Counteracting Modeling Attacks using Hardware-based Dynamic Physical Unclonable Function
CSR(2023)
摘要
The widespread adoption of Internet of Things (IoT) devices across various application domains has significantly improved quality of life. However, the resource-constrained, heterogeneous, and low-power nature of these devices poses challenges in ensuring secure communication and authenticity. Physical Unclonable Functions (PUFs) provide a solution by creating a unique and device-specific identity through manufacturing process variations without requiring additional resources. To authenticate IoT devices, a challenge-response pair (CRP) is generated based on the unique characteristics of each device. However, the CRPs generated by PUFs often exhibit high correlation, making them vulnerable to modeling attacks. Despite the proposal of numerous intricate PUF architectures, such as XOR PUF and Interpose PUF, the advancement in machine learning algorithms has enabled modeling attacks on these PUFs. This work presents a hardware-based dynamic PUF and evaluates its performance on field programmable gate arrays (FPGAs). The dynamic nature of the proposed PUF architecture makes it challenging for prevalent machine learning models to predict accurate PUF responses. The research also compares the efficacy of logistic regression and multilayer perceptron-based modeling attacks on Arbiter PUF and XOR PUF architectures. The experimental findings reveal that the dynamic PUF outperforms the other two PUFs against machine learning-based attacks. These results suggest that the dynamic PUF architecture is viable for IoT applications.
更多查看译文
关键词
Hardware Security,Internet of Things,Physical Unclonable Functions (PUF),Machine Learning,Artificial Neural Networks,Modeling Attack Attacks,Dynamic PUF
AI 理解论文
溯源树
样例
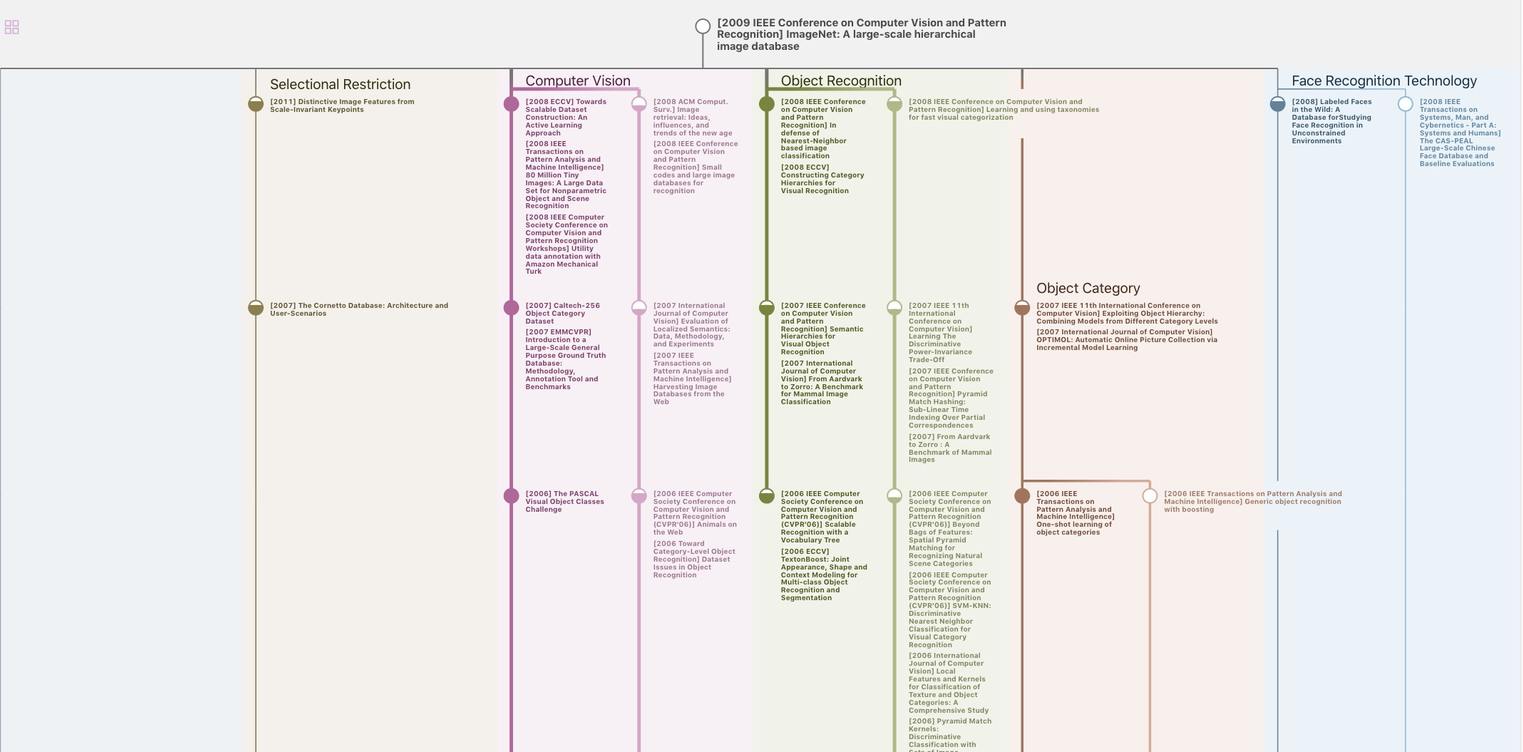
生成溯源树,研究论文发展脉络
Chat Paper
正在生成论文摘要