Degradation-Aware Self-Attention Based Transformer for Blind Image Super-Resolution
IEEE TRANSACTIONS ON MULTIMEDIA(2024)
摘要
Compared to CNN-based methods, Transformer-based methods achieve impressive image restoration outcomes due to their ability to model remote dependencies. However, how to apply Transformer-based methods to the field of blind super-resolution (SR) and further make an SR network adaptive to degradation information is still an open problem. In this paper, we propose a new degradation-aware self-attention-based Transformer model, where we incorporate contrastive learning into the Transformer network for learning the degradation representations of input images with unknown noise. In particular, we integrate both CNN and Transformer components into the SR network, where we first use the CNN modulated by the degradation information to extract local features, and then employ the degradation-aware Transformer to extract global semantic features. We apply our proposed model to several popular large-scale benchmark datasets for testing, and achieve the state-of-the-art performance compared to existing methods. In particular, our method yields a PSNR of 32.43 dB on the Urban100 dataset at x2 scale, 0.94 dB higher than DASR, and 26.62 dB on the Urban100 dataset at x4 scale, 0.26 dB improvement over KDSR, setting a new benchmark in this area.
更多查看译文
关键词
Super-resolution,transformer,degradation-aware self-attention,contrastive learning
AI 理解论文
溯源树
样例
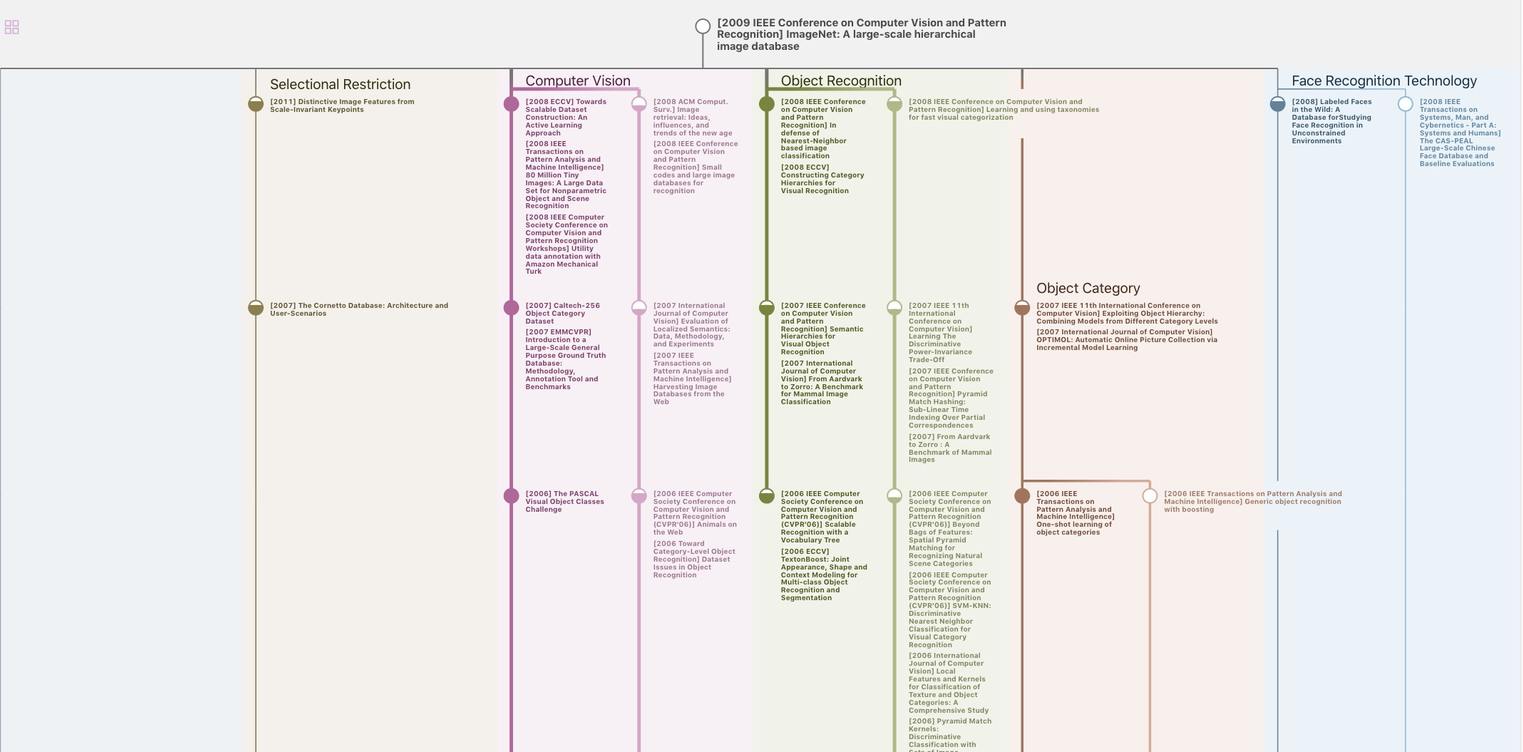
生成溯源树,研究论文发展脉络
Chat Paper
正在生成论文摘要