Crack detection and evaluation of photovoltaic modules based on data-driven
2023 IEEE PELS Students and Young Professionals Symposium (SYPS)(2023)
摘要
Photovoltaic (PV) modules are prone to crack faults in harsh outdoor environments. Therefore, the diagnosis and evaluation of PV module cracks are essential for improving the reliability, efficiency, and safety of PV power stations. When PV cracks occur, the characteristics of PV modules change significantly, making it challenging to diagnose cracks using physical models. In addition, a large number of modules in PV power stations require the real-time and rapid detection of cracks. Because of the abovementioned problems, for a large-scale PV dataset, this paper proposes machine learning-based LightGBM to achieve the fault diagnosis of PV module cracks in PV strings. LightGBM improves the gradient-boosting framework in the process of training a massive dataset. Due to the advantages of its framework, LightGBM can achieve fast and accurate crack fault diagnosis compared with other machine learning classifiers. On this basis, the paper constructs an evaluation model based on the weight of evidence (WOE) value to evaluate the operation state of photovoltaic string. Experiments show the accuracy and effectiveness of the proposed method.
更多查看译文
关键词
Photovoltaic module cracks,LightGBM,crack diagnosis,state evaluation
AI 理解论文
溯源树
样例
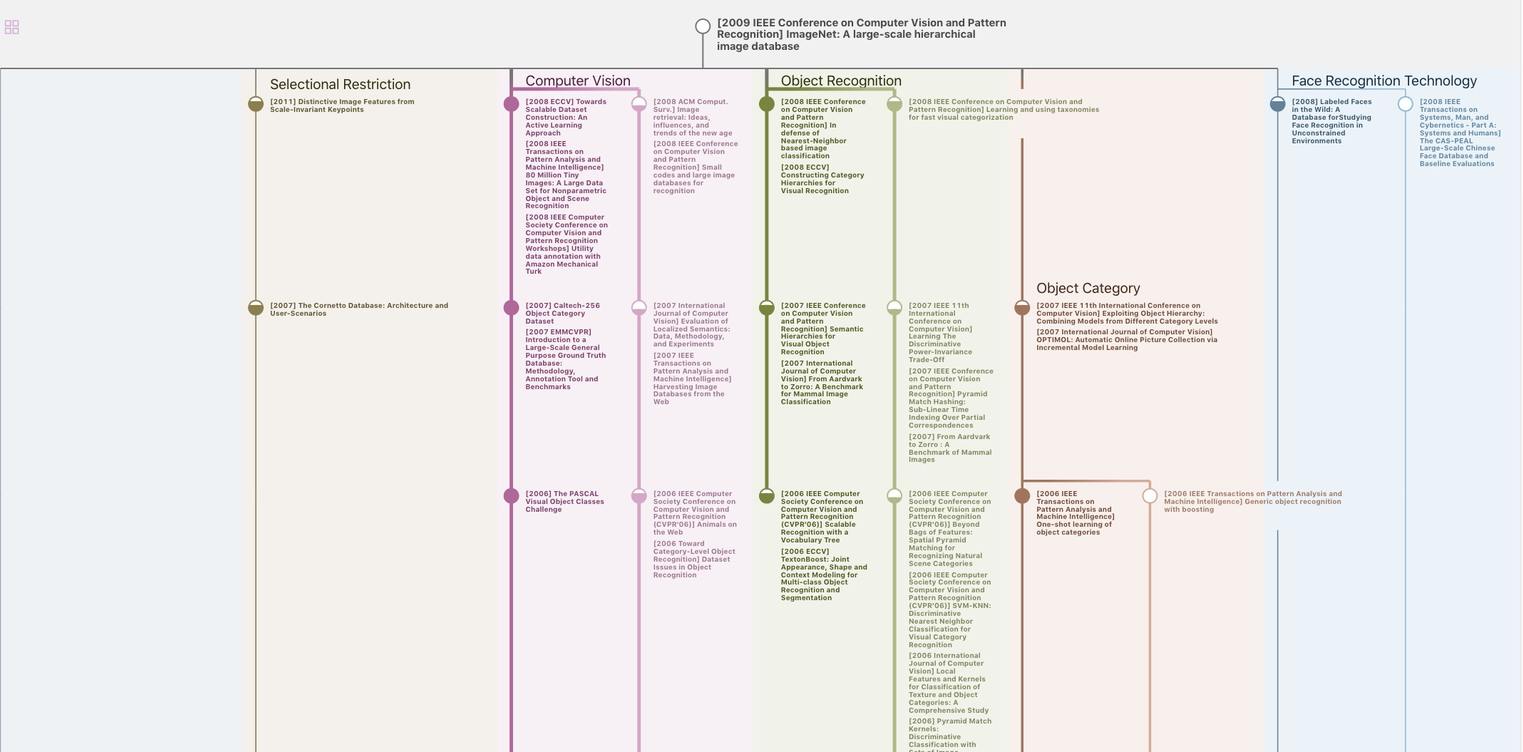
生成溯源树,研究论文发展脉络
Chat Paper
正在生成论文摘要