Contribution of image statistics and semantics in local vs. distributed EEG decoding of rapid serial visual presentation
bioRxiv (Cold Spring Harbor Laboratory)(2023)
摘要
Spatio-temporal patterns of evoked brain activity contain information that can be used to decode and categorize the semantic content of visual stimuli. This procedure can be biased by statistical regularities which can be independent from the concepts that are represented in the stimuli, prompting the need to dissociate between the contributions of image statistics and semantics to decoding accuracy. We trained machine learning models to distinguish between concepts included in the THINGS-EEG dataset using electroencephalography (EEG) data acquired during a rapid serial visual presentation protocol. After systematic univariate feature selection in the temporal and spatial domains, we constructed simple models based on local signals which superseded the accuracy of more complex classifiers based on distributed patterns of information. Simpler models were characterized by their sensitivity to biases in the statistics of visual stimuli, with some of them preserving their accuracy after random replacement of the training dataset while maintaining the overall statistics of the images. We conclude that model complexity impacts on the sensitivity to confounding factors regardless of performance; therefore, the choice of EEG features for semantic decoding should ideally be informed by the underlying neurobiological mechanisms.
### Competing Interest Statement
The authors have declared no competing interest.
更多查看译文
关键词
eeg,image statistics
AI 理解论文
溯源树
样例
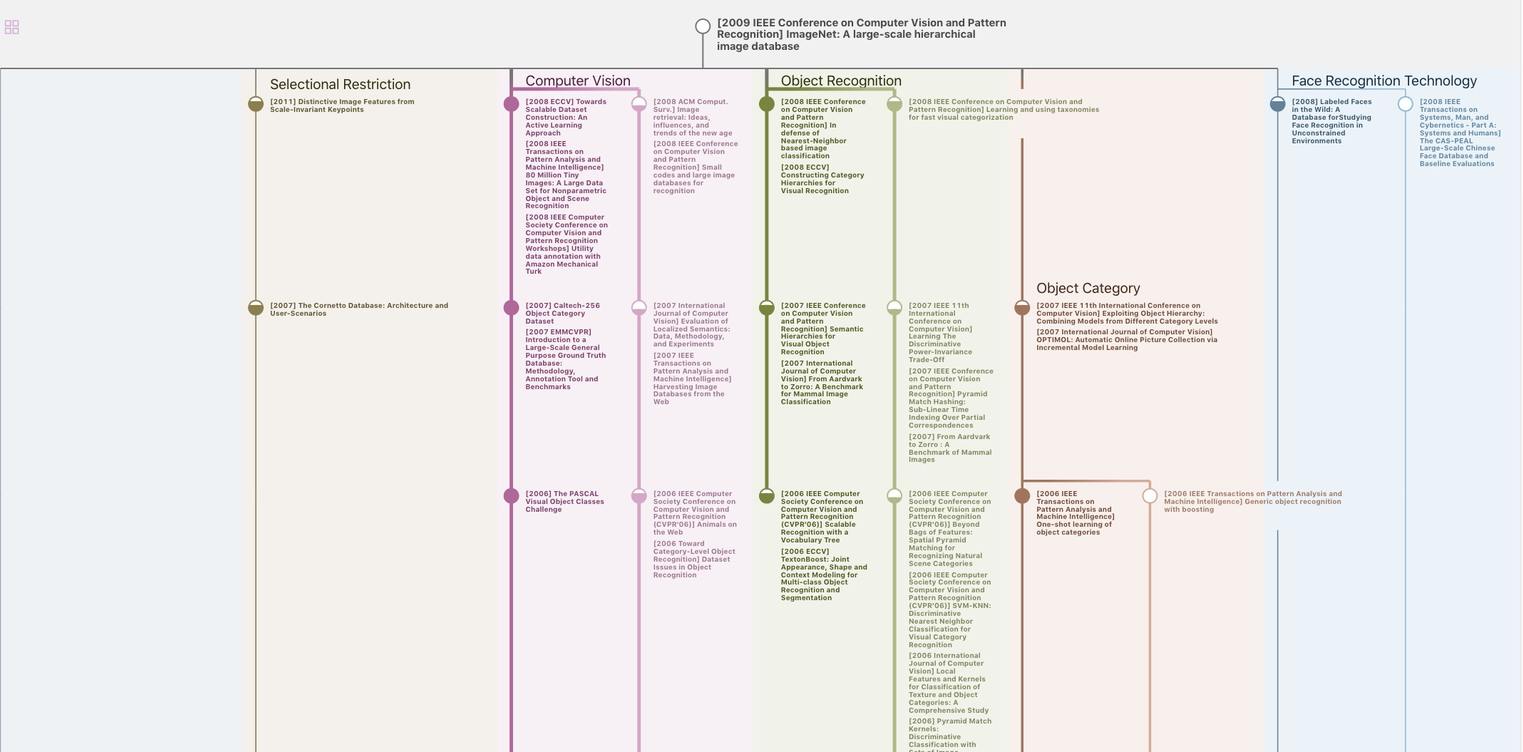
生成溯源树,研究论文发展脉络
Chat Paper
正在生成论文摘要