Accurate identification of cashmere and wool fibers based on enhanced ShuffleNetV2 and transfer learning
Journal of Big Data(2023)
摘要
Recognizing cashmere and wool fibers has been a challenging problem in the textile industry due to their similar morphological structure, chemical composition, and physicochemical properties. Traditional manual methods for identifying these fibers are inefficient, labor-intensive, and inaccurate. To address these issues, we present a novel method for recognizing cashmere and wool fibers using an improved version of ShuffleNetV2 and Transfer Learning, which we implement as a new cashmere and wool classification network (CWCNet).The approach leverages depthwise separable dilated convolution to extract more feature information for fiber classification. We also introduce a new activation function that enhances the nonlinear representation of the model and allows it to more fully extract negative feature information. Experimental results demonstrate that CWCNet achieves an accuracy rate of up to 98.438% on our self-built dataset, which is a 2.084% improvement over the original ShuffleNetV2 model. Furthermore, our proposed method outperforms classical models such as EfficientNetB0, MobileNetV2, Wide-ResNet50, and ShuffleNetV2 in terms of recognition accuracy while remaining lightweight.The method is capable of extracting more information on fiber characteristics and has the potential to replace manual labor as technological advancements continue to be made. This will greatly benefit engineering applications in the textile industry by providing more efficient and accurate fiber classification.
更多查看译文
关键词
Cashmere and wool,Transfer learning,Activation function,Data augmentation,Classification
AI 理解论文
溯源树
样例
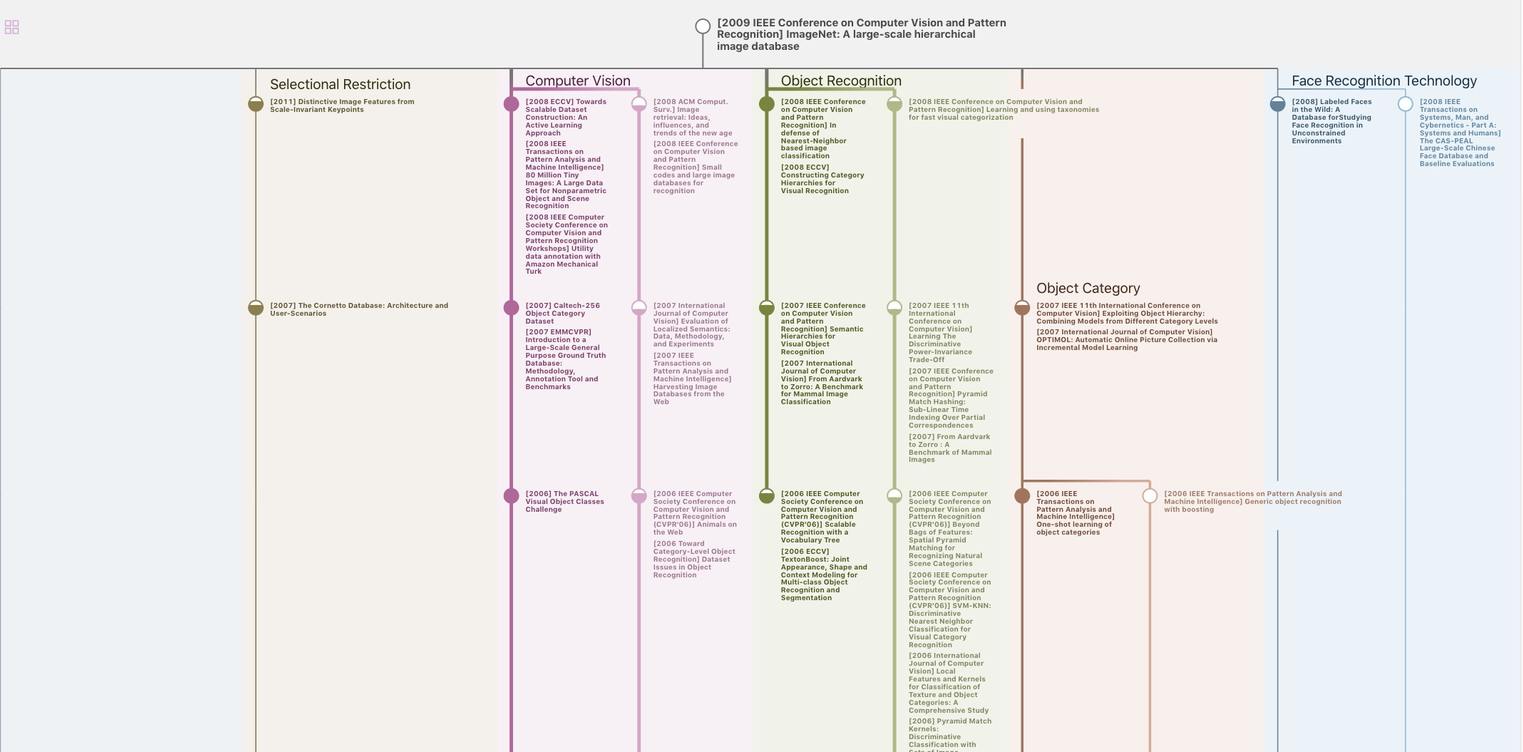
生成溯源树,研究论文发展脉络
Chat Paper
正在生成论文摘要