A Deeply Supervised Semantic Segmentation Method Based on GAN
CoRR(2023)
摘要
In recent years, the field of intelligent transportation has witnessed rapid advancements, driven by the increasing demand for automation and efficiency in transportation systems. Traffic safety, one of the tasks integral to intelligent transport systems, requires accurately identifying and locating various road elements, such as road cracks, lanes, and traffic signs. Semantic segmentation plays a pivotal role in achieving this task, as it enables the partition of images into meaningful regions with accurate boundaries. In this study, we propose an improved semantic segmentation model that combines the strengths of adversarial learning with state-of-the-art semantic segmentation techniques. The proposed model integrates a generative adversarial network (GAN) framework into the traditional semantic segmentation model, enhancing the model's performance in capturing complex and subtle features in transportation images. The effectiveness of our approach is demonstrated by a significant boost in performance on the road crack dataset compared to the existing methods, \textit{i.e.,} SEGAN. This improvement can be attributed to the synergistic effect of adversarial learning and semantic segmentation, which leads to a more refined and accurate representation of road structures and conditions. The enhanced model not only contributes to better detection of road cracks but also to a wide range of applications in intelligent transportation, such as traffic sign recognition, vehicle detection, and lane segmentation.
更多查看译文
关键词
Generative Adversarial Networks,Semantic Segmentation,Traffic Safety,Intelligent Transportation,Intelligent Transportation Systems,Semantic Segmentation Models,Vehicle Detection,Deep Learning,Convolutional Neural Network,Computer Vision,Input Image,Image Pixels,Pedestrian,Intersection Over Union,Dice Similarity Coefficient,Segmentation Performance,Adversarial Training,Generator Output,Fully Convolutional Network,Adversarial Attacks,Adversarial Examples,Fast Gradient Sign Method,Deep Supervision,Segmentation-based Methods,Decoding Performance,Atrous Spatial Pyramid Pooling,Output Resolution,Neural Network,Segmentation Results,Machine Learning
AI 理解论文
溯源树
样例
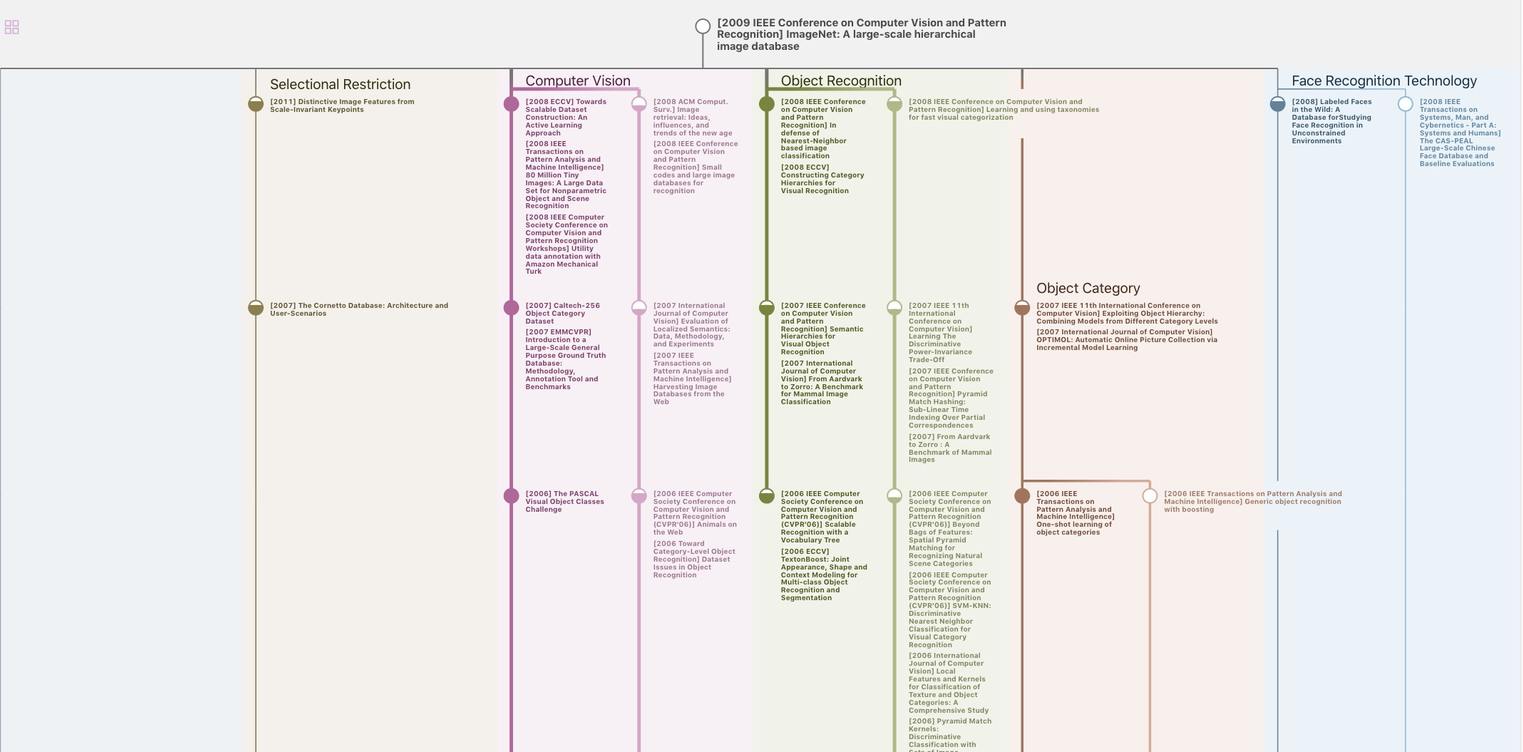
生成溯源树,研究论文发展脉络
Chat Paper
正在生成论文摘要