Data-Driven Modeling of Urban Traffic Travel Times for Short- and Long-Term Forecasting
IEEE Transactions on Intelligent Transportation Systems(2023)
摘要
Travel time duration and traffic forecasting in urban environments is of critical importance for efficient supply chain logistics and accurate navigation services. The paper compares data-driven statistical and machine learning approaches for urban traffic travel times forecasting on a 15-minute resolution for the next 24 hours. A detailed analysis of the available historical data is performed, including historical floating car data for a one-year period. Data analysis is followed by development and evaluation of baseline, ARIMAX, neural network and gradient boosting models. The predictions are generated on a day-ahead forecasting horizon where the machine learning models are propagated in a recursive strategy. Recursive approach implies more accurate short-term forecasts that are crucial in the context of nowcasting, while maintaining good accuracy on long-term horizons necessary for daily operation planning. The evaluation of the models accuracy is conducted on four main arterial urban routes in the city of Zagreb, Croatia, with travel times data obtained from a commercial navigation service. A detailed analysis of the results is performed, with a special focus put on the accuracy of near future predictions, as well as different models and model structures.
更多查看译文
关键词
urban traffic travel times,forecasting,data-driven,long-term
AI 理解论文
溯源树
样例
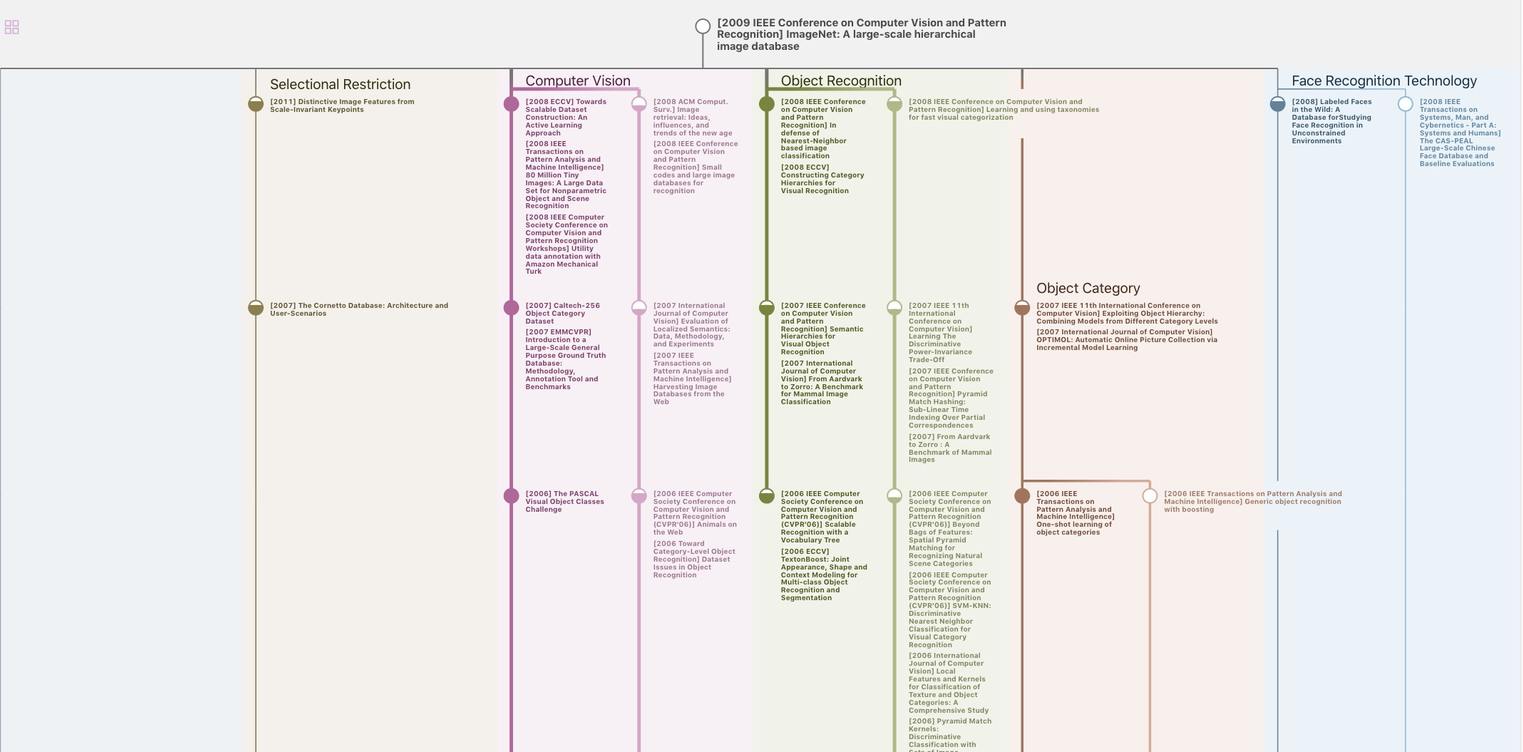
生成溯源树,研究论文发展脉络
Chat Paper
正在生成论文摘要