Predicting Patient's Willingness for Colorectal Cancer Screening Practices Using Machine Learning Classifiers.
ICIT(2023)
Abstract
Diagnosing and treating colorectal cancer (CRC) at its earlier stages is considered a significant public health issue since it is the second and third most commonly diagnosed cancer in both genders. However, early identification and screening of CRC can increase the survival rate to more than 90%. Employing machine learning to study individual predictors (socio-demographics, level of knowledge, and health beliefs) can affect the willingness to screen for CRC and enhance screening rates. This paper presents the application of five machine learning classifiers for predicting the patient's willingness for CRC screening. The classifiers are tested on a dataset obtained from Jordan University Hospital (JUH) and Albasheer Hospital in Amman, Jordan. The dataset is used to train and test the machine learning models with attributes related to socio-demographics, level of knowledge, and health beliefs. By applying 10-fold cross-validation, 90.9% is the best-obtained accuracy using Random Forest (RF) classifier compared to the accuracies achieved by Multi-layer Perceptron (MLP) Neural Network, Logistic Regression (LR), Random Forest (RF), J48 with Decorate algorithm, and multi-objective evolutionary fuzzy classifier. Additionally, this paper presents the importance of the attributes obtained by the RF. The results show that the level of knowledge and health beliefs impact the prediction accuracy almost as well as the socio-demographic attributes, where omitting them decreases the accuracy.
MoreTranslated text
Key words
Colorectal Cancer Screening,Logistic Regression,Machine Learning,Multi-layer Perceptron,Prediction Models,Random Forest
AI Read Science
Must-Reading Tree
Example
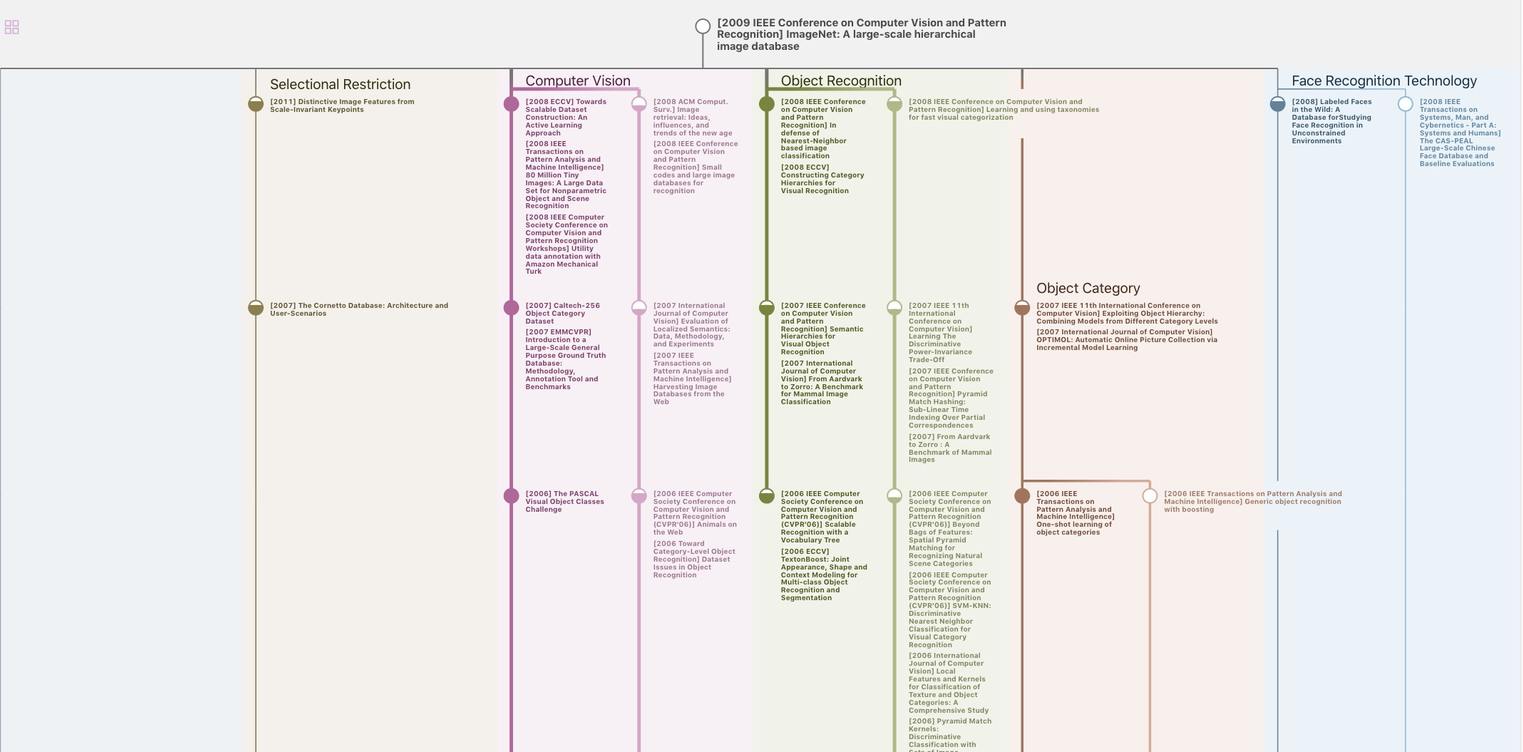
Generate MRT to find the research sequence of this paper
Chat Paper
Summary is being generated by the instructions you defined