Plug-and-Play Posterior Sampling under Mismatched Measurement and Prior Models
ICLR 2024(2023)
摘要
Posterior sampling has been shown to be a powerful Bayesian approach for solving imaging inverse problems. The recent plug-and-play unadjusted Langevin algorithm (PnP-ULA) has emerged as a promising method for Monte Carlo sampling and minimum mean squared error (MMSE) estimation by combining physical measurement models with deep-learning priors specified using image denoisers. However, the intricate relationship between the sampling distribution of PnP-ULA and the mismatched data-fidelity and denoiser has not been theoretically analyzed. We address this gap by proposing a posterior-L2 pseudometric and using it to quantify an explicit error bound for PnP-ULA under mismatched posterior distribution. We numerically validate our theory on several inverse problems such as sampling from Gaussian mixture models and image deblurring. Our results suggest that the sensitivity of the sampling distribution of PnP-ULA to a mismatch in the measurement model and the denoiser can be precisely characterized.
更多查看译文
关键词
Inverse problems,plug-and-play priors,posterior sampling,unadjusted Langevin algorithm
AI 理解论文
溯源树
样例
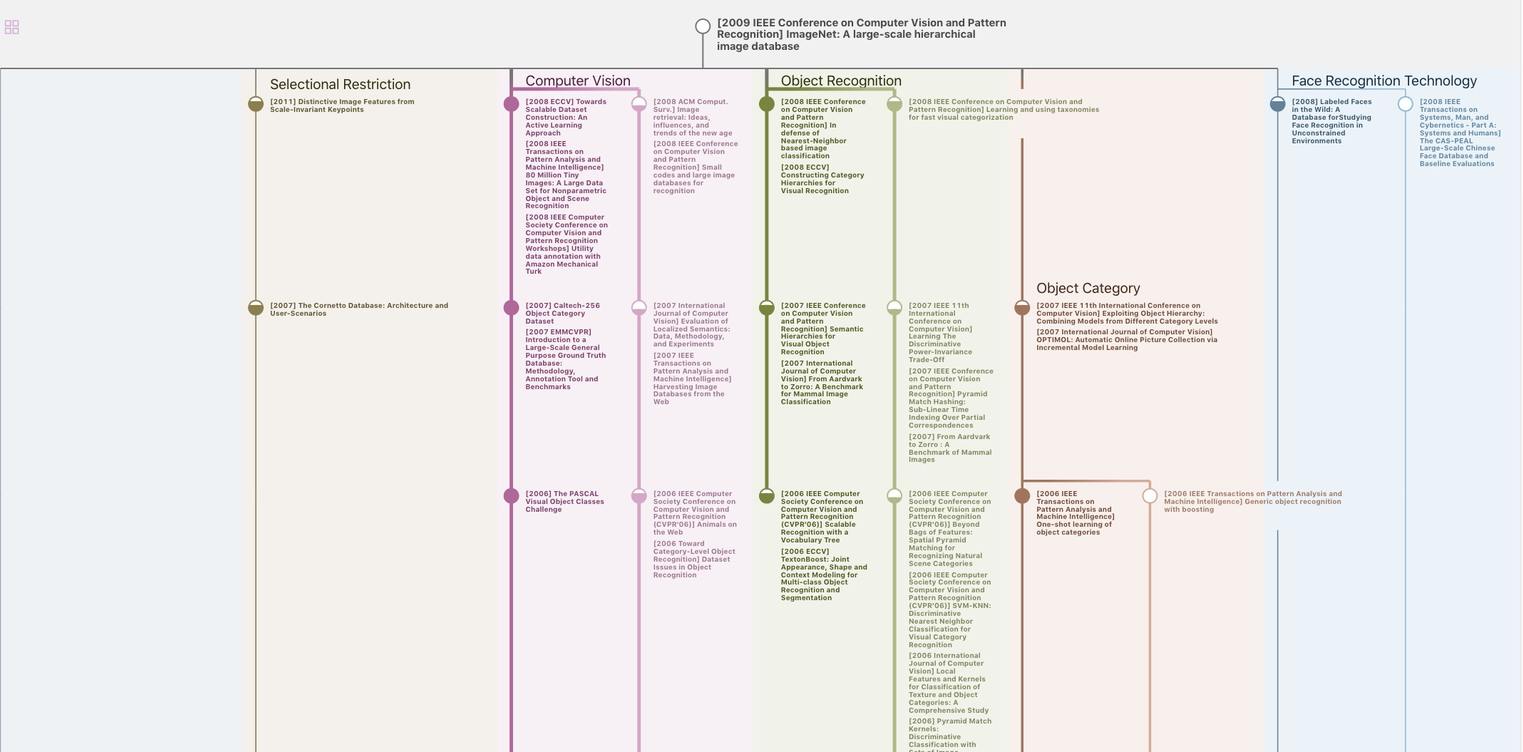
生成溯源树,研究论文发展脉络
Chat Paper
正在生成论文摘要