Radiomics and artificial intelligence analysis by T2-weighted imaging and dynamic contrast-enhanced magnetic resonance imaging to predict Breast Cancer Histological Outcome
La radiologia medica(2023)
摘要
Objective The objective of the study was to evaluate the accuracy of radiomics features obtained by MR images to predict Breast Cancer Histological Outcome. Methods A total of 217 patients with malignant lesions were analysed underwent MRI examinations. Considering histological findings as the ground truth, four different types of findings were used in both univariate and multivariate analyses: (1) G1 + G2 vs G3 classification; (2) presence of human epidermal growth factor receptor 2 (HER2 + vs HER2 −); (3) presence of the hormone receptor (HR + vs HR −); and (4) presence of luminal subtypes of breast cancer. Results The best accuracy for discriminating HER2 + versus HER2 − breast cancers was obtained considering nine predictors by early phase T1-weighted subtraction images and a decision tree (accuracy of 88% on validation set). The best accuracy for discriminating HR + versus HR − breast cancers was obtained considering nine predictors by T2-weighted subtraction images and a decision tree (accuracy of 90% on validation set). The best accuracy for discriminating G1 + G2 versus G3 breast cancers was obtained considering 16 predictors by early phase T1-weighted subtraction images in a linear regression model with an accuracy of 75%. The best accuracy for discriminating luminal versus non-luminal breast cancers was obtained considering 27 predictors by early phase T1-weighted subtraction images and a decision tree (accuracy of 94% on validation set). Conclusions The combination of radiomics analysis and artificial intelligence techniques could be used to support physician decision-making in prediction of Breast Cancer Histological Outcome.
更多查看译文
关键词
Radiomics,Artificial intelligence,Magnetic resonance imaging,Breast cancer
AI 理解论文
溯源树
样例
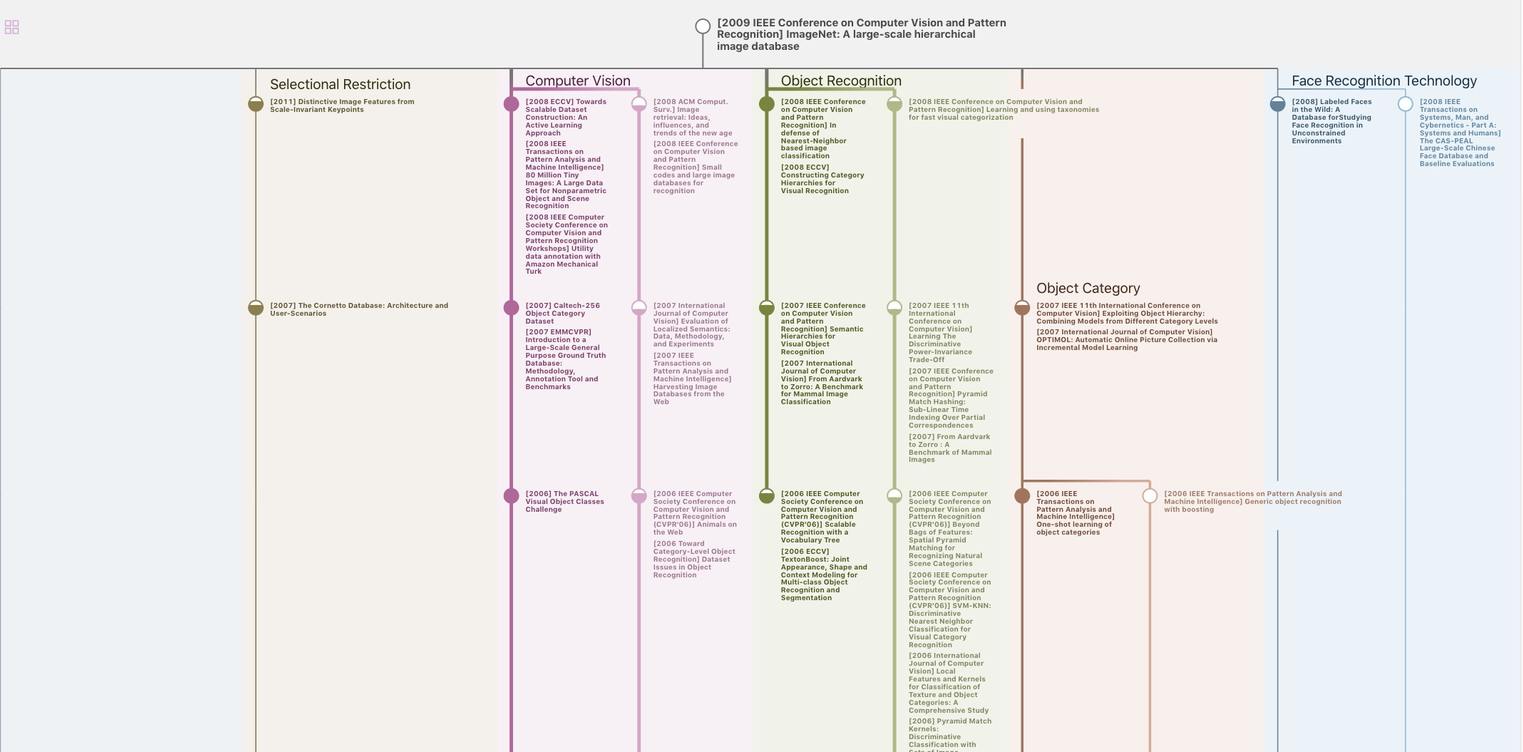
生成溯源树,研究论文发展脉络
Chat Paper
正在生成论文摘要