CellPLM: Pre-training of Cell Language Model Beyond Single Cells
bioRxiv (Cold Spring Harbor Laboratory)(2023)
摘要
The current state-of-the-art single-cell pre-trained models are greatly inspired by the success of large language models. They trained transformers by treating genes as tokens and cells as sentences. However, three fundamental differences between single-cell data and natural language data are overlooked: (1) scRNA-seq data are presented as bag-of-genes instead of sequences of RNAs; (2) Cell-cell relations are more intricate and important than inter-sentence relations; and (3) The quantity of single-cell data is considerably inferior to text data, and they are very noisy. In light of these characteristics, we propose a new pre-trained model CellPLM, which takes cells as tokens and tissues as sentences. In addition, we leverage spatially-resolved transcriptomic data in pre-training to facilitate learning cell-cell relationships and introduce a Gaussian mixture prior distribution as an additional inductive bias to overcome data limitation. CellPLM is the first single-cell pre-trained transformer that encodes cell-cell relations and it consistently outperforms existing pre-trained and non-pre-trained models in diverse downstream tasks, with 100x times higher inference speed compared to existing pre-trained models.
### Competing Interest Statement
The authors have declared no competing interest.
更多查看译文
关键词
cellplm language model,cells,pre-training
AI 理解论文
溯源树
样例
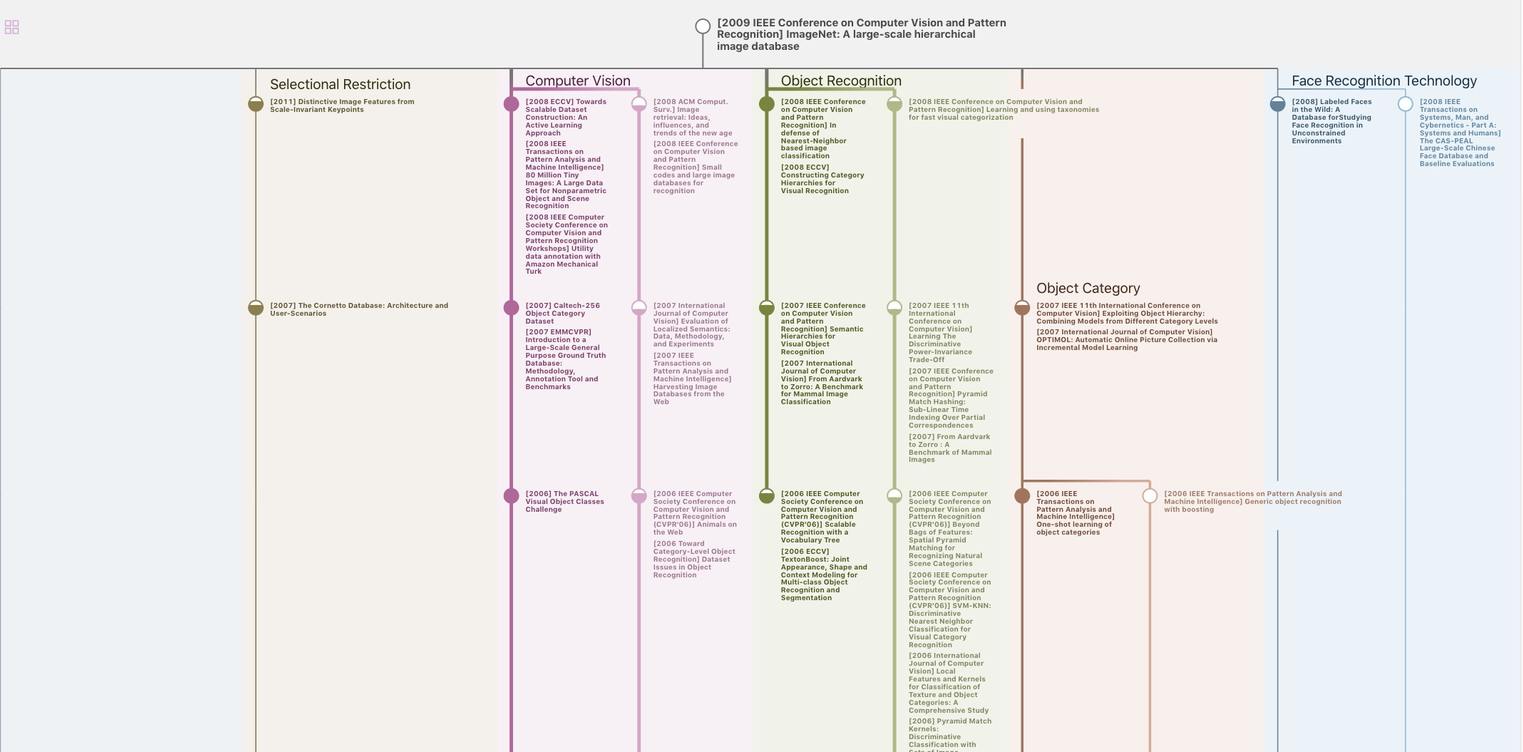
生成溯源树,研究论文发展脉络
Chat Paper
正在生成论文摘要