Utilizing Meta Pseudo Labels for Semantic Segmentation of Targeted Optic Nerve Features
OMIA@MICCAI(2023)
摘要
Evaluating optic nerve cross-sectional images requires significant time and effort by experts to perform. Autonomous systems using deep learning can help to reduce this workload by performing the evaluation automatically, though they tend to require labeled images to train, which can be time and effort intensive to produce. This work utilizes a semi-supervised training algorithm based on the Meta Pseudo Labels (MPL) model, combined with the feature pyramid network architecture used by AxonDeep, to create a highly adaptable network model that can leverage large amounts of unlabeled data and minimal labeled data to train. This opens up the possibility of being able to quickly retrain the network to adapt to different contexts. This method was applied for semantic segmentations of axons within optic nerve cross-sectional images of mice. The tests performed in this work utilized the same network architecture, training data, and post-processing as an existing deeplearning approach, AxonDeep, to establish a fair comparison. The evaluations performed involved training four models using 10%, 25%, 50%, and 100% of the labeled images (n= 26) alongside unlabeled images (n= 50). Results from the test set (n= 18) show that with 10% of the labeled training data, the MPL model was able to achieve a similar Dice score as the AxonDeep model when trained with 100% of the labeled training data, though the axon-count results calculated during post-processing did not achieve a similar level of accuracy from minimal labeled training data.
更多查看译文
关键词
targeted optic nerve features,meta pseudo labels,semantic segmentation
AI 理解论文
溯源树
样例
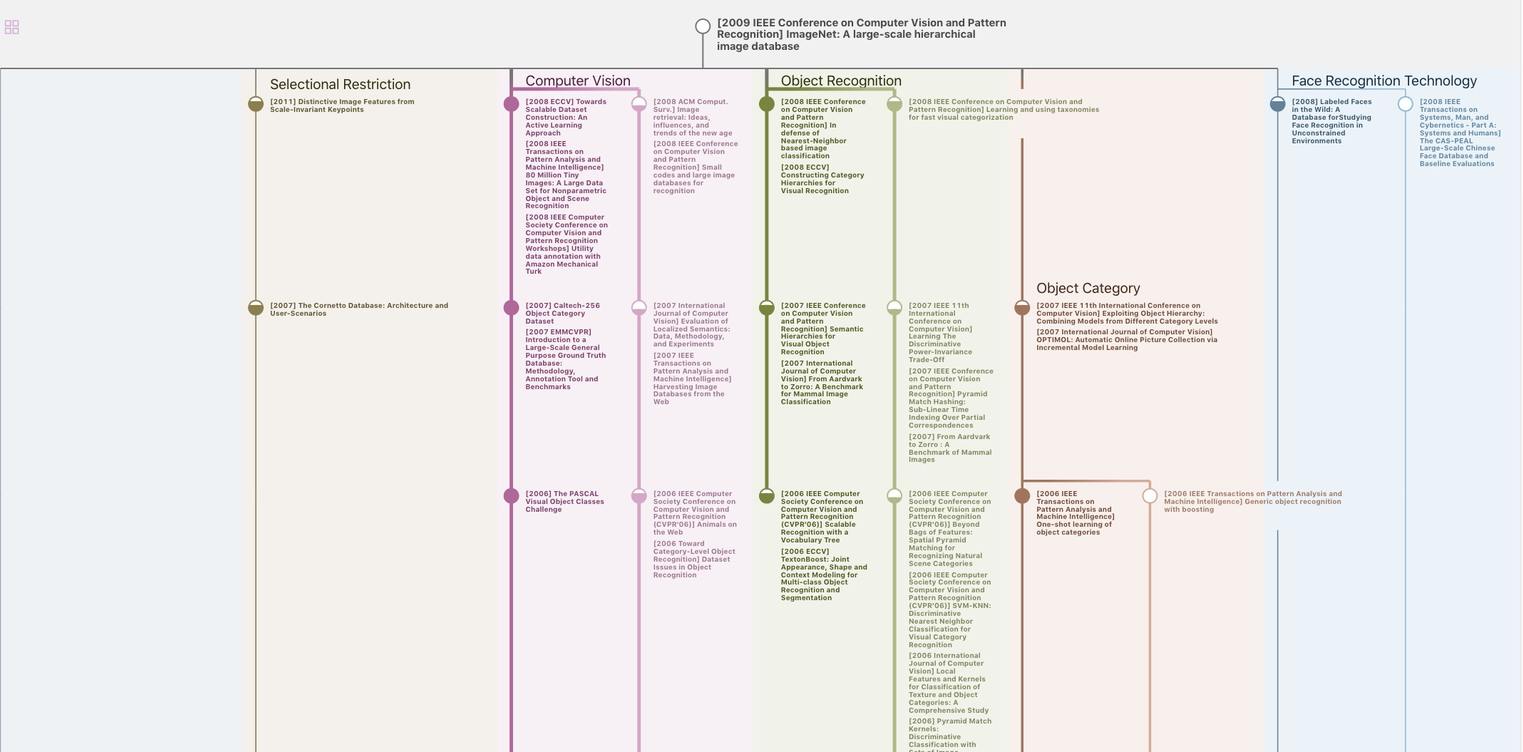
生成溯源树,研究论文发展脉络
Chat Paper
正在生成论文摘要