CrossFormer: Multi‐scale cross‐attention for polyp segmentation
IET Image Processing(2023)
摘要
Abstract Colonoscopy is a common method for the early detection of colorectal cancer (CRC). The segmentation of colonoscopy imagery is valuable for examining the lesion. However, as colonic polyps have various sizes and shapes, and their morphological characteristics are similar to those of mucosa, it is difficult to segment them accurately. To address this, a novel neural network architecture called CrossFormer is proposed. CrossFormer combines cross‐attention and multi‐scale methods, which can achieve high‐precision automatic segmentation of the polyps. A multi‐scale cross‐attention module is proposed to enhance the ability to extract context information and learn different features. In addition, a novel channel enhancement module is used to focus on the useful channel information. The model is trained and tested on the Kvasir and CVC‐ClinicDB datasets. Experimental results show that the proposed model outperforms most existing polyps segmentation methods.
更多查看译文
关键词
channel enhancement,colorectal cancer,cross‐attention,multi scale,polyp segmentation
AI 理解论文
溯源树
样例
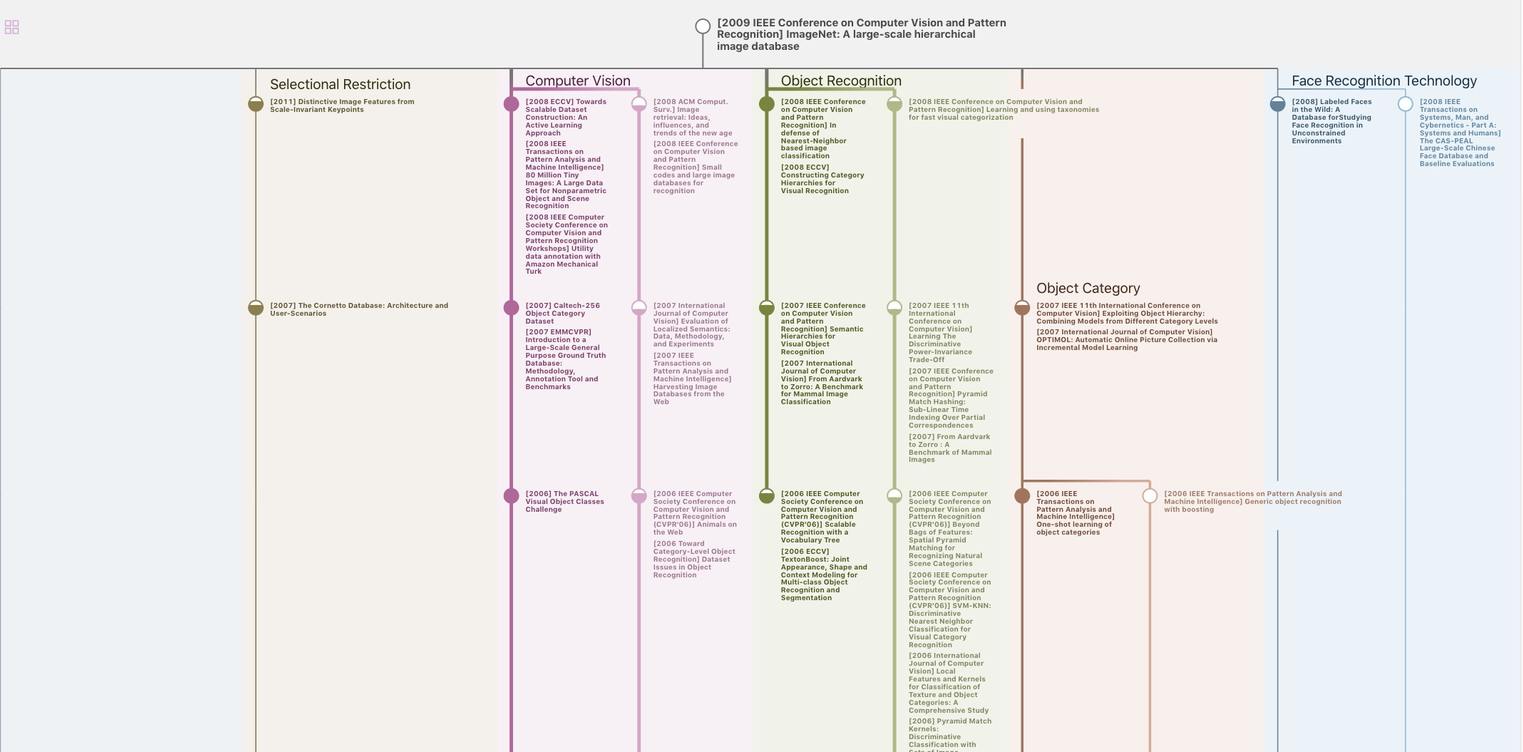
生成溯源树,研究论文发展脉络
Chat Paper
正在生成论文摘要