A generalized fuzzy clustering framework for incomplete data by integrating feature weighted and kernel learning
PeerJ Computer Science(2023)
摘要
Missing data presents a challenge to clustering algorithms, as traditional methods tend to pad incomplete data first before clustering. To combine the two processes of padding and clustering and improve the clustering accuracy, a generalized fuzzy clustering framework is proposed based on optimal completion strategy (OCS) and nearest prototype strategy (NPS) with four improved algorithms developed. Feature weights are introduced to reduce outliers’ influence on the cluster centers, and kernel functions are used to solve the linear indistinguishability problem. The proposed algorithms are evaluated regarding correct clustering rate, iteration number, and external evaluation indexes with nine datasets from the UCI (University of California, Irvine) Machine Learning Repository. The results of the experiment indicate that the clustering accuracy of the feature weighted kernel fuzzy C-means algorithm with NPS (NPS-WKFCM) and feature weighted kernel fuzzy C-means algorithm with OCS (OCS-WKFCM) under varying missing rates is superior to that of seven conventional algorithms. Experiments demonstrate that the enhanced algorithm proposed for clustering incomplete data is superior.
更多查看译文
关键词
Incomplete data, Fuzzy C-Means, Kernel function, Feature weights, OCS, NPS
AI 理解论文
溯源树
样例
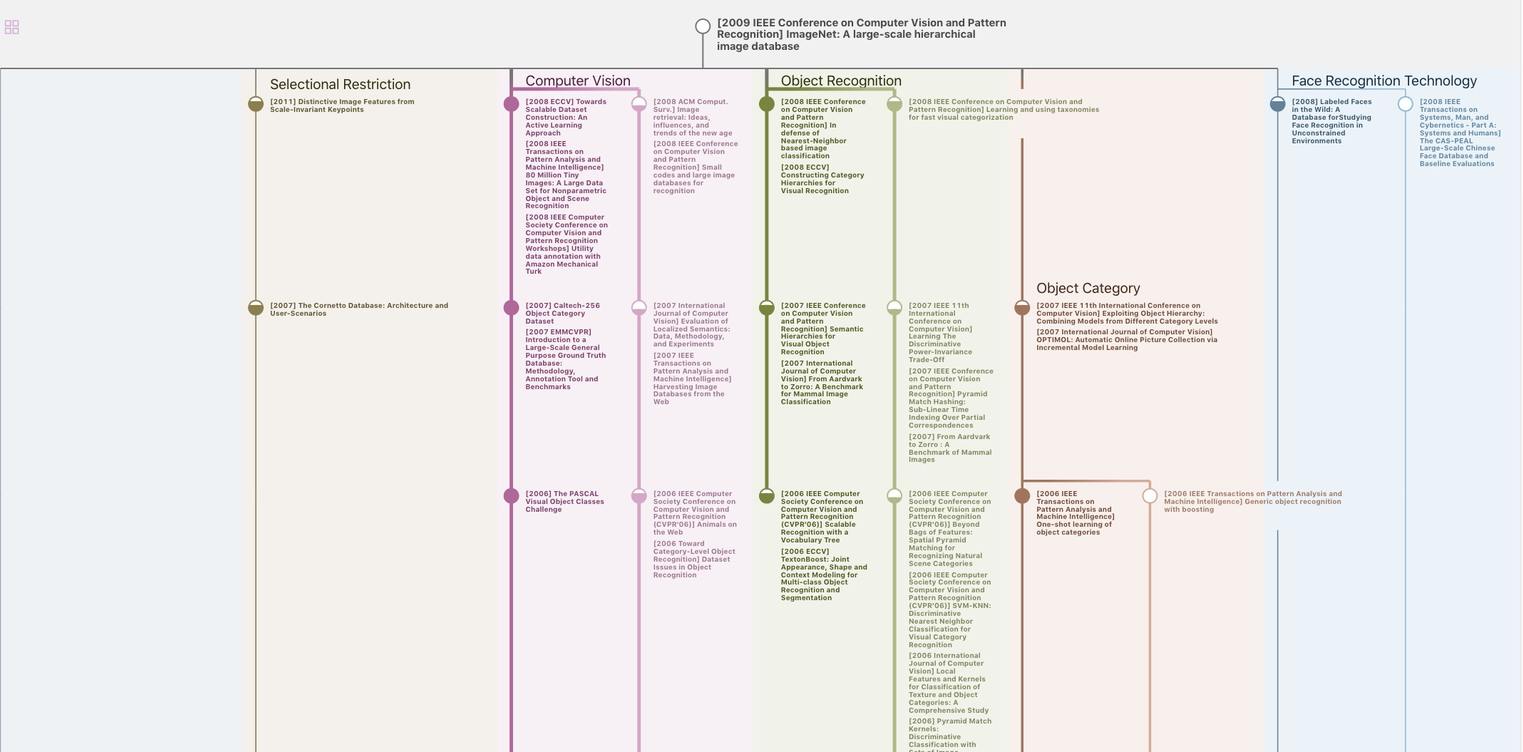
生成溯源树,研究论文发展脉络
Chat Paper
正在生成论文摘要