Ensembling Unets, sparse representation and low dimensional visualization for rare chromosomal aberration detection in light microscopy images
bioRxiv (Cold Spring Harbor Laboratory)(2023)
摘要
In biological dosimetry, a radiation dose is estimated using the average number of chromosomal aberration per peripheral blood lymphocytes. To achieve an adequate precision in the estimation of this average, hundreds of cells must be analyzed in 2D microscopy images. Currently, this analysis is performed manually, as conventional computer vision techniques struggle with the wide variety of shapes showcased by chromosomes. The false discovery rate of current automated detection systems is high and variable, depending on small variations in data quality (chromosome spread, illumination variations …), which makes using it in a fully automated fashion impossible. Automating chromosomal aberration is needed to reduce diagnosis time. Furthermore, an automated system can process more images, which improves confidence intervals around the estimated radiation dose. We build an object detection model to automate chromosomal aberration detection using recent advances in deep convolutional neural networks and statistical learning. We formulated the problem of rare aberration detection as a heatmap regression problem requiring the minimization of a sparsity-promoting loss to reduce the false alarm rate. Our Unet-based approach is analoguous to a one-stage object detector, and keeps the number of hyperparameters to a minimum. Finally, we demonstrate large performance improvements using an ensemble of checkpoints collected during a single run of training. A PCA-based strategy is used to provide cues for interpretation of our deep neural network-based model. The methodology is demonstrated on real, large, and challenging datasets depicting rare chromosomal aberrations and is favorably compared to a reference dosimetry technique.
### Competing Interest Statement
The authors have declared no competing interest.
更多查看译文
关键词
rare chromosomal aberration detection,light microscopy images,light microscopy,sparse representation
AI 理解论文
溯源树
样例
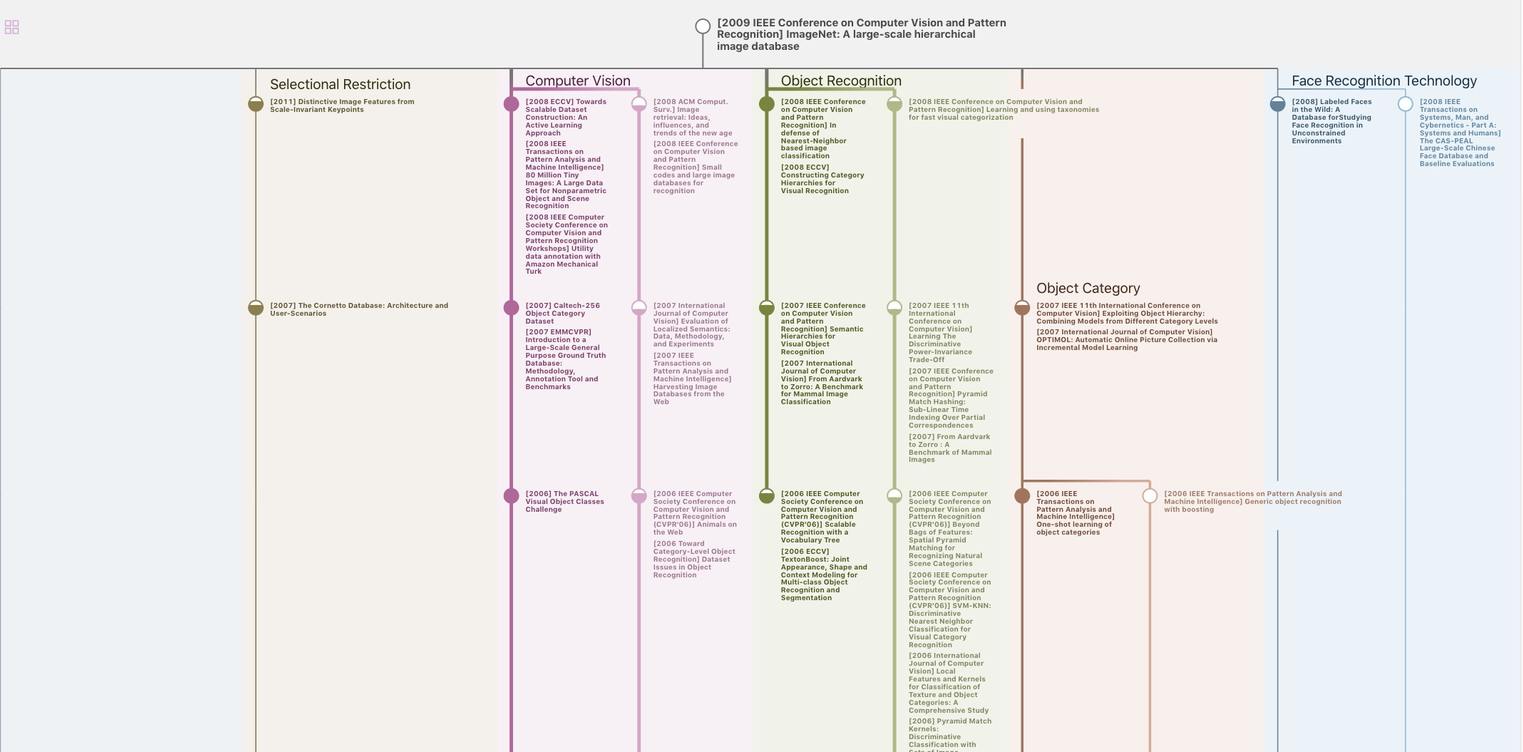
生成溯源树,研究论文发展脉络
Chat Paper
正在生成论文摘要