An Lstm&Topic-Cnn Model For Classification Of Online Chinese Medical Questions
IEEE ACCESS(2021)
摘要
In recent years, people's interest in health question and answer (Q&A) websites has been growing with the development of the internet technologies. How to seek appropriate professional medical information among the massive data has become the focus of all patients. Therefore, it is vital to obtain reasonable predictions and automatic recommendations on the basis of patients' keyword descriptions of their health status and question intention. The key to solving this problem is to achieve automatic text classification of health questions. This paper considered a feature fusion model for the classification of Chinese short texts on medical health Q&A websites by combining the text features and topic features. Firstly, we generated the text word vector by word embedding method and obtained the text features under Long Short-Term Memory (LSTM) model. Given the difficulty in determination of topic numbers, we conducted a sub-sample experiment to obtain the few optimal topic numbers under which the classification performances were good. Then we extracted the topic features and used the one-dimensional convolution idea of the Convolutional Neural Network (CNN) model for topic feature filtering. Finally, we combined the two features together subtly for text classification. Two experiments were conducted to illustrate our model in terms of recall rate, precision, and F1 value when the datasets were from different online medical Q&A websites. Results showed that the LSTM&Topic-CNN model could efficiently enhance the classification effect of Chinese medical health question texts.
更多查看译文
关键词
Feature extraction, Semantics, Data models, Analytical models, Training, Recurrent neural networks, Predictive models, Chinese medical Q&, A text, long short-term memory, latent dirichlet allocation, convolutional neural network, feature fusion
AI 理解论文
溯源树
样例
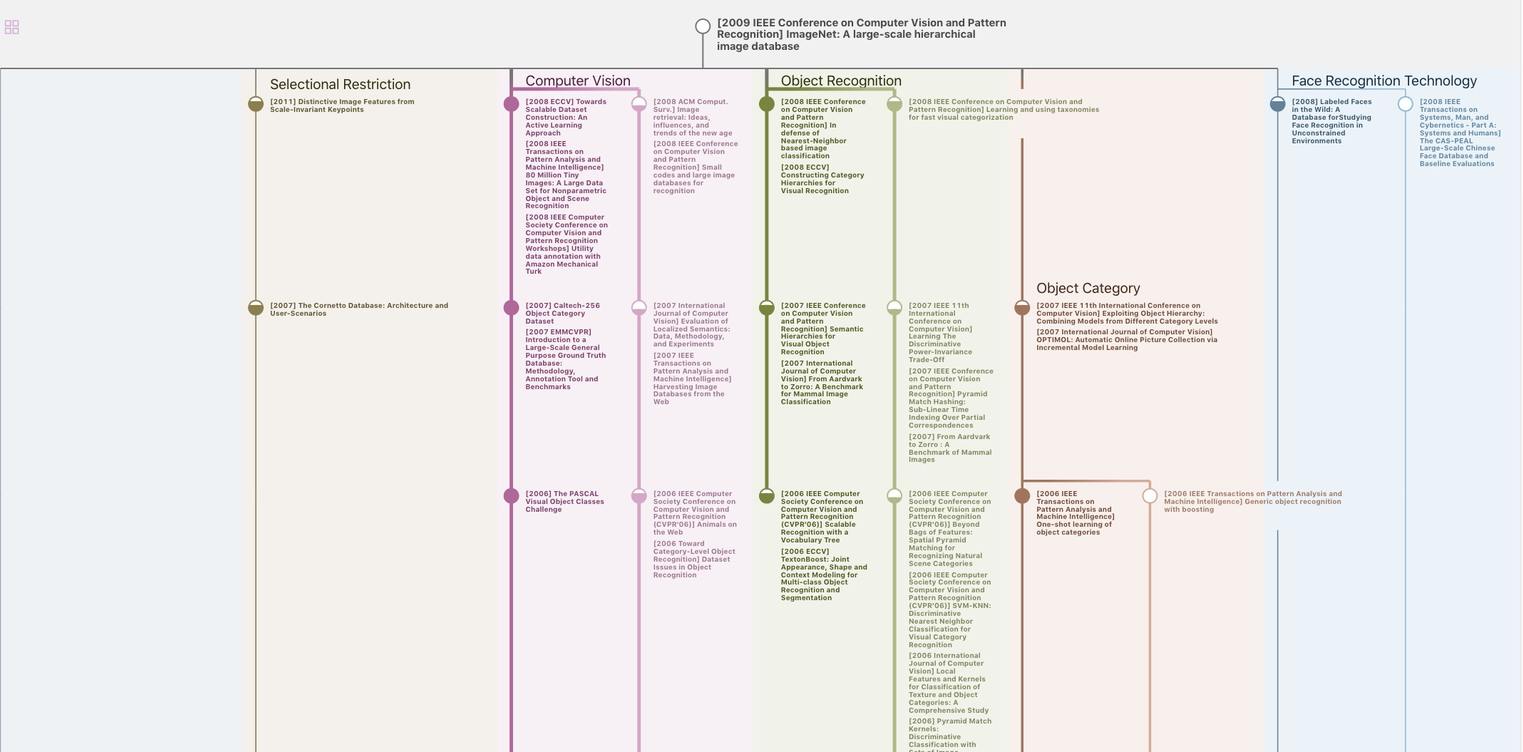
生成溯源树,研究论文发展脉络
Chat Paper
正在生成论文摘要