Federated learning for multi-omics A performance evaluation in Parkinson's disease
Patterns(2024)
摘要
While machine learning (ML) research has recently grown more in popularity, its application in the omics domain is constrained by access to sufficiently large, high -quality datasets needed to train ML models. Federated learning (FL) represents an opportunity to enable collaborative curation of such datasets among participating institutions. We compare the simulated performance of several models trained using FL against classically trained ML models on the task of multi-omics Parkinson's disease prediction. We find that FL model performance tracks centrally trained ML models, where the most performant FL model achieves an AUC-PR of 0.876 +/- 0.009, 0.014 +/- 0.003 less than its centrally trained variation. We also determine that the dispersion of samples within a federation plays a meaningful role in model performance. Our study implements several open -source FL frameworks and aims to highlight some of the challenges and opportunities when applying these collaborative methods in multi-omics studies.
更多查看译文
AI 理解论文
溯源树
样例
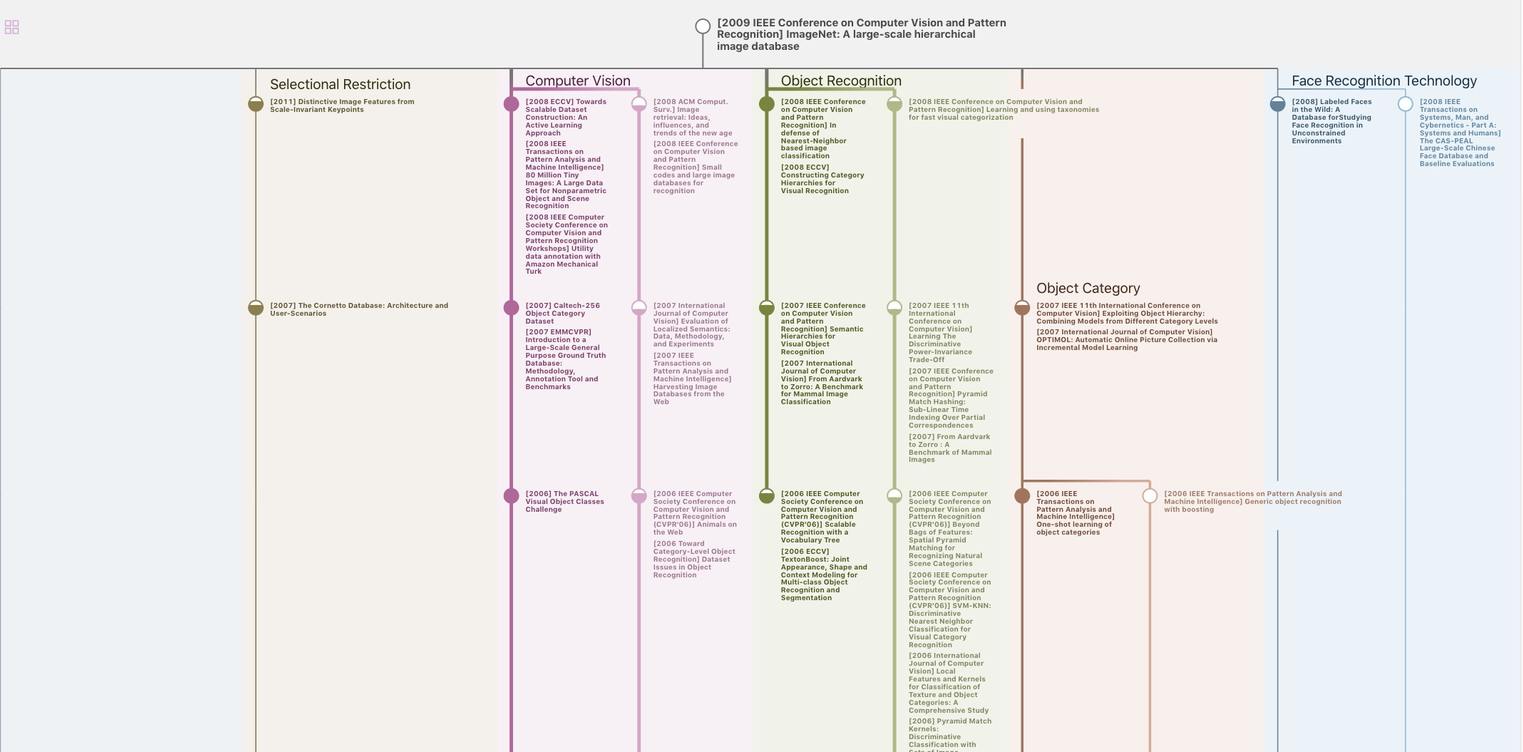
生成溯源树,研究论文发展脉络
Chat Paper
正在生成论文摘要