SimVLG: Simple and Efficient Pretraining of Visual Language Generative Models
CoRR(2023)
Abstract
In this paper, we propose ``SimVLG'', a streamlined framework for the pre-training of computationally intensive vision-language generative models, leveraging frozen pre-trained large language models (LLMs). The prevailing paradigm in vision-language pre-training (VLP) typically involves a two-stage optimization process: an initial resource-intensive phase dedicated to general-purpose vision-language representation learning, aimed at extracting and consolidating pertinent visual features, followed by a subsequent phase focusing on end-to-end alignment between visual and linguistic modalities. Our one-stage, single-loss framework circumvents the aforementioned computationally demanding first stage of training by gradually merging similar visual tokens during training. This gradual merging process effectively compacts the visual information while preserving the richness of semantic content, leading to fast convergence without sacrificing performance. Our experiments show that our approach can speed up the training of vision-language models by a factor $\times 5$ without noticeable impact on the overall performance. Additionally, we show that our models can achieve comparable performance to current vision-language models with only $1/10$ of the data. Finally, we demonstrate how our image-text models can be easily adapted to video-language generative tasks through a novel soft attentive temporal token merging modules.
MoreTranslated text
Key words
visual language generative
AI Read Science
Must-Reading Tree
Example
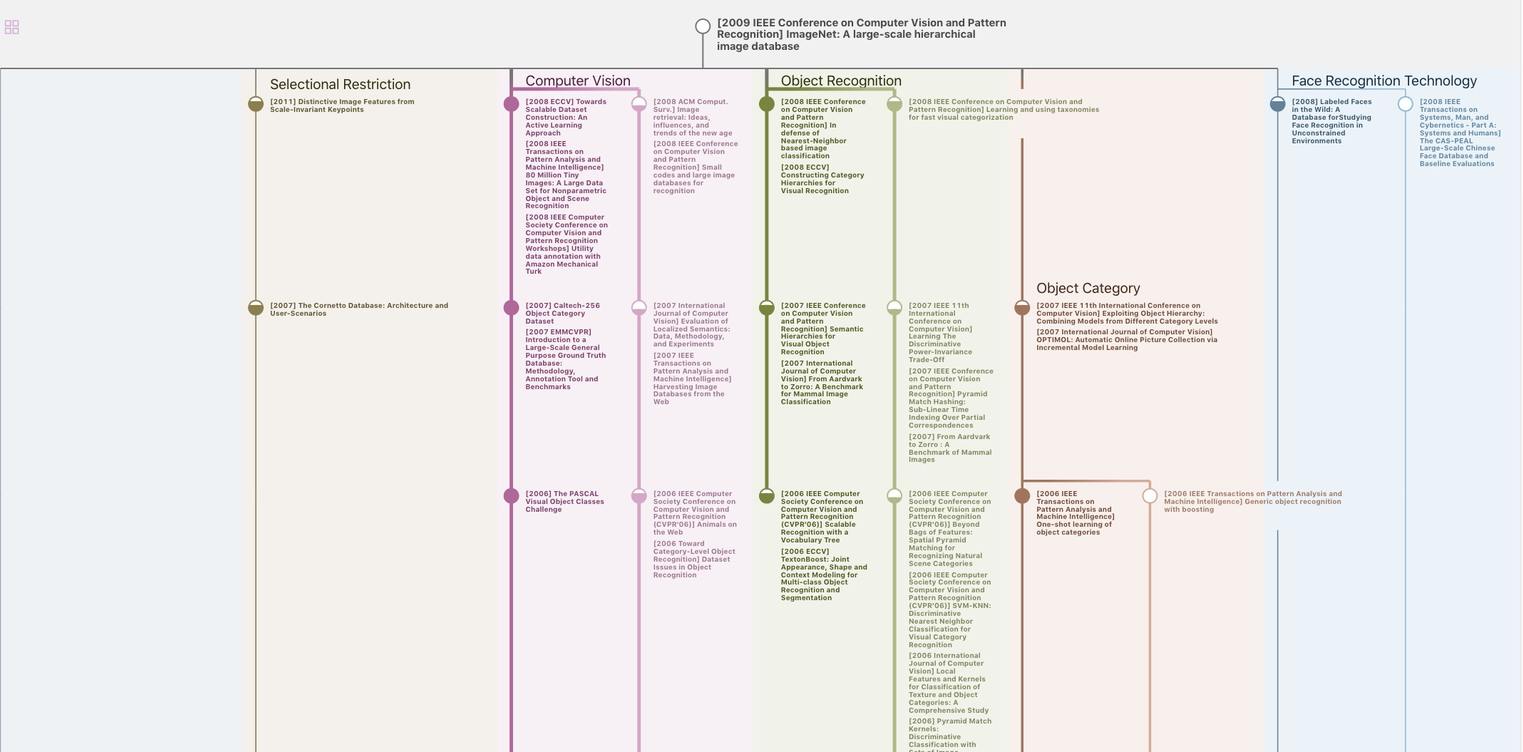
Generate MRT to find the research sequence of this paper
Chat Paper
Summary is being generated by the instructions you defined