Multiplexing eMBB and URLLC in Wireless Powered Communication Networks: A Deep Reinforcement Learning-Based Approach
IEEE Wireless Communications Letters(2023)
摘要
This letter investigates the multiplexing of enhanced mobile broadband (eMBB) and ultra-reliable low-latency communications (URLLC) services in a wireless powered communication network, where a hybrid access point coordinates the wireless energy transfer (WET) to users and receives information from them. The preemptive puncturing is adopted to multiplex URLLC traffic onto eMBB transmission. Apart from the energy used for wireless information transmission (WIT), the rest energy in user’s battery is reserved to avoid insufficient energy for future WIT. The problem is formulated to jointly allocate subcarriers, time, and energy to maximize the uplink eMBB sum rate under the constraints of URLLC latency, radio frequency to direct current (RF/DC) sensitivity, user’s battery capacity, and subcarriers availability. We propose a deep reinforcement learning-based approach named mixed deep deterministic policy gradient (Mixed-DDPG), which decomposes the optimization problem into a discrete subproblem for subcarriers allocation and a continuous subproblem for time and energy allocation, and solves them alternately. Numerical results show that the proposed algorithm achieves a higher eMBB sum rate than the existing schemes.
更多查看译文
关键词
eMBB, URLLC, wireless powered communication, preemptive puncturing, RF/DC sensitivity
AI 理解论文
溯源树
样例
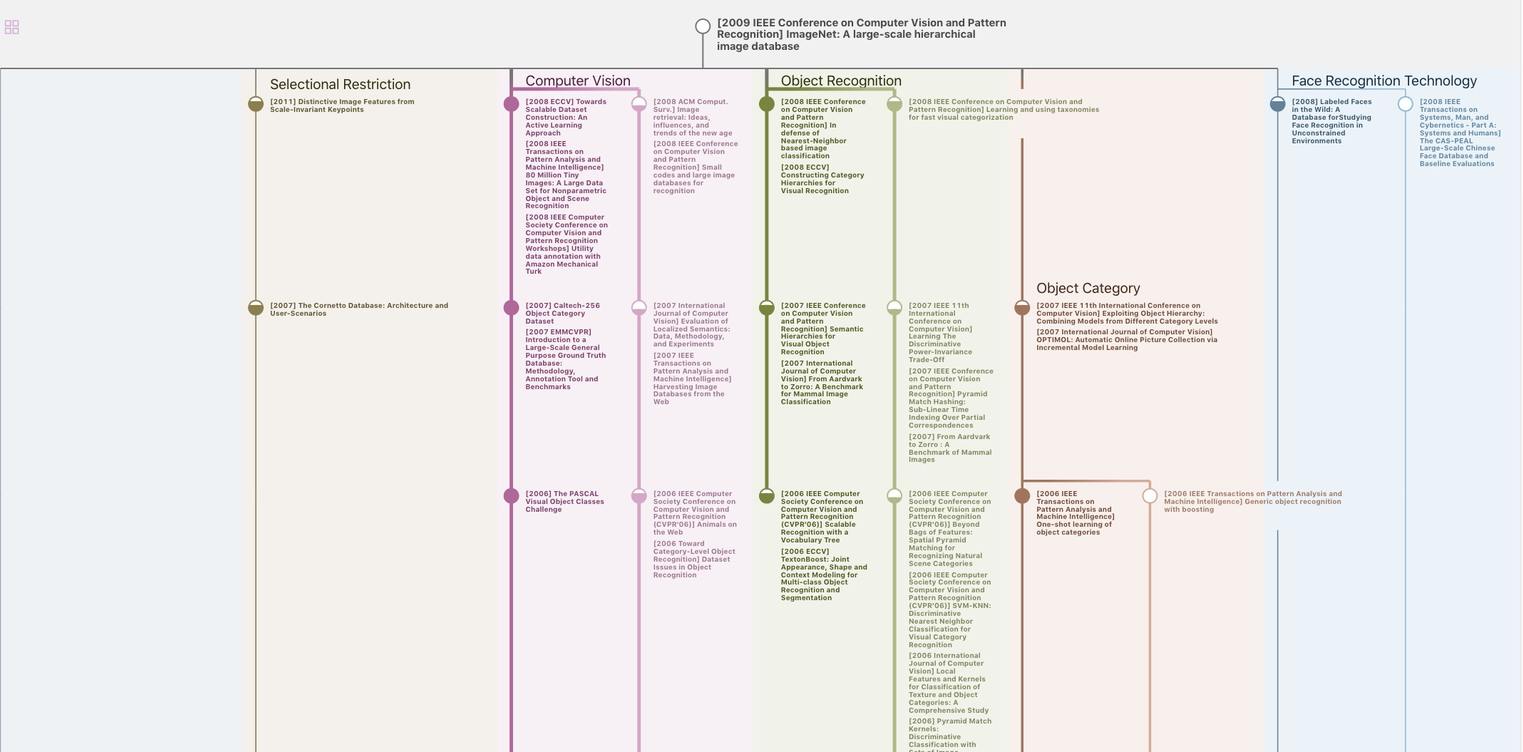
生成溯源树,研究论文发展脉络
Chat Paper
正在生成论文摘要