Two-Step Feature Selection Technique for Secure and Lightweight Internet of Things.
ICCCN(2023)
摘要
With the arrival of the information age, the amount of usable high-dimensional data is rapidly increasing; however, the vast amount of unrefined data may deteriorate the performance of machine learning algorithms. Furthermore, lightweight devices, such as the secure Internet of Things (IoT), have small memory capacity and limited computing power for large data processing; thus, it is difficult to introduce machine learning algorithms to IoT applications. Therefore, it is necessary to develop an efficient learning method that can be used on lightweight devices. This paper proposes a feature selection model consisting of two steps to efficiently reduce the computational complexity of conventional models in the learning process. The proposed two-step feature selection model (TFSM), which performs an exhaustive search for all features by selecting features based on variable importance and then deriving the optimal feature set, significantly reduced complexity, memory usage, and latency with similar accuracy to the greedy model. Particularly, TFSM decreased the complexity by 99.73%, memory usage by 99.97%, and latency by 72.4% compared to the greedy model that uses all features, and improved accuracy by 15.89% compared to random models that randomly perform feature selection.
更多查看译文
关键词
Internet of Things,Lightweight devices,Feature selection,Machine learning,Exhaustive search
AI 理解论文
溯源树
样例
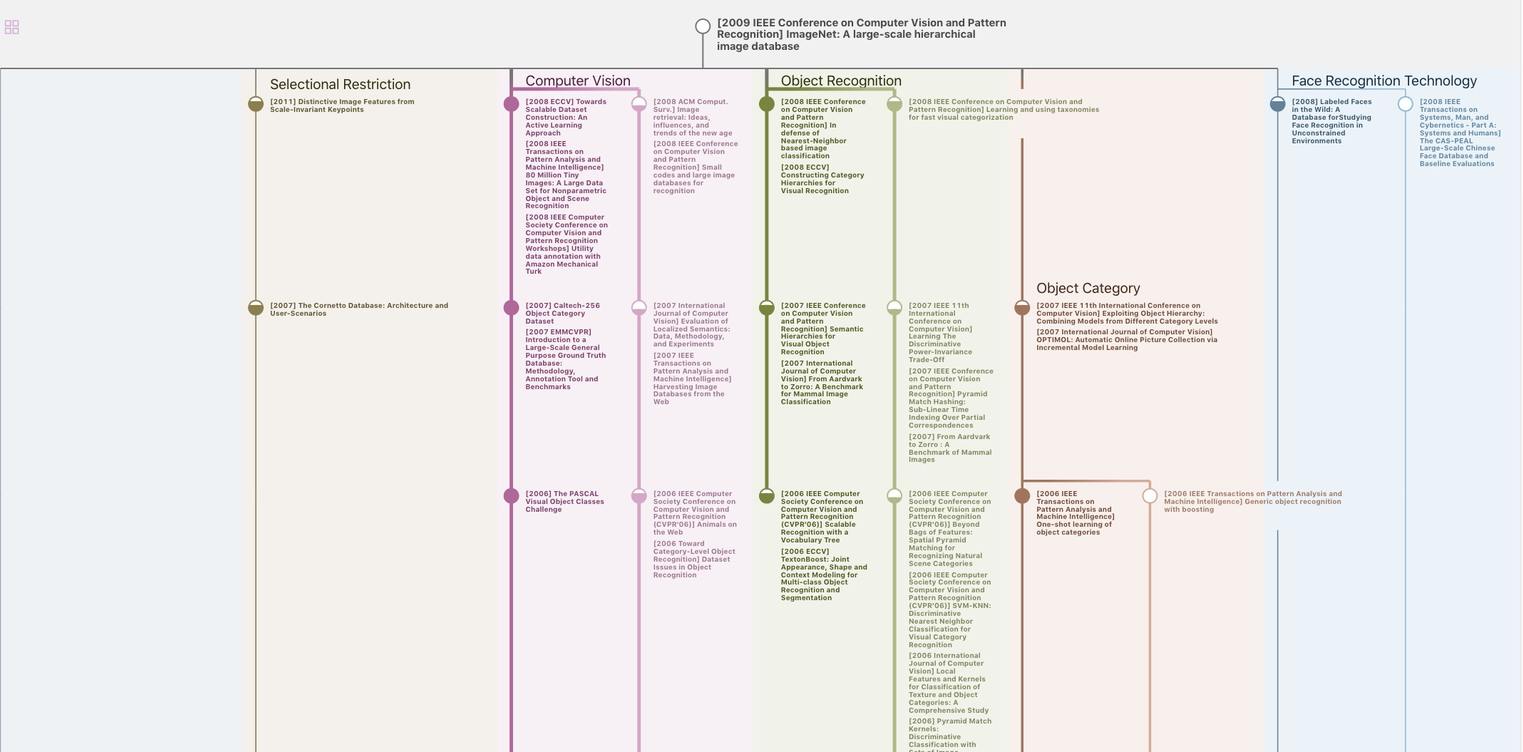
生成溯源树,研究论文发展脉络
Chat Paper
正在生成论文摘要