COOLer: Class-Incremental Learning for Appearance-Based Multiple Object Tracking
Lecture Notes in Computer Science Pattern Recognition(2023)
摘要
Continual learning allows a model to learn multiple tasks sequentially while retaining the old knowledge without the training data of the preceding tasks. This paper extends the scope of continual learning research to class-incremental learning for \ac{mot}, which is desirable to accommodate the continuously evolving needs of autonomous systems. Previous solutions for continual learning of object detectors do not address the data association stage of appearance-based trackers, leading to catastrophic forgetting of previous classes' re-identification features. We introduce COOLer, a COntrastive- and cOntinual-Learning-based tracker, which incrementally learns to track new categories while preserving past knowledge by training on a combination of currently available ground truth labels and pseudo-labels generated by the past tracker. To further exacerbate the disentanglement of instance representations, we introduce a novel contrastive class-incremental instance representation learning technique. Finally, we propose a practical evaluation protocol for continual learning for MOT and conduct experiments on the \bdd and \shift datasets. Experimental results demonstrate that COOLer continually learns while effectively addressing catastrophic forgetting of both tracking and detection. The code is available at \url{https://github.com/BoSmallEar/COOLer}.
更多查看译文
AI 理解论文
溯源树
样例
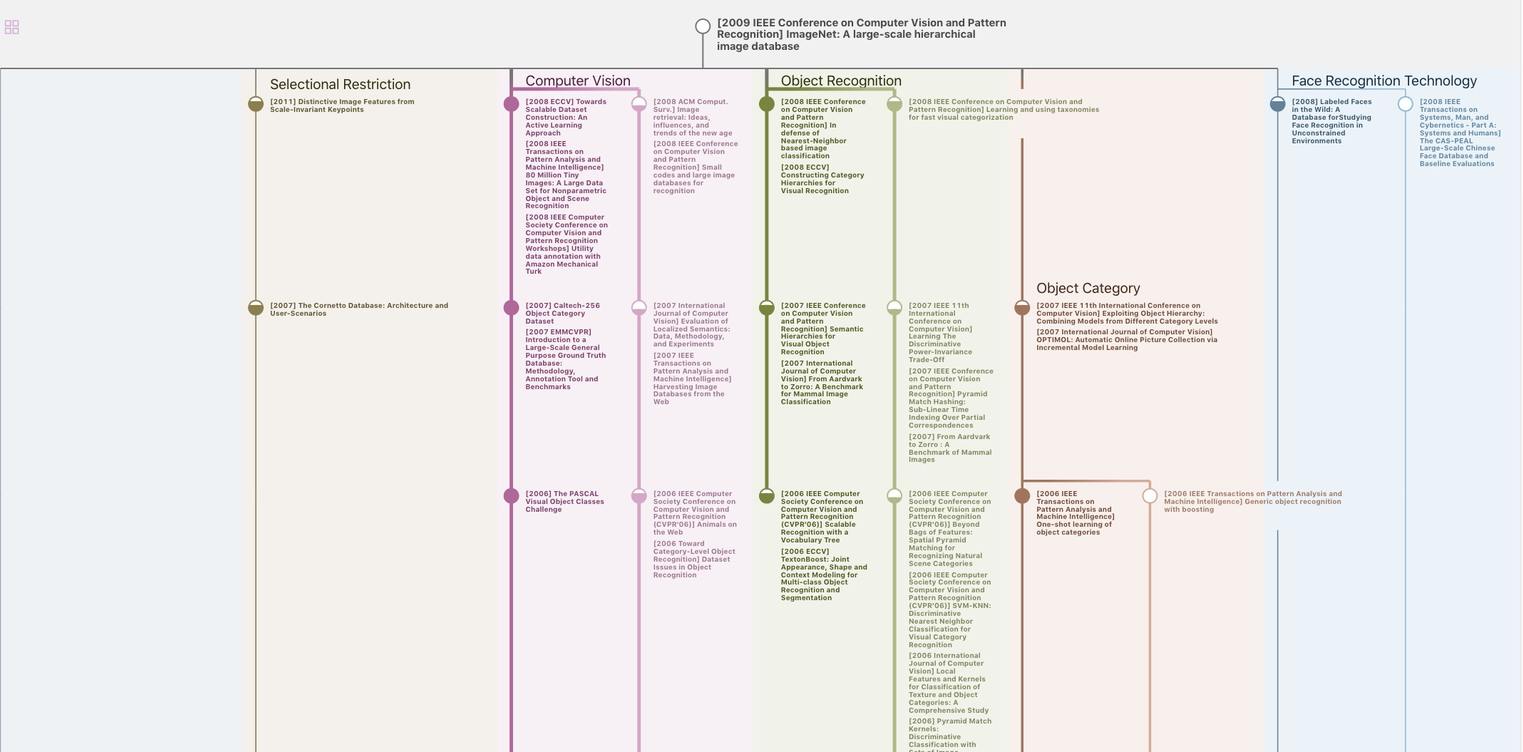
生成溯源树,研究论文发展脉络
Chat Paper
正在生成论文摘要