Exploring Identifiability in Hybrid Models of Cell Signaling Pathways
ADVANCES IN BIOINFORMATICS AND COMPUTATIONAL BIOLOGY, BSB 2023(2023)
摘要
Various processes, including growth, proliferation, migration, and death, mediate the activity of a cell. To better understand these processes, dynamic modeling can be a helpful tool. First-principle modeling provides interpretability, while data-driven modeling can offer predictive performance using models such as neural network, however at the expense of the understanding of the underlying biological processes. A hybrid model that combines both approaches might mitigate the limitations of each of them alone; nevertheless, to this end one needs to tackle issues such as model calibration and identifiability. In this paper, we report a methodology to address these challenges that makes use of a universal differential equation (UDE)-based hybrid modeling, were a partially known, ODE-based, first-principle model is combined with a feedforward neural network-based, data-driven model. We used a synthetic signaling network composed of 38 chemical species and 51 reactions to generate simulated time series for those species, and then defined twelve of those reactions as a partially known first-principle model. A UDE system was defined with this latter and it was calibrated with the data simulated with the whole network. Initial results showed that this approach could identify the missing communication of the partially-known first-principle model with the remainder of the network. Therefore, we expect that this type of hybrid modeling might become a powerful tool to assist in the investigation of underlying mechanisms in cellular systems.
更多查看译文
关键词
Scientific Machine Learning,First-principle Modeling,Universal Differential Equation,Inverse Problem,Cell Signaling Pathway
AI 理解论文
溯源树
样例
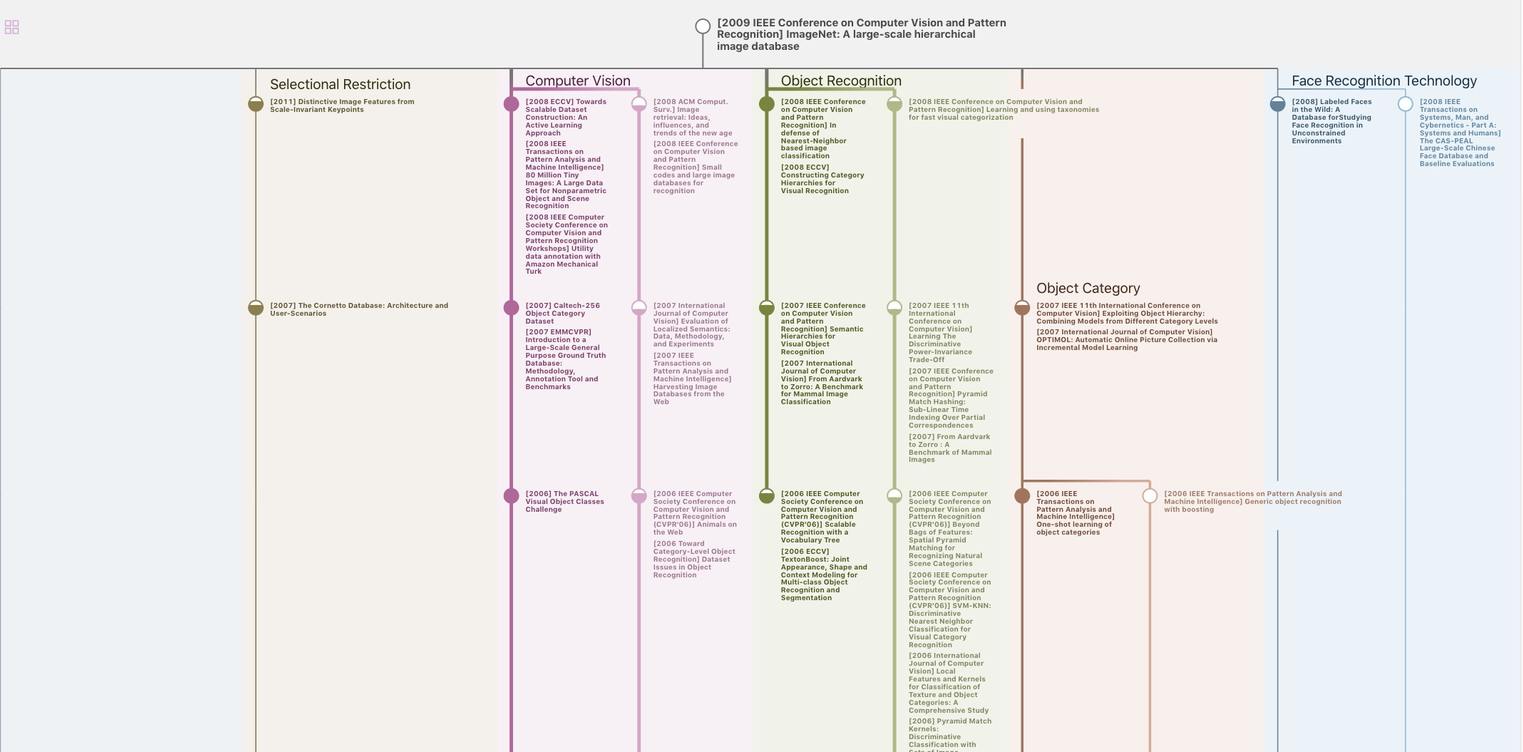
生成溯源树,研究论文发展脉络
Chat Paper
正在生成论文摘要