Overview of data preprocessing for machine learning applications in human microbiome research
Frontiers in Microbiology(2023)
Abstract
Although metagenomic sequencing is now the preferred technique to study microbiome-host interactions, analyzing and interpreting microbiome sequencing data presents challenges primarily attributed to the statistical specificities of the data (e.g., sparse, over-dispersed, compositional, inter-variable dependency). This mini review explores preprocessing and transformation methods applied in recent human microbiome studies to address microbiome data analysis challenges. Our results indicate a limited adoption of transformation methods targeting the statistical characteristics of microbiome sequencing data. Instead, there is a prevalent usage of relative and normalization-based transformations that do not specifically account for the specific attributes of microbiome data. The information on preprocessing and transformations applied to the data before analysis was incomplete or missing in many publications, leading to reproducibility concerns, comparability issues, and questionable results. We hope this mini review will provide researchers and newcomers to the field of human microbiome research with an up-to-date point of reference for various data transformation tools and assist them in choosing the most suitable transformation method based on their research questions, objectives, and data characteristics.
MoreTranslated text
Key words
human microbiome, data preprocessing, machine learning, compositionality, normalization, metagenomics data
AI Read Science
Must-Reading Tree
Example
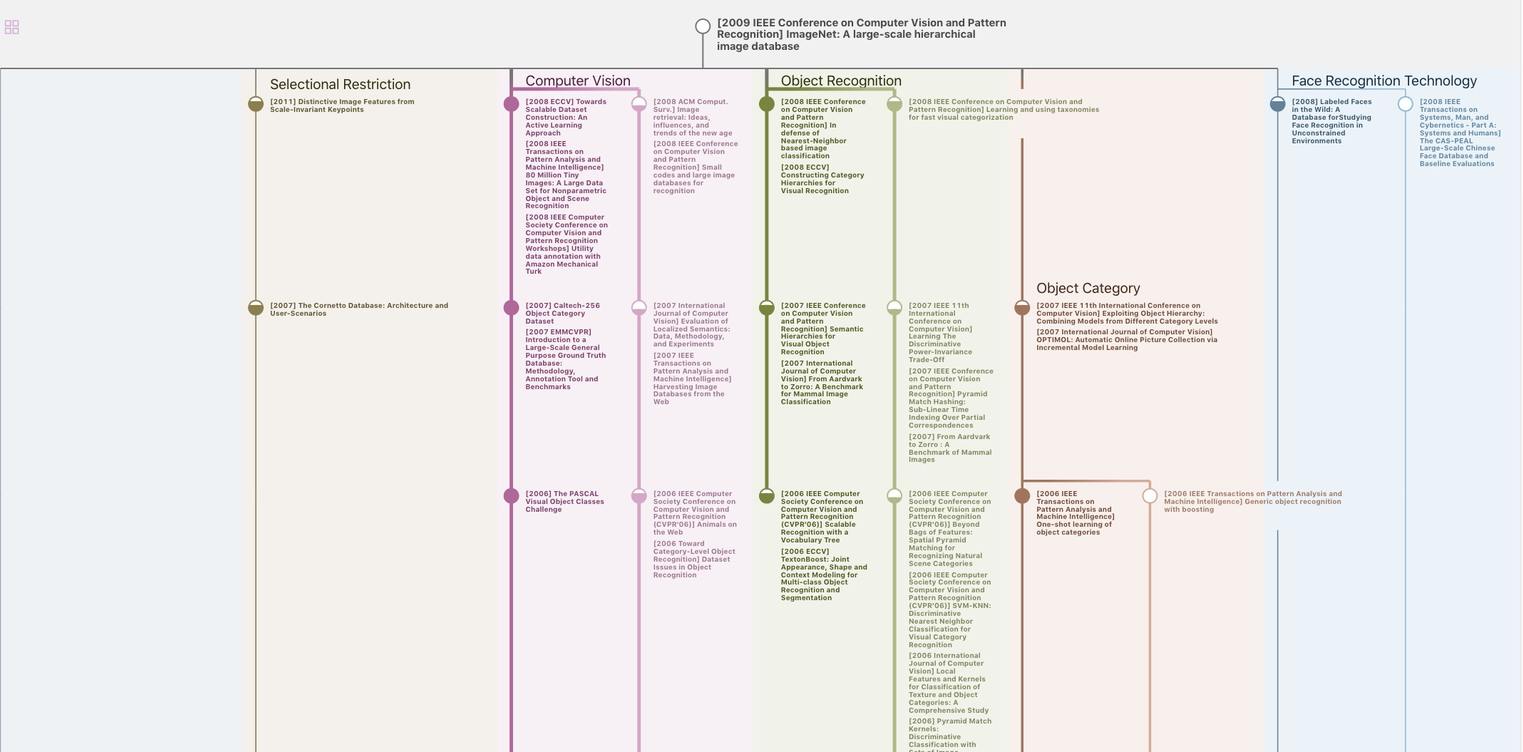
Generate MRT to find the research sequence of this paper
Chat Paper
Summary is being generated by the instructions you defined