Accelerating Sparse Attention with a Reconfigurable Non-volatile Processing-In-Memory Architecture
DAC(2023)
摘要
Attention-based neural networks have shown superior performance in a wide range of tasks. Non-volatile processing-in-memory (NVPIM) architecture shows its great potential to accelerate the dense attention model. However, the unique unstructured and dynamic sparsity pattern in the sparse attention model challenges the mapping efficiency of the NVPIM architecture, as the conventional NVPIM architecture uses a vector-matrix-multiplication primitives. In this paper, we propose a NVPIM architecture to accelerate a dynamic and unstructured sparse computation in the sparse attention. We aim to improve the mapping efficiency for both SDDMM and SpMM by introducing two vector-based primitives with a reconfigurable NVPIM bank. Further, based on our reconfigurable NVPIM bank, we further propose a hybrid stationary data flow to hide the latency. Our evaluation result shows that, over previous NVPIM accelerators, our design could deliver up to 12.36× performance improvement and 3.4× energy efficiency improvement on a range of vision and language tasks.
更多查看译文
关键词
3.4× energy efficiency improvement,conventional NVPIM architecture,dense attention model,dynamic computation,dynamic sparsity pattern,mapping efficiency,neural networks,previous NVPIM accelerators,processing-in-memory architecture,reconfigurable NVPIM bank,sparse attention model,unique unstructured sparsity pattern,unstructured sparse computation,vector-based primitives,vector-matrix-multiplication primitives
AI 理解论文
溯源树
样例
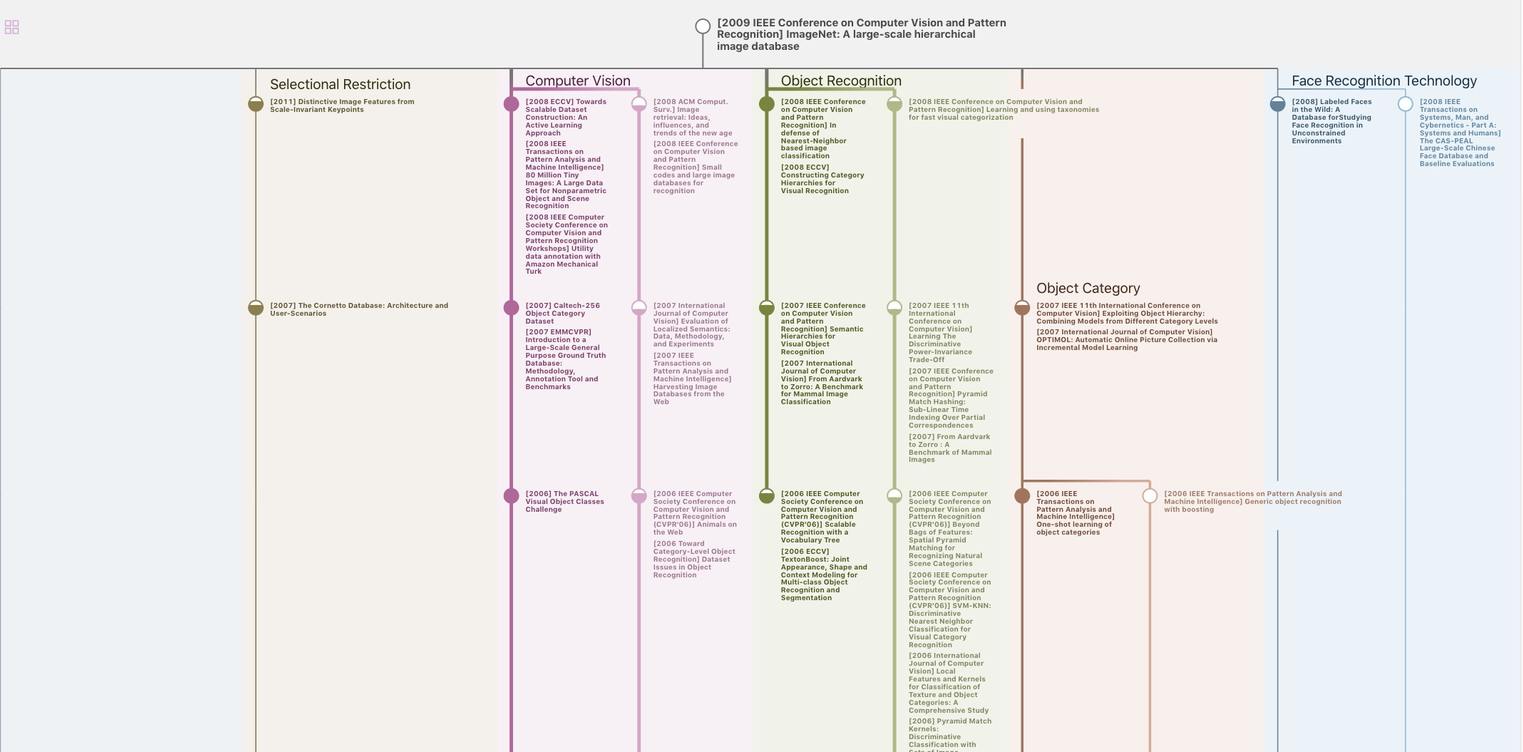
生成溯源树,研究论文发展脉络
Chat Paper
正在生成论文摘要