HammerDodger: A Lightweight Defense Framework against RowHammer Attack on DNNs.
DAC(2023)
摘要
RowHammer attacks have become a serious security problem on deep neural networks (DNNs). Some carefully induced bit-flips degrade the prediction accuracy of DNN models to random guesses. This work proposes a lightweight defense framework that detects and mitigates adversarial bit-flip attacks. We employ a dynamic channel-shuffling obfuscation scheme to present moving targets to the attack, and develop a logits-based model integrity monitor with negligible performance loss. The parameters and architecture of DNN models remain unchanged, which ensures lightweight deployment and makes the framework compatible with commodity models. We demonstrate that our framework can protect various DNN models against RowHammer attacks.
更多查看译文
关键词
Neural networks, Computer security, Fault protection
AI 理解论文
溯源树
样例
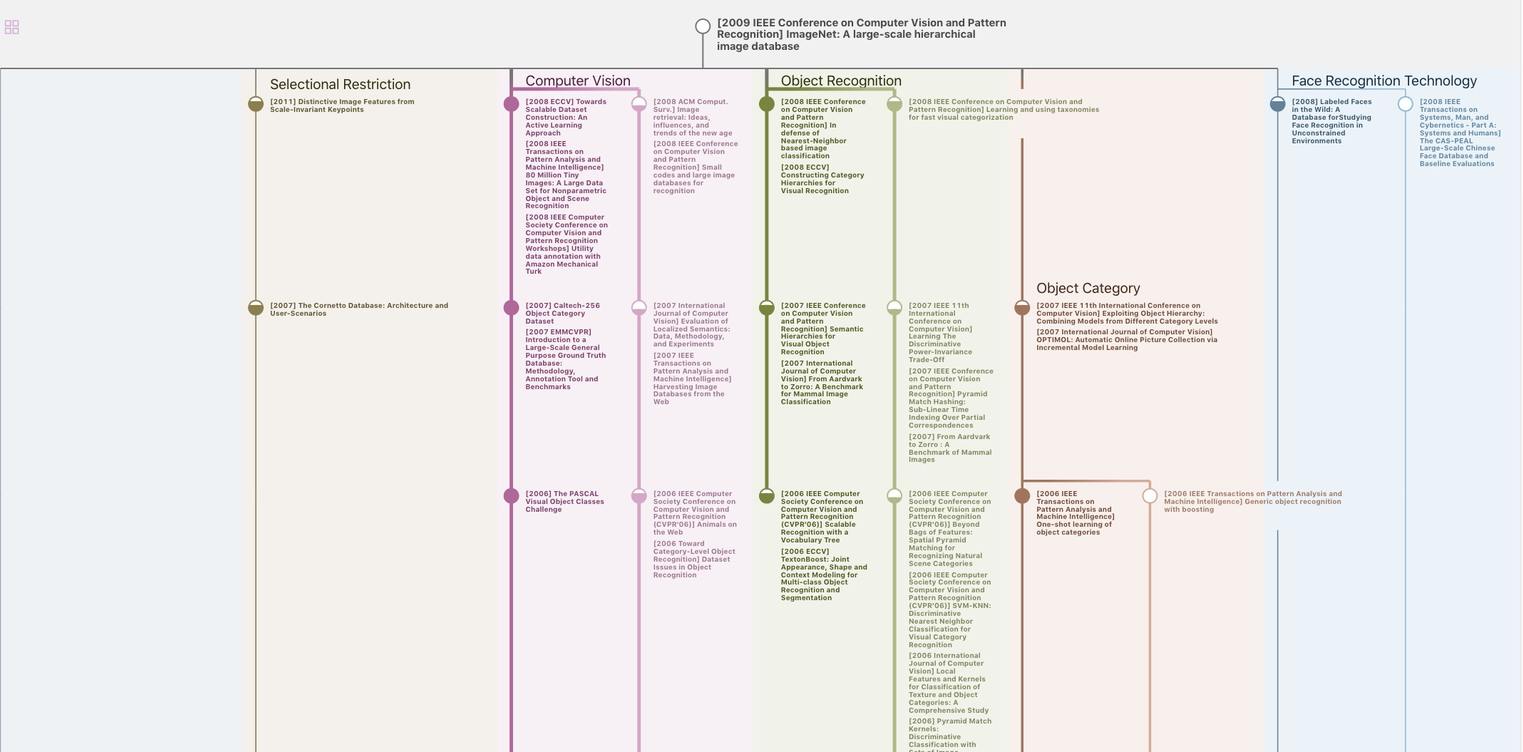
生成溯源树,研究论文发展脉络
Chat Paper
正在生成论文摘要