Generalizing an Improved GrowCut Algorithm for Mammography Lesion Detection.
HAIS(2023)
摘要
In the past five years, 7.8 million women were diagnosed with breast cancer. Breast cancer is curable if it is discovered in early stages. Therefore, mammography screening is essential. But, since interpretation can prove difficult, various automated interpretation systems have been proposed so far. A crucial step of the interpretation process is segmentation: identifying the region of interest. In this paper we aim to evaluate an improved version of the GrowCut algorithm - which reduces both the human intervention and the computational time, while preserving a high level of accuracy, and analyzes three possibilities of avoiding the need for initial background seeds: (1) automatically generating seeds inside the breast, (2) using the mammogram’s black pixels and (3) not using background seeds - on a different, much larger dataset than the one initially used for experiments, in order to analyze the impact of a dataset’s particularities and the influence of different seed types on the segmentation results, and to validate the initial conclusions. However, the experimental results presented in this paper do not only validate the premises, but also demonstrate that the improved version is a generic algorithm which can be used on any dataset with different types of background seeds.
更多查看译文
关键词
mammography,improved growcut algorithm,detection
AI 理解论文
溯源树
样例
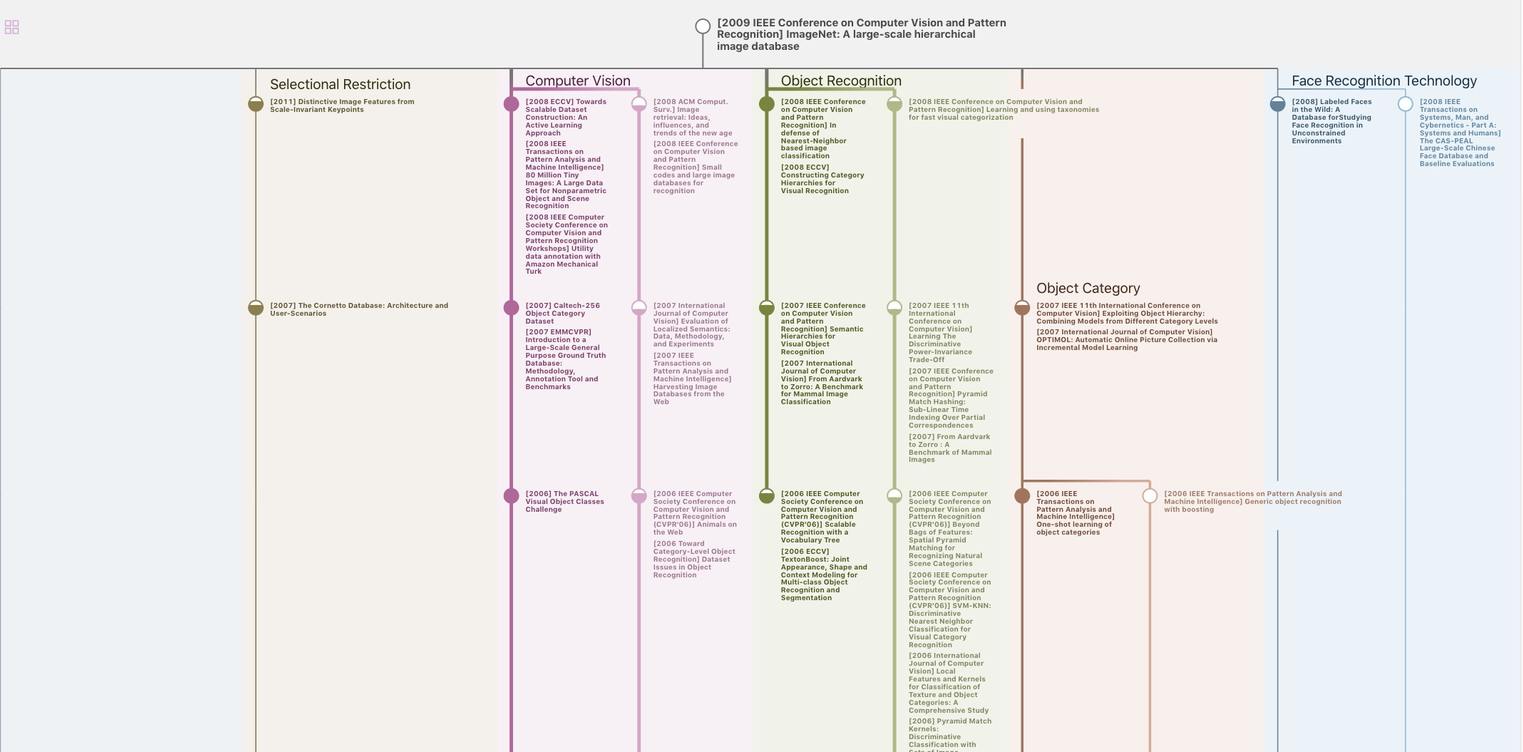
生成溯源树,研究论文发展脉络
Chat Paper
正在生成论文摘要