Contextualised Out-of-Distribution Detection Using Pattern Identification
COMPUTER SAFETY, RELIABILITY, AND SECURITY, SAFECOMP 2023 WORKSHOPS(2023)
摘要
In this work, we propose CODE, an extension of existing work from the field of explainable AI that identifies class-specific recurring patterns to build a robust Out-of-Distribution (OoD) detection method for visual classifiers. CODE does not require any classifier retraining and is OoD-agnostic, i.e., tuned directly to the training dataset. Crucially, pattern identification allows us to provide images from the In-Distribution (ID) dataset as reference data to provide additional context to the confidence scores. In addition, we introduce a new benchmark based on perturbations of the ID dataset that provides a known and quantifiable measure of the discrepancy between the ID and OoD datasets serving as a reference value for the comparison between OoD detection methods.
更多查看译文
关键词
Out-of-distribution detection,Explainable AI,Pattern identification
AI 理解论文
溯源树
样例
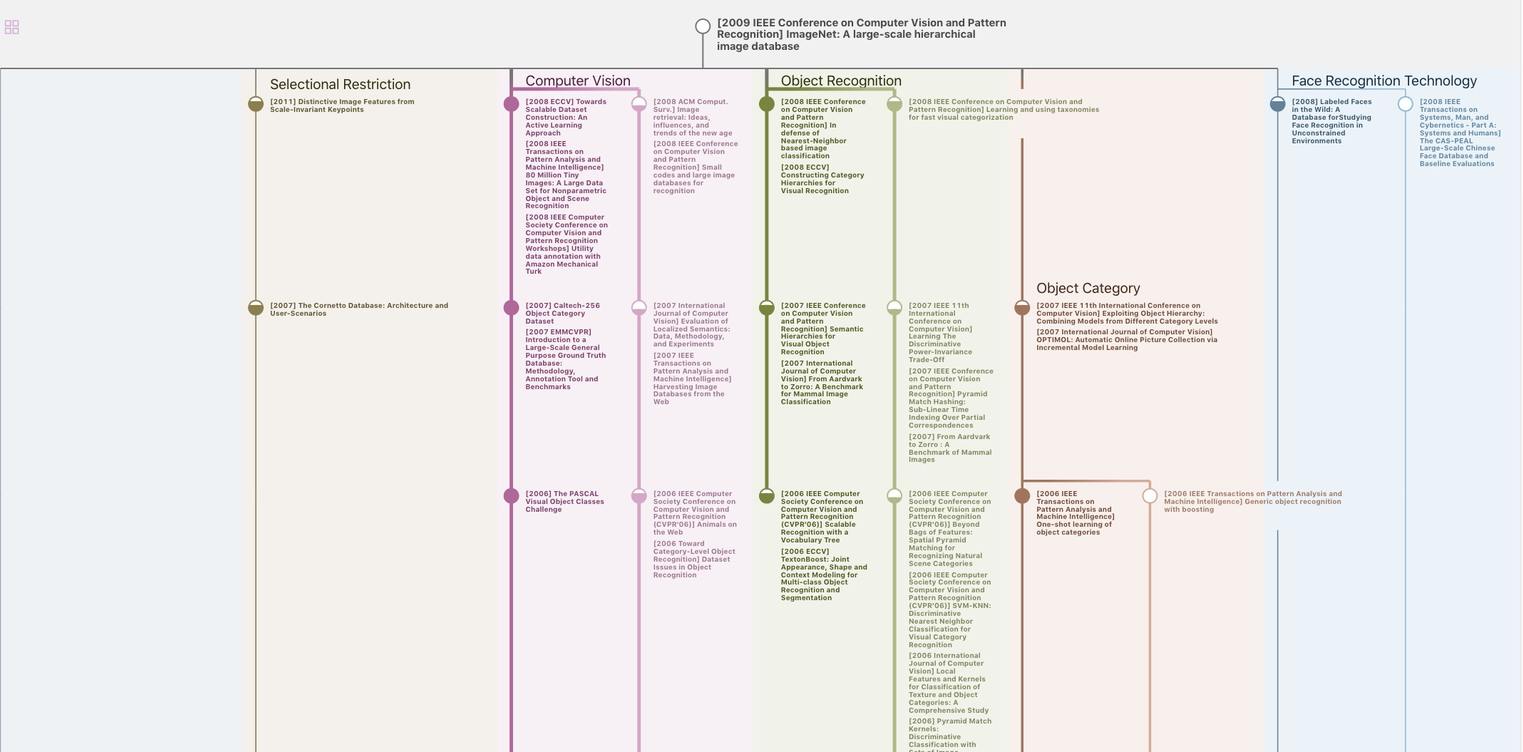
生成溯源树,研究论文发展脉络
Chat Paper
正在生成论文摘要