Benchmarking Classifiers for DDoS Attack Detection in Industrial IoT Networks.
CISIS-ICEUTE(2023)
摘要
In recent years, the proliferation of Internet of Things (IoT) devices has led to an increase in network DDoS attacks. This requires effective methods to classify traffic on IoT networks as benign or DDoS-vulnerable. The present research compares the performance of some of the existing classification models to determine which is better at detecting suspicious packets from an IoT network. We evaluate six different Machine Learning (ML) models: linear, instance-based, SVM, probabilistic, tree-based, and boosted ones. The analyzed dataset contains labeled traffic packets, used to train and test the models on real-life data. Model performance is benchmarked in terms of the standard metrics: accuracy, precision, recall, TN Ratio, and F1-score. The outcomes of this study can be applied to improve IoT network security in Industry 4.0 environments as it provides valuable insights into the most effective ML algorithms to classify IoT network traffic.
更多查看译文
关键词
ddos attack detection,industrial iot networks,benchmarking
AI 理解论文
溯源树
样例
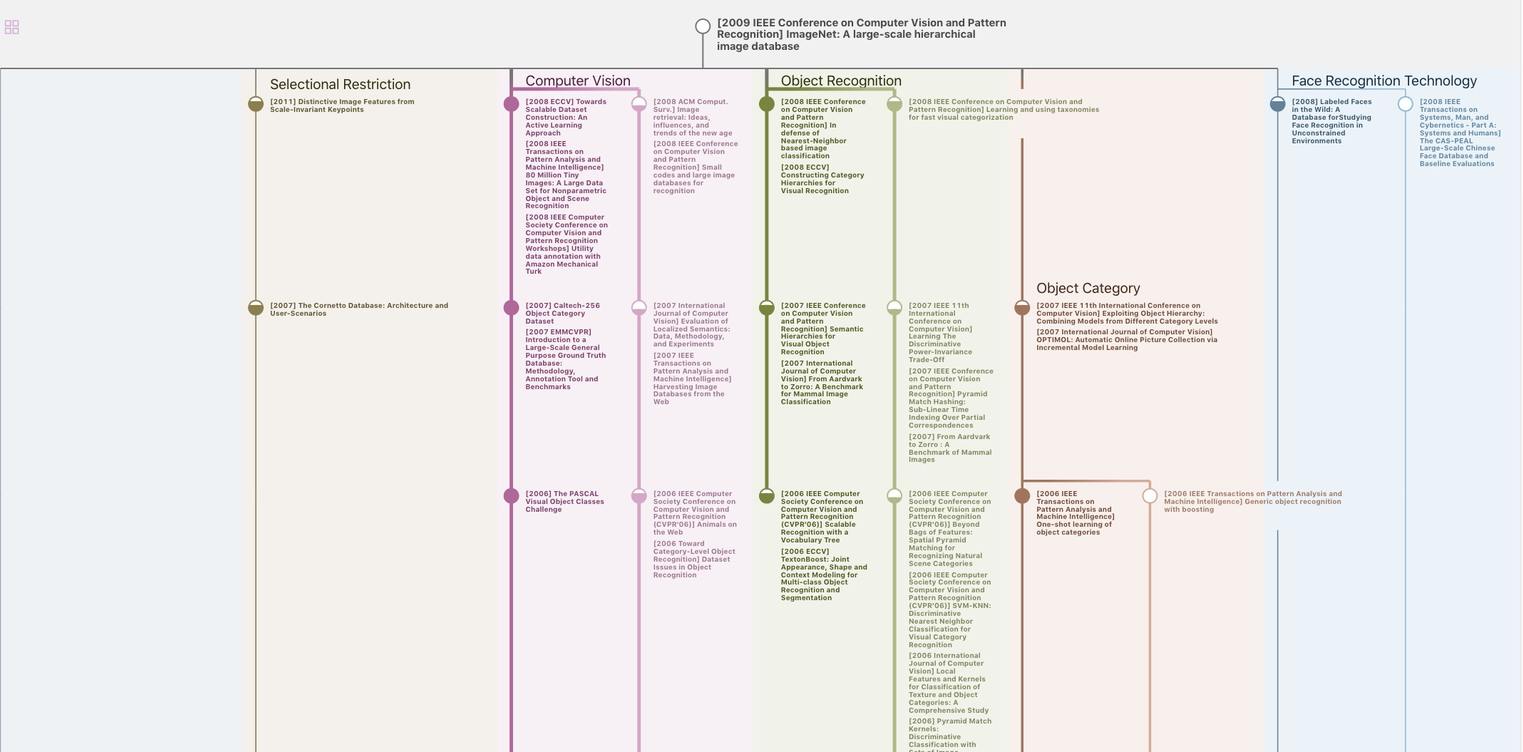
生成溯源树,研究论文发展脉络
Chat Paper
正在生成论文摘要