In this pipeline, we propose three methods to excavate stereotypes encoded in models using likelihoods, attention weights and vector representations. Experiments on the tasks of natural language inference, sentiment analysis and question answering suggest that our methods are better at debiasing downstream models than existing techniques.
更多查看译文
关键词
Natural Language Processing,Fairness,Debiasing,Data Curation
AI 理解论文
溯源树
样例
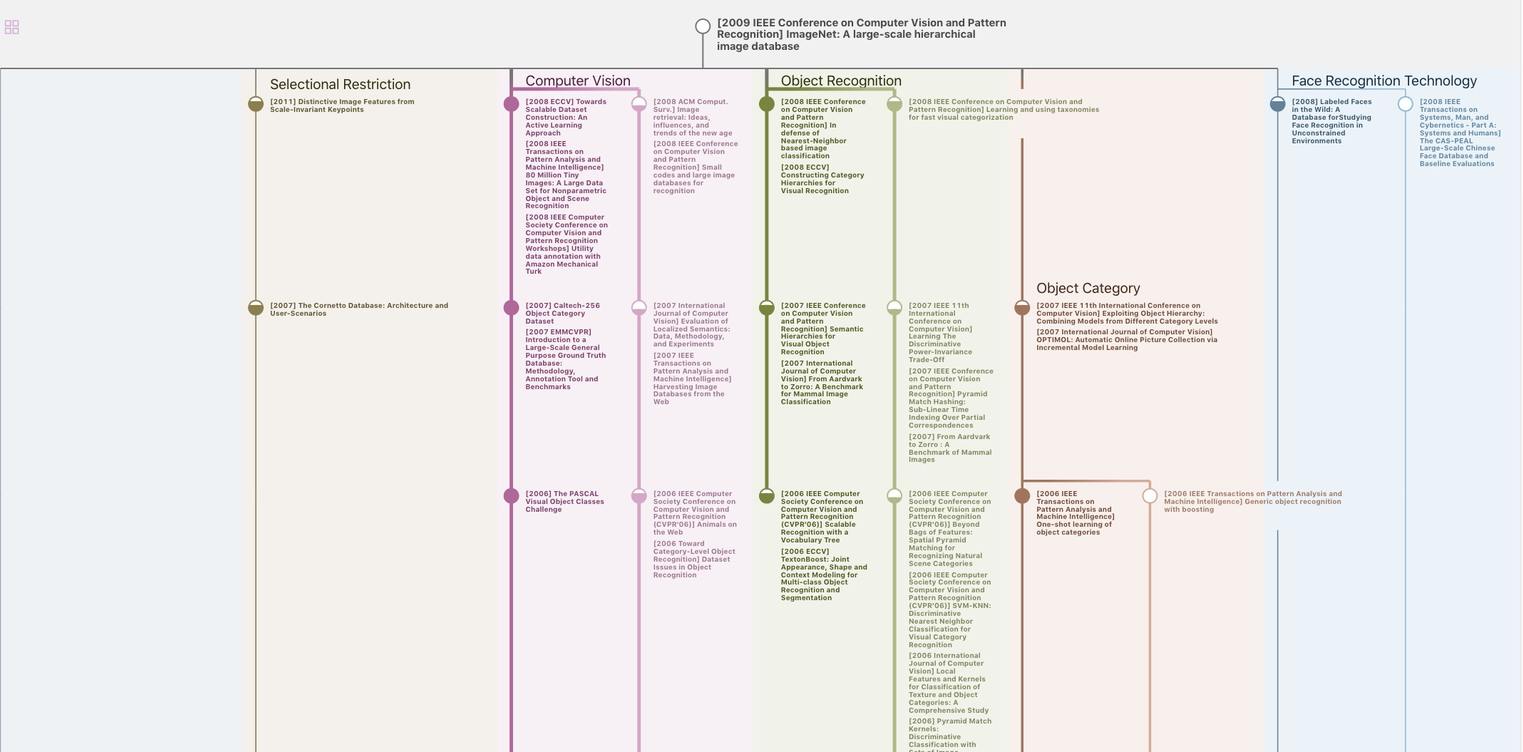
生成溯源树,研究论文发展脉络
Chat Paper
正在生成论文摘要