SecureGAN: Secure Three-Party GAN Training.
ICCCN(2023)
摘要
Generating Adversarial Network (GAN) is a prominent unsupervised learning method that utilizes two competing neural networks to generate realistic data, which has been widely employed in image synthesis and data augmentation. Outsourcing GAN training to cloud servers can significantly reduce the computation load on local devices. Furthermore, in outsourcing settings, training data can be gathered from multiple users, leading to larger amounts of data and, as a result, improved training accuracy. However, outsourcing is associated with privacy risks, as training data often contains sensitive information. To address this problem, we propose SecureGAN, a privacy-preserving framework for GAN that aims to protect the privacy of the training input and output. We implement secure protocols based on replicated secret sharing technology to protect the privacy of the linear and nonlinear layers. We conduct experiments using the MP-SPDZ framework, and the results demonstrate the effectiveness of the proposed protocols.
更多查看译文
关键词
privacy-preserving,secure computation,deep learning,generative adversarial network,cloud computing
AI 理解论文
溯源树
样例
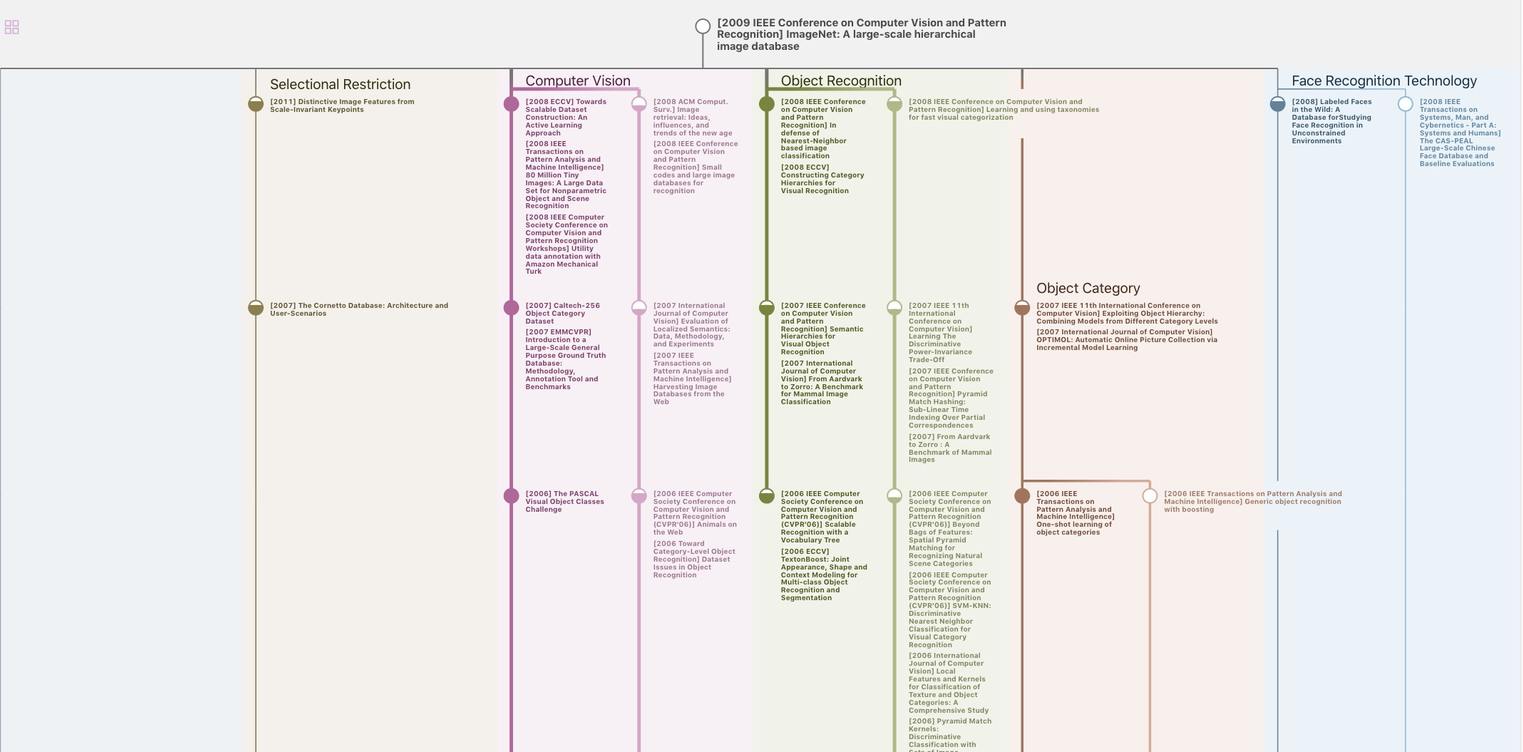
生成溯源树,研究论文发展脉络
Chat Paper
正在生成论文摘要