MixOmics Integration of Biological Datasets Identifies Highly Correlated Key Variables of COVID-19 severity
bioRxiv (Cold Spring Harbor Laboratory)(2023)
摘要
Background Despite several years since the COVID-19 pandemic was declared, challenges remain in understanding the factors that can predict the severity of COVID-19 disease and complications of SARS-CoV-2 infection. While many large-scale Multiomic datasets have been published, integration of these datasets has the potential to substantially increase the biological insight gained allowing a more complex comprehension of the disease pathogenesis. Such insight may improve our ability to predict disease progression, detect severe cases more rapidly and develop effective therapeutics.
Methods In this study we have applied an innovative machine learning algorithm to delineate COVID-severity based on integration of paired samples of proteomic and transcriptomic data from a small cohort of patients testing positive for SARS-CoV-2 infection with differential disease severity. Targeted plasma proteomics and an onco-immune targeted transcriptomic panel was performed on sequential samples from a cohort of 23 severe, 21 moderate and 10 mild COVID-19 patients. We applied DIABLO, a new integrative method, to identify multi- omics biomarker panels that can discriminate between multiple phenotypic groups, such as the varied severity of disease in COVID-19 patients.
Results As COVID-19 severity is known among our sample group, we can train models using this as the outcome variable and calculate features that are important predictors of severe disease. In this study, we detect highly correlated key variables of severe COVID-19 using transcriptomic discriminant analysis and multi-omics integration methods.
Conclusions This approach highlights the power of data integration from a small cohort of patients offering a better biological understanding of the molecular mechanisms driving COVID-19 severity and an opportunity to improve prediction of disease trajectories and targeted therapeutics.
### Competing Interest Statement
The authors have declared no competing interest.
更多查看译文
关键词
biological datasets identifies
AI 理解论文
溯源树
样例
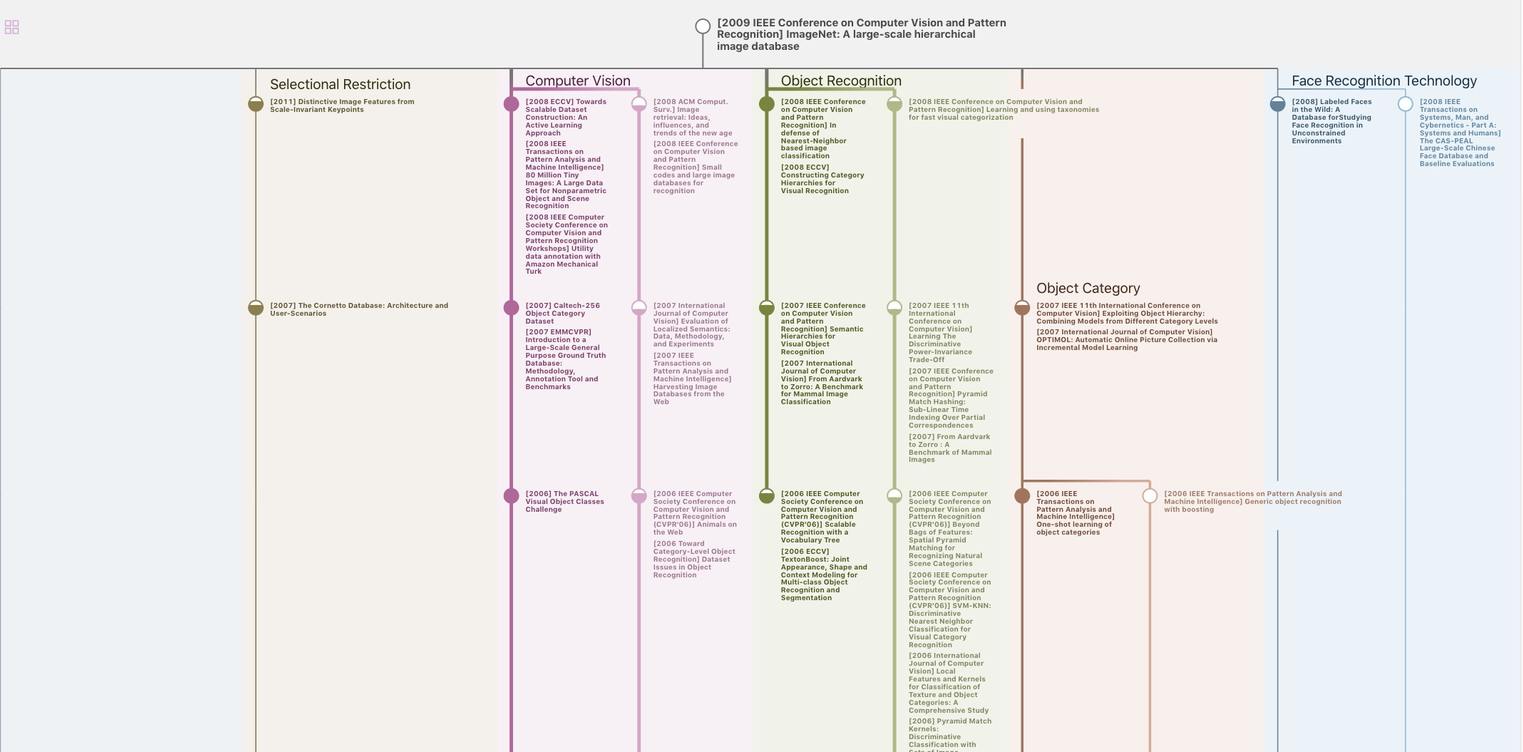
生成溯源树,研究论文发展脉络
Chat Paper
正在生成论文摘要