Deep reinforcement learning using a multi-scale agent with a normalized reward strategy for automatic cephalometric landmark detection
2023 4th International Conference on Big Data Analytics and Practices (IBDAP)(2023)
摘要
Accurate detection of cephalometric landmarks is necessary for precise analysis, diagnosis, and surgical planning. Many studies on automated landmark detection have been conducted including machine learning, convolutional neural network, and reinforcement learning. In this study, deep Q network (DQN) with a normalized reward strategy in multi-scale agent was employed for automated cephalometric landmark detection. The performance of the standard DQN and DQN with a normalized reward strategy were evaluated using the IEEE International Symposium of Biomedical Imaging (ISBI) 2015 Challenge dataset and compared with previously proposed methods. Our data demonstrated that the DQN with a normalized reward strategy using a multi-scale agent achieved the top rank among recently published methods, with an average mean radius error of 1.2 mm and a success detection ratio of 82.34%. These findings indicate that the normalized reward strategy improved the performance of cephalometric landmark detection.
更多查看译文
关键词
cephalometric landmark detection,deep Q network,normalized reward
AI 理解论文
溯源树
样例
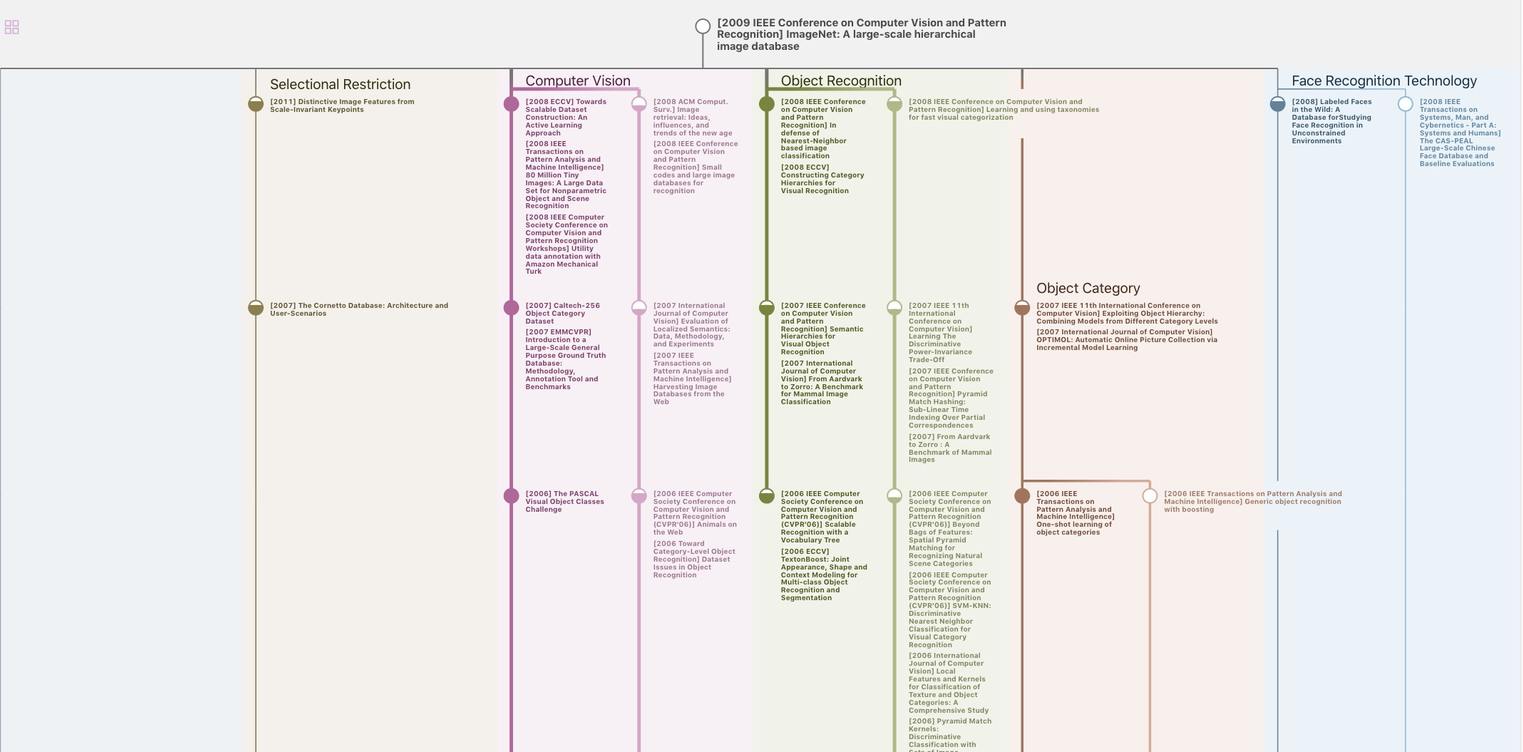
生成溯源树,研究论文发展脉络
Chat Paper
正在生成论文摘要