Multi-label Generalized Zero-Shot Learning Using Identifiable Variational Autoencoders
EXTENDED REALITY, XR SALENTO 2023, PT II(2023)
摘要
Multi-label Zero-Shot Learning (ZSL) is an extension of traditional single-label ZSL, where the objective is to accurately classify images containing multiple unseen classes that are not available during training. Current techniques depends on attention mechanisms and Generative Adversarial Networks (GAN) to address multi-label ZSL and Generalized Zero-Shot Learning (GZSL) challenge. However, generating features for both multi-label ZSL and GZSL in the context of disentangled representation learning remains unexplored. In this paper, we propose an identifiable Variational Autoencoder (iVAE) based generative framework for multi-label ZSL and GZSL. The main idea of our proposed approach is to learn disentangled representations for generating semantically consistent multi-label features using an attribute-level feature fusion technique. We perform comprehensive experiments on two benchmark datasets, NUS-WIDE and MS COCO, for both multi-label ZSL and GZSL. Furthermore, disentangled representation learning for both multi-label ZSL and GZSL on standard datasets achieves commendable performance as compared to existing methods.
更多查看译文
关键词
Attribute-Level Feature Fusion,Zero-Shot Learning,Disentangled Representation Learning,Generalized Zero-Shot Learning
AI 理解论文
溯源树
样例
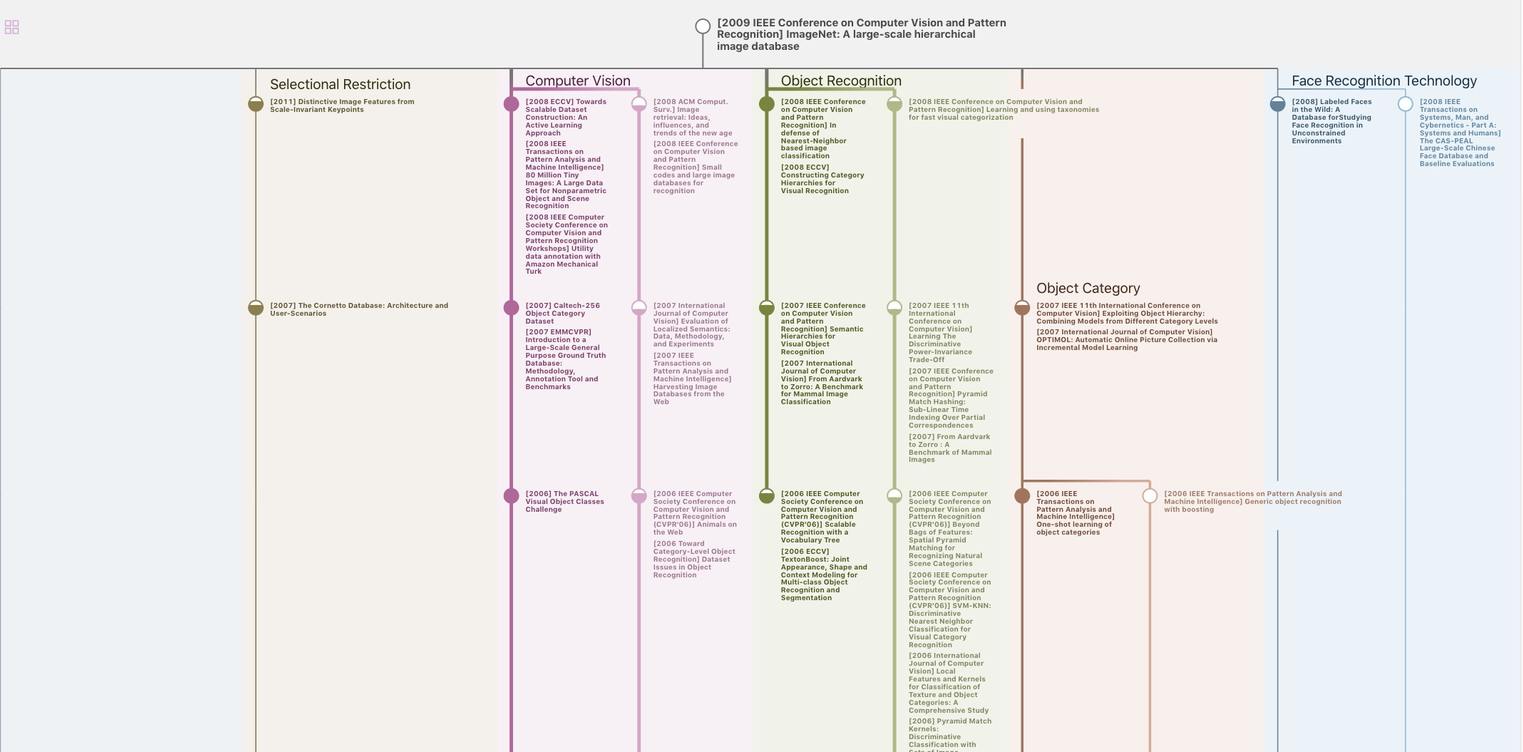
生成溯源树,研究论文发展脉络
Chat Paper
正在生成论文摘要