OpenIncrement: A Unified Framework for Open Set Recognition and Deep Class-Incremental Learning
2023 IEEE/CVF INTERNATIONAL CONFERENCE ON COMPUTER VISION WORKSHOPS, ICCVW(2023)
摘要
In most works on deep incremental learning research, it is assumed that novel samples are pre-identified for neural network retraining. However, practical deep classifiers often misidentify these samples, leading to erroneous predictions. Such misclassifications can degrade model performance. Techniques like open set recognition offer a means to detect these novel samples, representing a significant area in the machine learning domain. In this paper, we introduce a deep class-incremental learning framework integrated with open set recognition. Our approach refines class-incrementally learned features to adapt them for distance-based open set recognition. Experimental results validate that our method outperforms state-of-the-art incremental learning techniques and exhibits superior performance in open set recognition compared to baseline methods.
更多查看译文
AI 理解论文
溯源树
样例
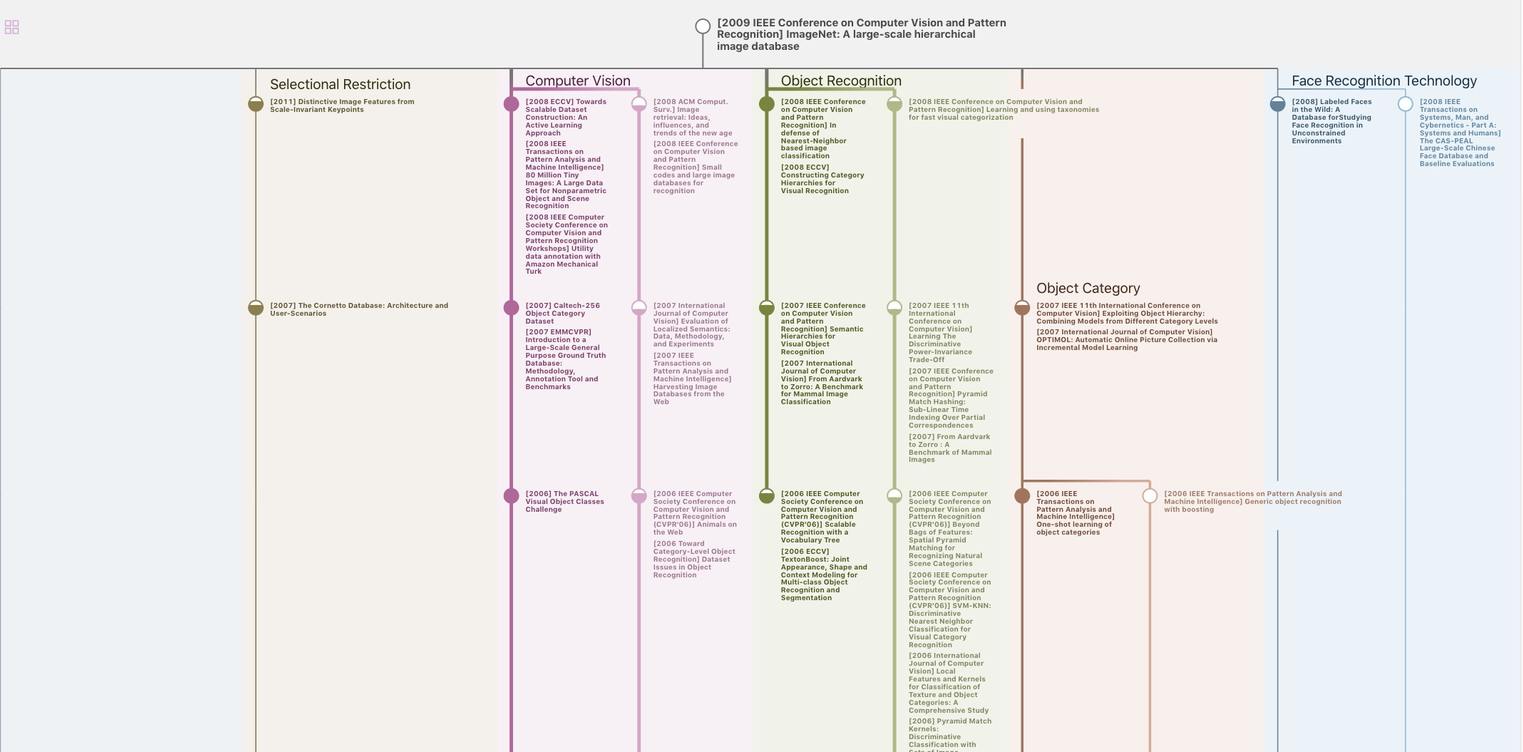
生成溯源树,研究论文发展脉络
Chat Paper
正在生成论文摘要