Localized and Incremental Probabilistic Inference for Large-Scale Networked Dynamical Systems
IEEE Transactions on Robotics(2023)
摘要
In this article, we present new algorithms for distributed factor graph optimization (DFGO) problems that arise in the probabilistic inference of large-scale networked robotic systems for both batch and real-time problems. First, for the batch DFGO problem, we derive a type of the alternating direction method of multipliers (ADMM) algorithm called the local consensus ADMM (LC-ADMM). The LC-ADMM is fully localized; therefore, the computational effort, communication bandwidth, and memory for each agent scale like $o(1)$ with respect to the network size. We establish two new theoretical results for the LC-ADMM: 1) exponential convergence when the objective is strongly convex and has a Lipschitz continuous subdifferential and 2) $o(1/k)$ convergence when the objective is convex and has a unique solution. We also show that the LC-ADMM allows the use of nonquadratic loss functions, such as $\ell _{1}$-norm and Huber loss. Second, we also develop the incremental DFGO (iDFGO) algorithm for real-time problems by combining the ideas from the LC-ADMM and the Bayes tree. To derive a time-scalable algorithm, we exploit the temporal sparsity of the real-time factor graph and the convergence of the augmented factors of the LC-ADMM. The iDFGO algorithm incrementally recomputes estimates when new factors are added to the graph and is scalable with respect to both network size and time. We validate the LC-ADMM and iDFGO in simulations with examples from multiagent simultaneous localization and mapping and power grids.
更多查看译文
关键词
Distributed estimation,distributed optimization,multiagent systems
AI 理解论文
溯源树
样例
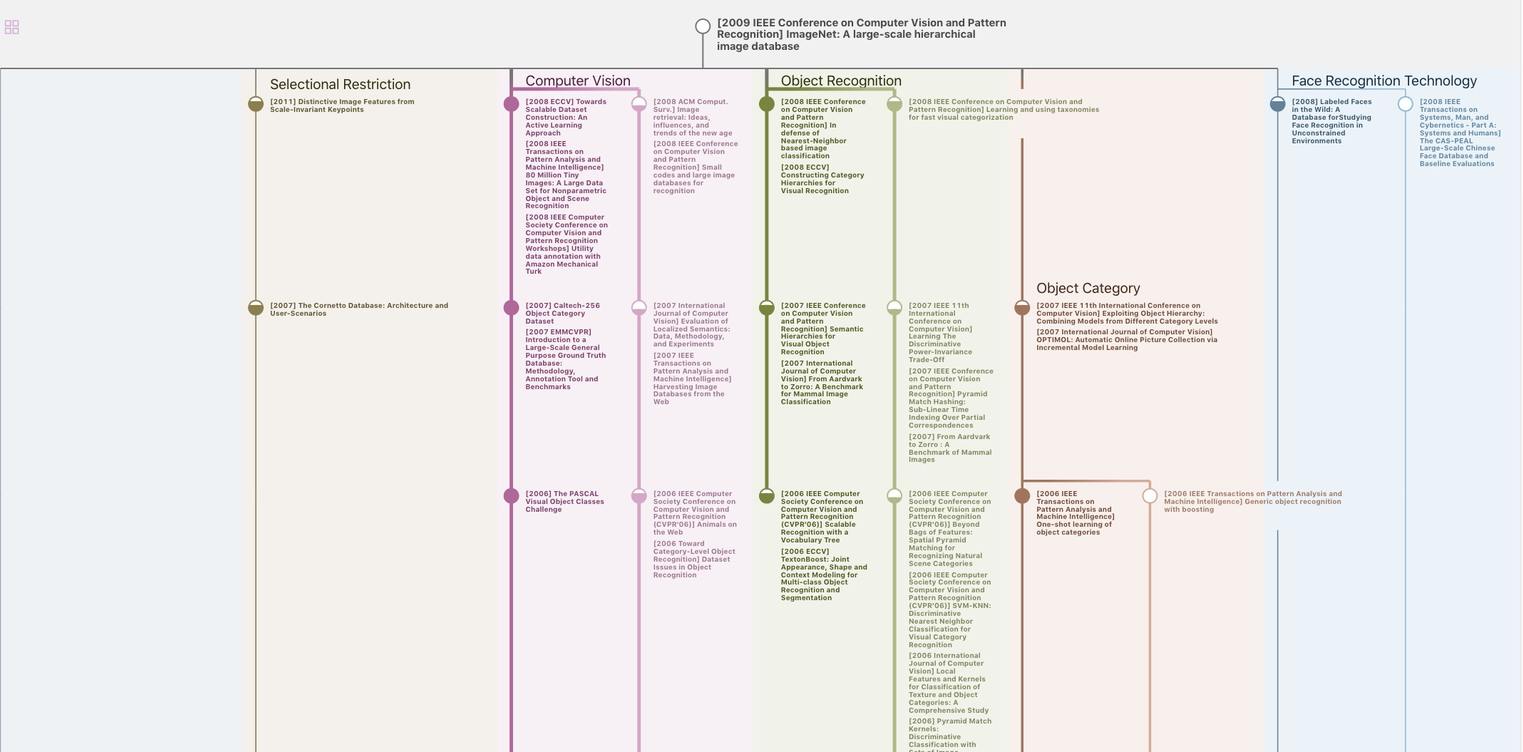
生成溯源树,研究论文发展脉络
Chat Paper
正在生成论文摘要