Evaluating Self-Supervised Speech Representations for Indigenous American Languages
arXiv (Cornell University)(2023)
摘要
The application of self-supervision to speech representation learning has garnered significant interest in recent years, due to its scalability to large amounts of unlabeled data. However, much progress, both in terms of pre-training and downstream evaluation, has remained concentrated in monolingual models that only consider English. Few models consider other languages, and even fewer consider indigenous ones. In our submission to the New Language Track of the ASRU 2023 ML-SUPERB Challenge, we present an ASR corpus for Quechua, an indigenous South American Language. We benchmark the efficacy of large SSL models on Quechua, along with 6 other indigenous languages such as Guarani and Bribri, on low-resource ASR. Our results show surprisingly strong performance by state-of-the-art SSL models, showing the potential generalizability of large-scale models to real-world data.
更多查看译文
关键词
speech representations,american languages,self-supervised
AI 理解论文
溯源树
样例
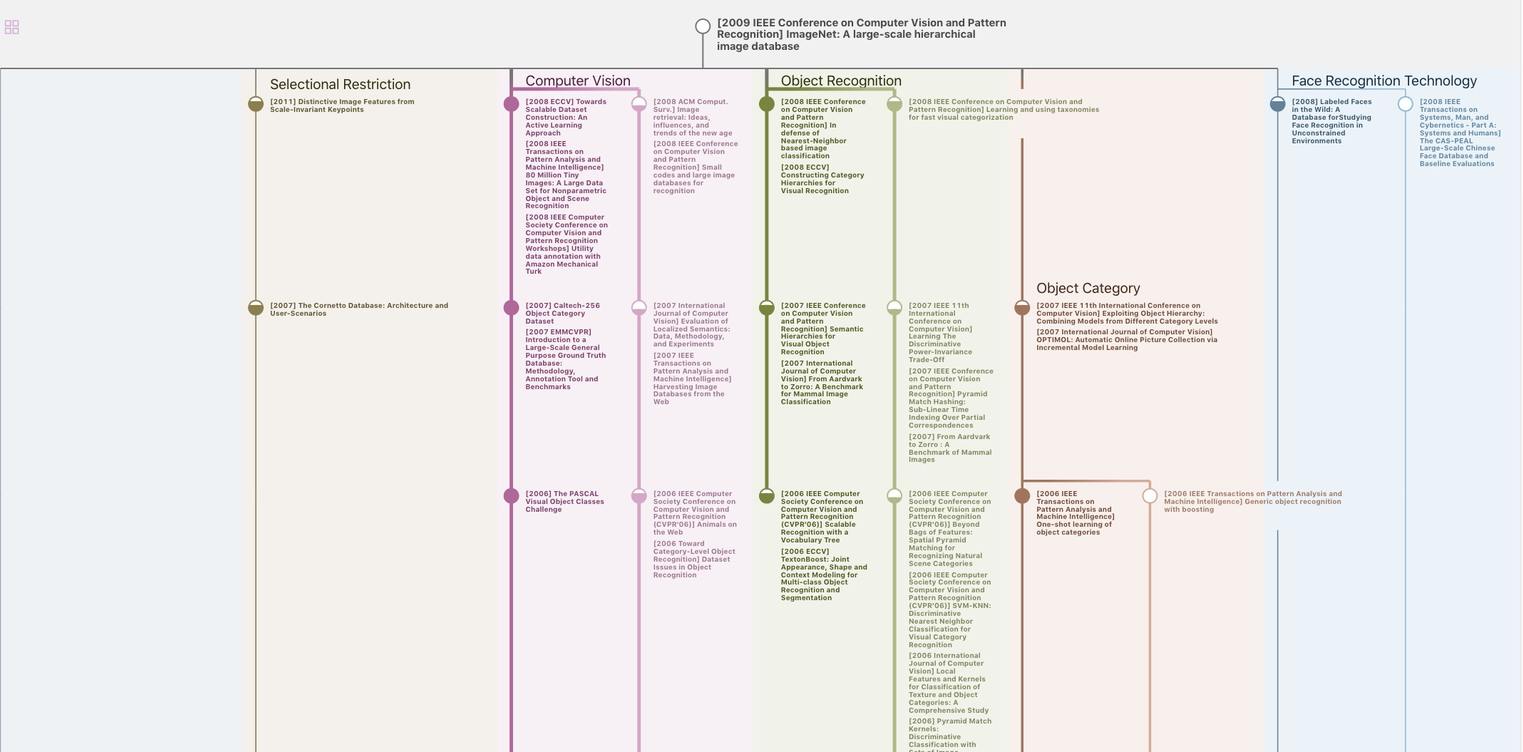
生成溯源树,研究论文发展脉络
Chat Paper
正在生成论文摘要