A self-training algorithm based on the two-stage data editing method with mass-based
Neural networks : the official journal of the International Neural Network Society(2023)
Abstract
A self-training algorithm is a classical semi-supervised learning algorithm that uses a small number of labeled samples and a large number of unlabeled samples to train a classifier. However, the existing self training algorithms consider only the geometric distance between data while ignoring the data distribution when calculating the similarity between samples. In addition, misclassified samples can severely affect the performance of a self-training algorithm. To address the above two problems, this paper proposes a self training algorithm based on data editing with mass-based dissimilarity (STDEMB). First, the mass matrix with the mass-based dissimilarity is obtained, and then the mass-based local density of each sample is determined based on its k nearest neighbors. Inspired by density peak clustering (DPC), this study designs a prototype tree based on the prototype concept. In addition, an efficient two-stage data editing algorithm is developed to edit misclassified samples and efficiently select high-confidence samples during the self-training process. The proposed STDEMB algorithm is verified by experiments using accuracy and F-score as evaluation metrics. The experimental results on 18 benchmark datasets demonstrate the effectiveness of the proposed STDEMB algorithm.
MoreTranslated text
Key words
Self-training algorithm, Mass-based dissimilarity, Data editing, Relative node set
AI Read Science
Must-Reading Tree
Example
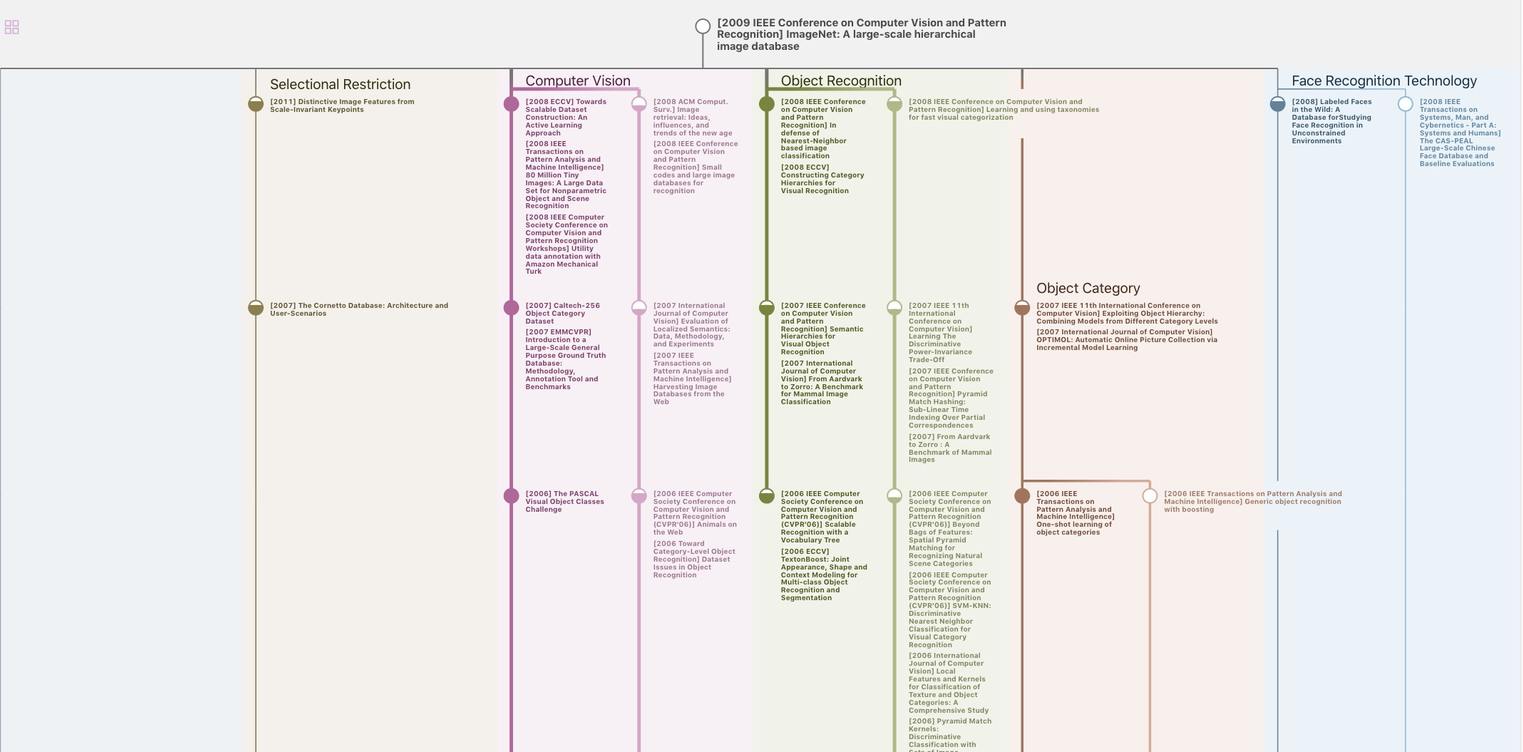
Generate MRT to find the research sequence of this paper
Chat Paper
Summary is being generated by the instructions you defined