Fault Detection in Biological Methanation Process Using Machine Learning: A Comparative Study of Different Algorithms.
SOCO (1)(2023)
Abstract
In this paper we present a study that evaluates different machine learning models for fault detection based on the optimal operation of the biological methanation process. The optimal operation has been obtained from a multi-objective dynamic optimization based on an extended model of the anaerobic digestion model (ADM1 ME). Two datasets have been generated for the ADM1 ME model by generating disturbances on the inlet liquid flow rate (dataset 1) and the inlet gas flow rate (dataset 2). Variations of ±10, ±15, and ±20 $$\%$$ of both optimal inlets have been assumed. These datasets have been used to train several algorithms: decision tree CART, Random Forest (RF), Gaussian Naive Bayes (GNB), k-Nearest Neighbors (KNN), Quadratic Discriminant Analysis (QDA), and Support Vector Machine (SVM). In dataset 1, CART, RF, and Radial Basis Function (RBF) SVM have achieved accuracies higher than 0.90 and 0.85 in the training and test, respectively. In dataset 2, accuracies higher than 0.90 and 0.87 have been obtained for the RF, QDA, and RBF SVM models in the training and test, respectively.
MoreTranslated text
Key words
biological methanation process,fault detection,machine learning,algorithms
AI Read Science
Must-Reading Tree
Example
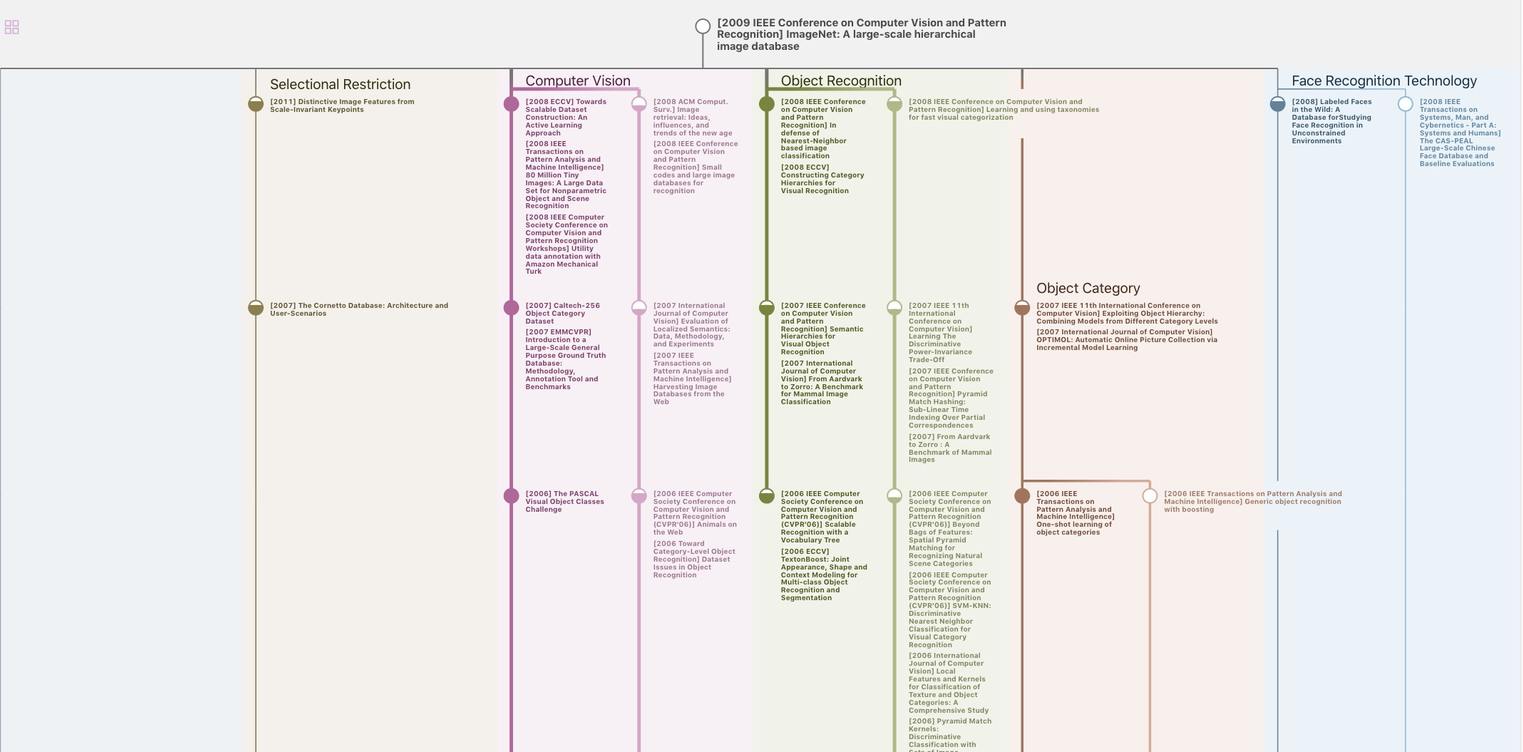
Generate MRT to find the research sequence of this paper
Chat Paper
Summary is being generated by the instructions you defined