Assessing the Risk of Permafrost Degradation with Physics-Informed Machine Learning
arXiv (Cornell University)(2023)
摘要
Global warming accelerates permafrost degradation, impacting the reliability of critical infrastructure used by more than five million people daily. Furthermore, permafrost thaw produces substantial methane emissions, further accelerating global warming and climate change and putting more than eight billion people at additional risk. To mitigate the upcoming risk, policymakers and stakeholders must be given an accurate prediction of the thaw development. Unfortunately, comprehensive physics-based permafrost models require location-specific fine-tuning that is challenging in practice. Models of intermediate complexity require few input parameters but have relatively low accuracy. The performance of pure data-driven models is low as well as the observational data is sparse and limited. In this work, we designed a physics-informed machine-learning approach for permafrost thaw prediction. The method uses a heat equation to regularize data-driven approach trained over permafrost monitoring data and climate projections. The latter leads to higher precision and better numerical stability allowing for reliable decision-making or construction and maintenance in the areas endangered by permafrost thaw with a time horizon of decades.
更多查看译文
关键词
permafrost degradation,machine learning,physics-informed
AI 理解论文
溯源树
样例
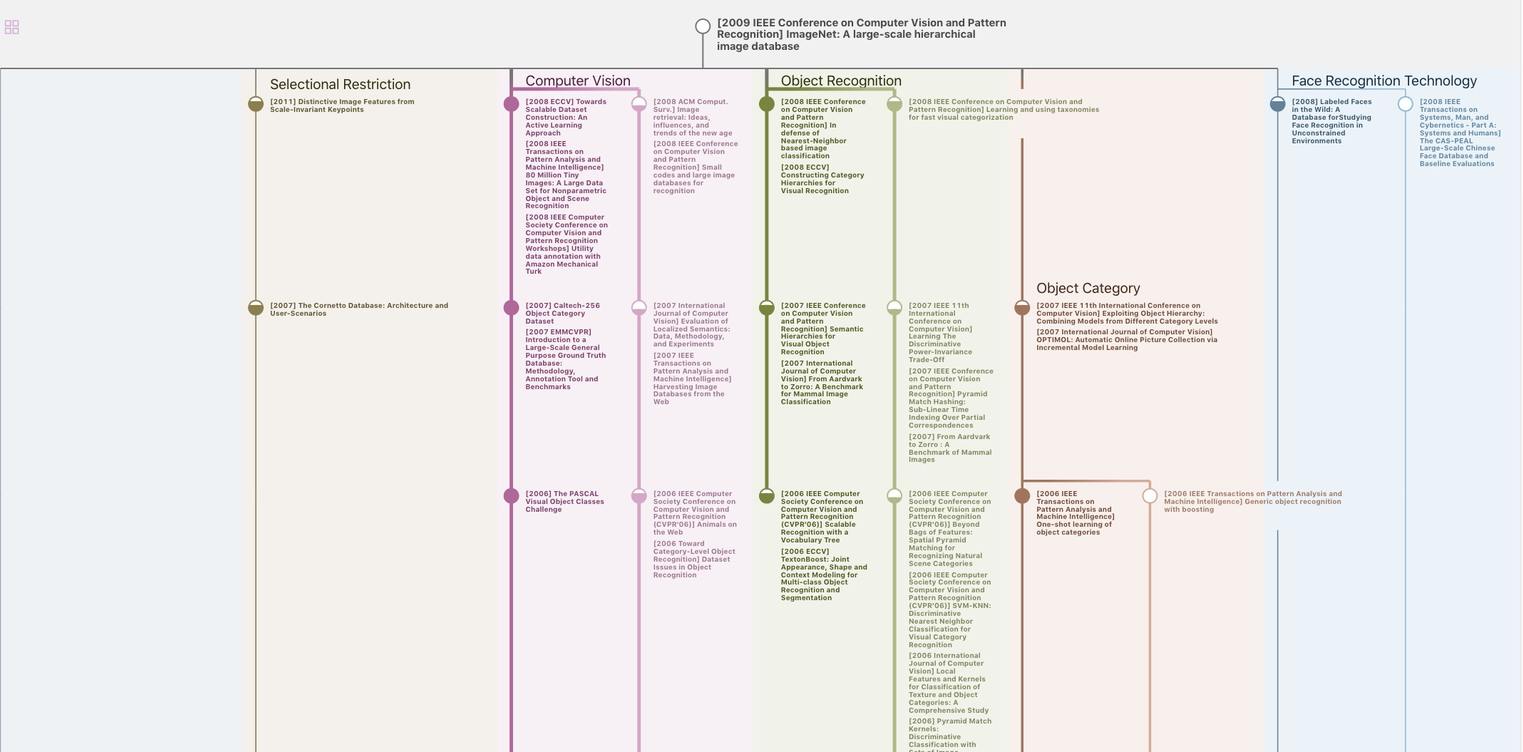
生成溯源树,研究论文发展脉络
Chat Paper
正在生成论文摘要